Artificial Intelligence – A Counterintelligence Perspective: Part IV
In my first post in this series, I wrote that one definition of artificial intelligence (AI) is a machine that thinks. But is it? Several people with technical backgrounds in the AI field reached out to me after reading that post. One comment I received that I found striking is that AI is neither A nor I. Instead, it is just computer code. Nothing is thinking; a computer is just following directions. And AI is just inputs to outputs for a goal.
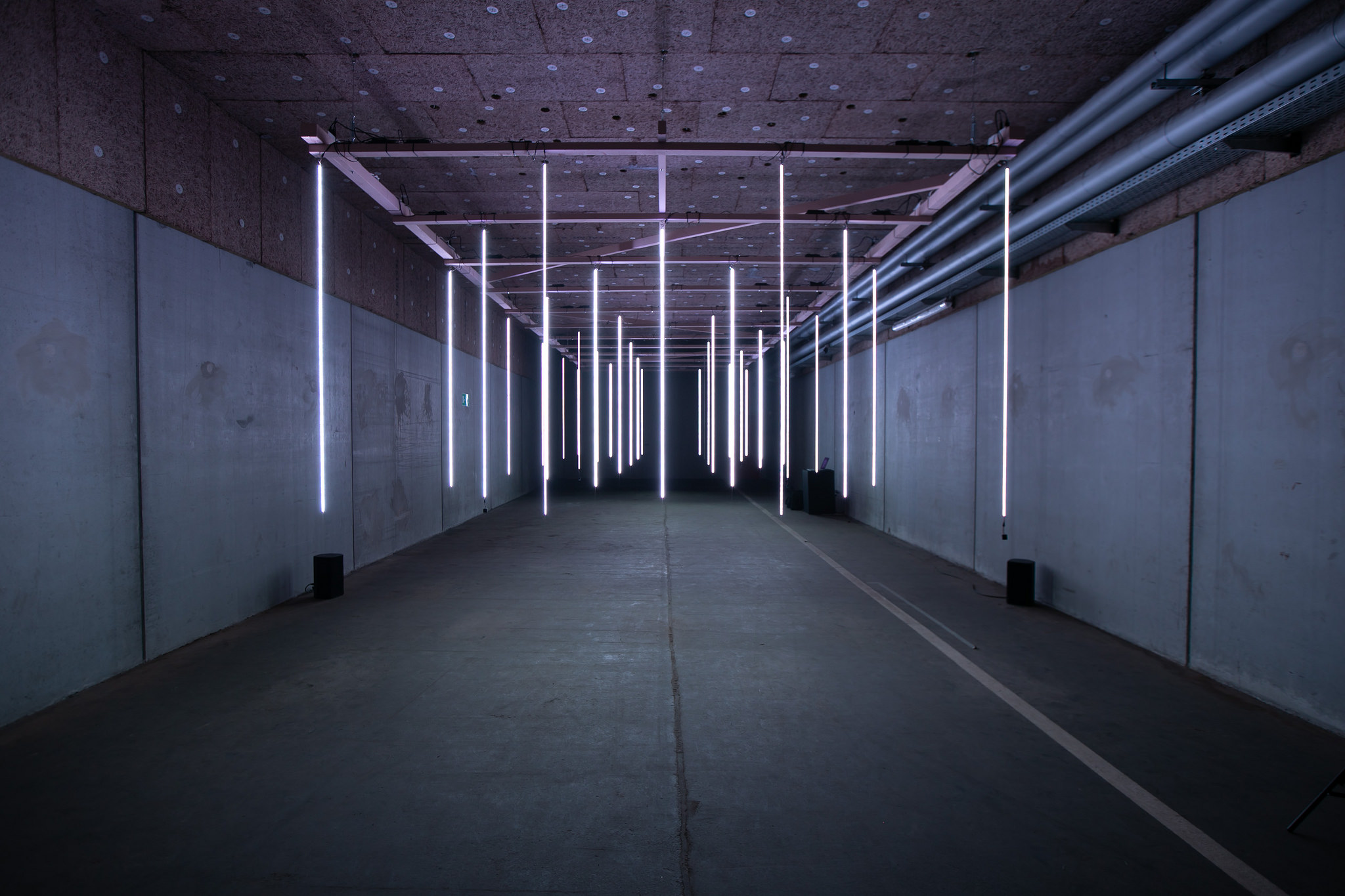
Published by The Lawfare Institute
in Cooperation With
In my first post in this series, I wrote that one definition of artificial intelligence (AI) is a machine that thinks. But is it? Several people with technical backgrounds in the AI field reached out to me after reading that post. One comment I received that I found striking is that AI is neither A nor I. Instead, it is just computer code. Nothing is thinking; a computer is just following directions. And AI is just inputs to outputs for a goal. In other words, the AI field is nowhere close to developing a machine that actually functions in any way that humans would regard as “thinking.”
Several critiques argued that despite all the hype, the algorithms that underlie much of what is called AI are old and have not progressed much in many years. What has changed is that raw computer processing power has increased substantially and the amount of data available for analysis and machine “learning,” especially through deep neural networks, has exploded. More computing power plus more data have made “AI” (or whatever you want to call it) much more powerful. But to the extent that developers have improved AI systems in recent years, they have done so through trial and error and numerous messy, unpublicized failures. The complexity of some of those programs makes them difficult to understand, explain or evaluate, and the truth about how they work may remain hidden. AI systems—algorithms, computers and data—produce significant results in limited and specialized contexts, but they do not do so in an elegant manner. Moreover, this is still a point at which to remain highly skeptical of any system (whether called AI or something else) that purports to produce a significant result if the developers cannot explain in understandable terms how it works. The initial critique continued that breathless claims about the transformative nature of AI today and in the near future is too often marketing hype that is difficult for corporate leaders, investors and government officials to understand or challenge.
It is hard for me to evaluate this critique fully. I am a lawyer and a policy guy who, at various times, had significant operational responsibilities in national security organizations. I am not a computer scientist or mathematician. I have never written a single line of code. Throughout most of my career, I have had to address the legal, operational and policy implications of some extremely complex technologies. I have usually dealt with that by getting over my fear of looking stupid in front of others and asking dumb questions to ensure that I actually understood something sufficiently to do the analysis and make the decisions that I had to make. In reality, this is the dilemma that all non-expert leaders face in dealing with the broader societal implications of technology; they must understand the technology as best they can while remaining mindful that they could be wrong about it in material ways. Even though there are limitations on their ability to understand AI, private- and public-sector leaders will confront AI-related decisions in the immediate future. They will need to do their best to understand AI—a piece of good news is that some are doing just that.
Two aspects that they will need to understand, however imperfectly, are whether a so-called AI system is explainable and whether it is biased. In part one of this series, I briefly discussed the concepts of “explainable AI” (XAI) and “ethical AI.” In a nutshell, as the name implies explainable AI is an AI system that a person (or the system itself) can explain to another person—that is, someone can explain how an AI system works and why it produces particular results. Here is one concise description of XAI.
An ethical AI system is one that is not biased in some unacceptable way, such as on the basis of race, gender or ethnicity. The two concepts are a bit intertwined, but fundamentally they are both about humans’ understanding what an AI system is doing and why and then viewing what it is doing as acceptable. Many people have written about both of these topics, and I will not try to cover the entire rich field that has developed around them. Below, I focus instead on a few of the counterintelligence implications of the two related concepts that also link to some broader issues.
Here is what I said about XAI and ethical AI in my first post in this series:
It is important to discuss the difference between what is called “explainable AI” or “ethical AI” and what is sometimes referred to as “black box AI.” The basic idea is simple: An explainable AI is one in which a human can understand and explain what the AI did and why it did it. An ethical AI is a system that is not biased in some unacceptable way. For example, with explainable and ethical AI we can answer basic questions such as, why did the autonomous vehicle decide to turn left instead of right? Why does the AI think that the face in the surveillance camera video is the same as the one in the mug shot? Why did it translate this sentence from Arabic into English in this fashion? Why does the AI think that this person is more likely than another person to commit a crime? Why does the AI think that person is not a good credit or insurance risk? Is the system biased for or against certain races or genders? AI systems that we can’t understand are black-box AI systems. Black-box systems are the opposite of explainable systems and of ethical systems, though a system can be explainable and still be biased.
AI systems can have biases that need to be understood. Those biases may come from the programmers who write the initial code to create the AI algorithms, or from the data sets that the programmers exposed the AI to for it to learn, or from some other source that people may not understand initially. The worry here is that gender, racial, ethnic or some other bias may have unintentionally influenced the initial programming or selection of the data set for learning. Such biases can have profound effects on the outcomes that the AI produces, something people need to be mindful and cautious about. Making sure that AI is explainable and ethical requires dedication and effort. As I understand it, the mathematics associated with some AI design today are so complicated that even the most advanced human mathematicians struggle to keep up. Developing and maintaining explainable and ethical AI systems will require effort and vigilance. In later posts, I’ll have more to say about some counterintelligence concerns I have about black-box AI that expands on comments I made at a recent Brookings event.
For many reasons, democratic societies will favor explainable and ethical AI. Google recently posted its AI principles, apparently in response to a vigorous internal debate on the topic. Whether or not one agrees with those principles, intuitively it makes sense that all of us should be concerned about ensuring the ethical use of AI. Free societies should have no interest in developing AI that is improperly biased and will cause injustice or will make important decisions that humans cannot understand or unpack.
Lots of folks are addressing the numerous domestic legal and policy implications of AI. This includes issues regarding the use of AI to drive decisions and actions in the criminal justice system, the insurance field and the transportation sector. If decisions regarding, for example, incarceration and insurance rates and coverage are based in part on AI, some people will not like the results and will challenge those decisions. Proponents of using AI in those settings eventually will have to explain to courts and regulators how it works and establish that it is not improperly biased. Similarly, when AI is used to support autonomous vehicles such as cars and delivery drones there will inevitably be accidents and litigation will ensue. Someone will have to explain how the AI system worked so that the judicial system can figure out who is liable to what degree for causing the accident. Although such questions are complex and will take some time for courts and regulatory agencies to sort out, I have every confidence that they will be able to do so. I will leave more detailed discussion of those issues and questions to others for the moment.
Instead, in keeping with the counterintelligence focus of this series, I want to discuss two important baskets of implications and risks related to XAI and ethical AI: (1) effective management of certain operational, privacy and reputational risks; and (2) adversaries’ use of black-box AI.
Managing operational, privacy and reputational risk. In the first post on this subject, I outlined some basic principles of counterintelligence. One way to synthesize those points is to say that conducting counterintelligence operations is really hard because the field itself is complex, the threats are numerous and the available resources are limited. As a result, counterintelligence officials need to be smart about focusing their efforts on the right problems. They need to make effective and efficient use of limited counterintelligence resources. AI tools might be able to help tremendously in that regard if deployed appropriately.
But this is where the AI critique that I outlined above comes in. If you are conducting counterintelligence operations (or, really, any governmental or private-sector operation) and someone in your organization or a contractor proposes spending time and money on an AI solution for one of the problems, how do you know it is worth it? How do you know that the solution is actually A and I? Spending organizational assets wisely is a basic management problem—but the part of the AI critique above that resonates with me is that often folks try to promote the latest technology tool that they have discovered or that they are selling with almost religious zeal. So-called AI can be made to sound so fantastic that there is a risk that a lot of resources might be wasted on junk AI—that is, AI that simply does not deliver as promised.
Junk AI would include a data analytics system that is said to learn from and provide insights into certain types of data but that in reality only works with a highly curated and scrubbed data set and not with messier data that customers are more likely to encounter in real life. For example, organizations logically want to develop or purchase tools to help them analyze open-source social media data to look for trends, patterns and threats. While these tools hold significant promise for solving some vexing problems of data management and analysis, buyers need to beware. They need to ask probing questions about how the systems work; what data was used to develop the system; why and how that data-driven machine learning will work in the actual theater of operation that the organization deals with; and how the developer insured that the algorithms, data selection, learning, and analytical results were not biased in some unacceptable way. In other words, decision-makers will not be doing their jobs if they do not insist that AI systems that someone proposes to develop or purchase for them are actually explainable and if they do not confirm that such systems are ethical. Putting aside for the moment questions about morality, values or ethics, at a minimum this is a question of effective management: It is bad to waste an organization’s resources on something that does not work.
Procuring, developing and deploying flawed AI systems would waste time, effort and money, and it would probably harm the reputations of organizations that mistakenly use junk AI. Consider the example of a law enforcement or intelligence agency that incorrectly includes or excludes people from consideration as threat actors because of the racial or gender bias of the AI that they use. This could potentially result in some level of misallocation of critical assets, such as deploying scarce counterintelligence or counterterrorism assets chasing the wrong people while the real bad guys go undetected. Obviously, this is dangerous and unacceptable. In addition, this might result in outright injustice as innocent people are subjected to the full panoply of intrusive government investigative activities. This could happen in a narrow way—for example, the AI might direct an agency at one particular person and his associates. More broadly, a flawed AI might be used to analyze data relating to thousands or millions of innocent people. In those situations, too many government personnel may be exposed to data they shouldn’t possess or review—because the system doesn’t really work—and data often ends up squirreled away in a variety of information technology systems that, again, needlessly expose people to that data and put it at increased risk of a breach from internal or external malicious actors. This is a privacy nightmare that might result in constitutional and/or statutory privacy violations and attendant civil liability for the organization. If any or all of this becomes known to the public, then the reputation of the organization will suffer and the public will be less inclined to trust, support or help such an organization.
Moreover, if a law enforcement or intelligence agency uses AI that is not explainable (and ineffective) or is biased and is not diligent in ferreting out that reality early, it runs the risk that it might be forced to defend that AI in court someday, either because a prosecutor tries to use some AI-generated fact in evidence or because the organization is sued as a result of, say, searching the home of an innocent person and injuring or arresting someone in the process. Law enforcement agencies are sued for searching the wrong place (it happens), and civil discovery will reveal organizational flaws. In addition, for the federal government and many states there are freedom-of-information laws that could result in poor AI purchasing and implementation practices coming to light. And there are leakers, whistleblowers and qui tam relators. There are also inspectors general. And there is Congress. Simply put, one way or another, junk AI will be outed and there will be a significant price to pay for using it.
Junk AI will be tantalizing in the short term and discrediting in the long term.
Risks With Adversaries’ Use of Black-Box AI. Democratic societies concerned with protecting civil liberties, justice, preventing discrimination, and complying with, for example, international human rights law and international humanitarian law are more likely to insist that public- and private-sector entities use explainable and unbiased AI. But whether or not the U.S. and its allies use black-box AI, I expect that their adversaries will. And I am quite concerned that democratic societies will not be able to hold the line on black-box AI across the board because black-box AI systems might produce advantageous results for the adversaries in certain circumstances notwithstanding the legal, political, moral and human costs. Especially in the areas of defense, intelligence and cybersecurity, it may not be possible for democracies to refuse to use black-box AI.
Consider a hypothetical system that provides discernible—even real—security advantages but that does so in a fashion that has high risks of collateral costs to innocent people, whose operation nobody can explain, and that is reasonably suspected of discriminating against some group. It is reasonable to expect the U.S. and its allies to look hard at such a system and probably decline to use it.
But some adversaries will not care about explaining how an AI system works or the ethical implications of using it in light of the collateral damage—such as injustice or physical harm—that it causes so long as they can understand and like the results it produces.
If they do that, they may be able to make advances in AI more quickly than the U.S. and its allies through a process that is more aggressive, messy and dangerous. Obviously, this will be bad. In a crisis, the pressure on public- and private-sector entities in democratic societies that are called upon to respond to adversaries’ aggressive and effective use of AI—which may be driven by black-box AI—will be tremendous. In a pinch, they may be offered and turn to a black-box solution.
Americans and our allies should not put our heads in the sand about this likelihood; instead, we should expect and plan for it. We should debate now how and when the use of black-box AI is acceptable and what after-the-fact oversight of such actions should occur. This is going to happen in some way that is impossible to predict accurately right now but that can still be planned for. To paraphrase Gen. Dwight Eisenhower, plans are useless but planning is essential.