Lawfare Daily: Daniel Kokotajlo and Eli Lifland on Their AI 2027 Report
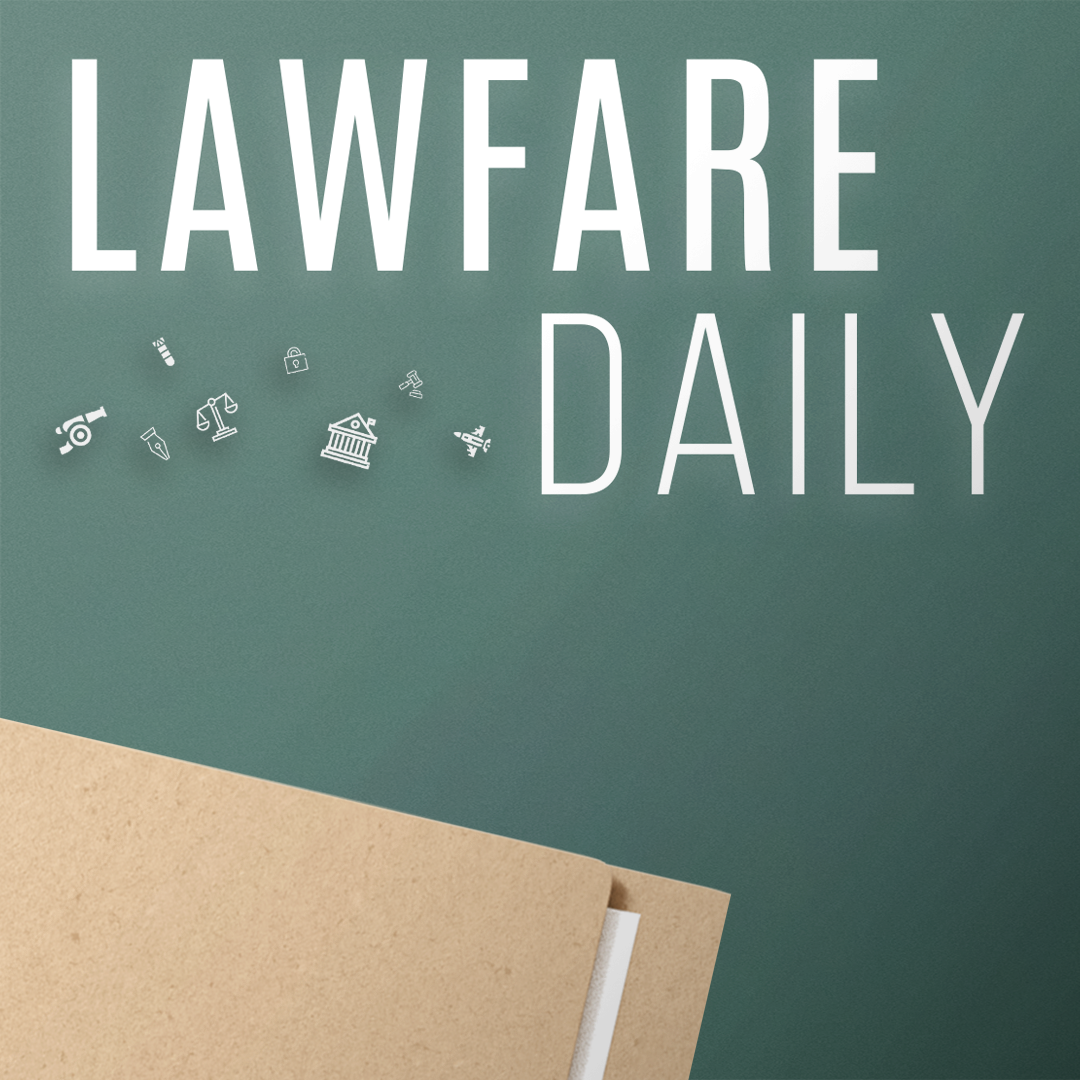
Published by The Lawfare Institute
in Cooperation With
Daniel Kokotajlo, former OpenAI researcher and Executive Director of the AI Futures Project, and Eli Lifland, a researcher with the AI Futures Project, join Kevin Frazier, AI Innovation and Law Fellow at Texas Law and Contributing Editor at Lawfare, to discuss what AI may look like in 2027. The trio explore a report co-authored by Daniel that dives into the hypothetical evolution of AI over the coming years. This novel report has already elicited a lot of attention with some reviewers celebrating its creativity and others questioning its methodology. Daniel and Eli tackle that feedback and help explain the report’s startling conclusion—that superhuman AI will develop within the next decade.
To receive ad-free podcasts, become a Lawfare Material Supporter at www.patreon.com/lawfare. You can also support Lawfare by making a one-time donation at https://givebutter.com/lawfare-institute.
Click the button below to view a transcript of this podcast. Please note that the transcript was auto-generated and may contain errors.
Transcript
[Intro]
Eli Lifland: What we hypothesize is that if you have this super, what we call a superhuman coder—which is like a, you know, an AI system that is as good at the, as the best human coder, except much faster and cheaper as well—that this would kind of like in various ways, improve the research productivity by a significant amount.
Kevin Frazier: It is the Lawfare Podcast. I'm Kevin Frazier, the AI Innovation and Law Fellow at Texas Law and a contributing editor at Lawfare, joined by Daniel Kokotajlo, former OpenAI researcher and executive director of the AI Futures Project, and Eli Lifland, an AI Futures Project researcher.
Daniel Kokotajlo: If we do get something like superintelligence, it's probably gonna look crazy. There's a lot to think about and a lot's gonna happen really fast. And not enough people are talking about this and not enough people are thinking about it, and a very small set of people are like thinking about it, specifically using the medium of actual concrete stories.
Kevin Frazier: Today we're talking about a report that Daniel and Eli co-authored, AI 2027. It's a hypothetical narrative exploring how AI may evolve in the coming years. Its bold predictions warrant a close read, and of course, a thorough podcast.
[Main podcast]
What if you could peer just two years into the future and catch a glimpse of a world shaped by superhuman AI? What if that future was bleaker than many hope? What if we change policies, alter AI development, make AI a key issue for the general public? What if that future was more utopian? What would you do to have those answers?
Well, Daniel, Eli, and a few other co-authors set out to describe in vivid detail what AI development may bring come 2027 and beyond. In their newly released report, AI 2027, they offer a bold, year by year narrative of how AI might evolve and upend our social, political, and economic systems by the end of the decade.
Relying on extensive research, tabletop exercises, and their own forecasting acumen, Daniel and Eli paint quite the picture: powerful new AI systems shaping geopolitics, accelerating scientific discovery, and forcing world leaders to grapple with questions of alignment, oversight, and control.
Some have praised the report's creativity and clarity. Others have raised an eyebrow or two at its speculative leaps, but few can deny that it struck a nerve. That's why I'm so glad they joined the Lawfare Podcast today.
Daniel, I just finished watching White Lotus and I was exposed to way too many spoilers, so some folks may be upset, but I'm gonna ask you to just go straight to the chase. Daniel, what future can we expect? What is the best case scenario for AI? What is the worst case scenario that you all paint in your AI 2027 report?
Daniel Kokotajlo: So AI 2027 is a scenario forecast. It is just one of many possible futures. It just is the one that seems most likely to us, but it's still, you know—the real future will probably be quite different in many ways from AI 2027. We, we did our best shot at trying to guess things, but obviously we're gonna be wrong in a bunch of ways. We still think it's valuable to try.
We, you know, if we had infinite time, we would've portrayed a whole spread of different possibilities representing a whole bunch of different ways things could go. Because we had limited resources, we just chose to pick two possibilities branching off from one branch point.
So in our story, we get superhuman coder autonomous agents in early 2027. And then that speeds up the overall AI research process enough to get complete automation of the AI research cycle, so what you might call artificial general intelligence for AI research at least, maybe not for other stuff, but at least for AI research, it's able to do everything by mid 2027.
And then we have a sort of branch point where there's some concerning warning signs that, you know, maybe, maybe the AIs aren't actually aligned. Maybe the goals that they're supposed to have, they haven't quite stuck. And in one of the branches, they sort of like apply more scrutiny, slow down a little bit, fix the underlying problems, and then proceed.
In the other branch, they do some sort of surface level patch that makes the problem seem to go away and doesn't require a sort of serious overhaul of, of what they're doing. And that allows them to go faster, but means that they end up with misaligned systems that are smarter than them running their data centers and all of that.
And then in both of our endings, the year of 2028 is very exciting. In both endings, there's this army of super intelligent AIs on the data centers over the course of 2028 that's working with the president and the company to be aggressively deployed into the military and the economy to make tons of money to build all sorts of new weapons, build all sorts of new factories to beat China, right? And meanwhile, of course, over in China, a similar thing is happening with, with their own AI systems.
In both endings, we depict there ultimately being a deal between the U.S. and China rather than a war. We could, it could very easily go to war, but we, we depict a deal happening instead in both endings. And then later after this sort of amazing robotic transformation has gone on for a while, the outcome is either really, really, really good for most humans or really, really, really bad depending on who controls the AI basically.
And in, in the ending where the AIs were misaligned, nobody controlled them. They had goals that were different from what their human owners wanted, and this only becomes truly apparent when it's too late and they've been put in charge of everything.
And then in the other ending where they managed to solve the technical alignment issues and figure out exactly how to put the goals that they want into the AIs, then the oversight committee of the project ends up in control because they're the ones who get to choose—they're the ones who control the AIs basically. And the oversight committee is a merger of, it involves people from both the corporate world, CEOs, and also the government, the president in particular.
Kevin Frazier: So we have this race scenario, we have this slow down scenario. And what I want listeners to, to realize is you can read this report, you can listen to this report, you can check out great graphics throughout the entire report. This is quite the multimedia experience. Honestly, I did listen to part of it on 1.25 because we scheduled this so quickly and during runs I was running from super coding AI, running from the Chinese, running from misaligned AI. It was a great experience. So highly recommend folks dive into this.
Daniel Kokotajlo: The best way to read it is on the website, I think.
Kevin Frazier: Yeah, reading on the website, I do recommend it because you get to check out these really cool graphics develop as you're reading it. Admittedly, I did just have to shove it into some of my workouts, so I, I do apologize. I promise I will get the full experience.
But Eli, I gotta know, you two and your co-authors are some of the, the smartest AI researchers—tons of experience, lots of technical knowledge. Why do this? Why come up with this story as, as Daniel used the term, this story of how AI might develop? What were your goals in developing this story and sharing it so broadly?
Eli Lifland: Yeah. In terms of our goals, I, I guess sort of like, you know we believe that—we're not sure—but we believe this sort of transformation to a world where, you know, superhuman and eventually, you know, very superhuman AI could come within three years, five years, if not maybe ten years, twenty years, etc.
And, you know, we think that this is something that's very hard to predict. So as Daniel said, you know, we're not gonna get everything right. But we think it's very important to think through very carefully. You know, it's very easy to kind of like have a very vague high-level story of what might happen, but you know, when you drill into the details, you realize some things are wrong.
It also seems helpful just to communicate as a tool of communication. You know, kind of like doing the scenario allowed us to even figure out ourselves what we thought would happen, 'cause you know, we didn't know going into the, into the story we hadn't thought in this little detail.
And we also hope that it serves at a good communication tool, right? So others can look at our scenario and be like. I disagree with that, you know, I disagree with this part because of, of, of this reason. And then, you know, we can, we can listen to their arguments and potentially change our mind if we agree and they can also lay out their alternative scenarios. And then, you know, over time we can kind of compare, compare the scenarios and see, see how things are going and see, you know, which direction it's heading. So I think, you know, a big, a big part of just the motivation for this is because, you know, we first of all just helped us ourselves figure out what's going on.
And we believe that kind of society is like not paying enough, nearly enough attention to this possibility and kind of like writing it out in this detailed way can help us like move towards a situation where we can really see a bunch of different concrete ways that things could play out from a, a wide variety of viewpoints and kind of like move forward from there.
Daniel Kokotajlo: It's important context. I think a lot of people might be coming to AI 2027, relatively fresh, not having thought much about super intelligence, but that's not the case for the authors of this. We have been thinking about these things for years, and it's not just us. Lots of people have been thinking about these things for years, especially lots of people at these companies.
So OpenAI, Anthro and Google DeepMind were literally founded on the idea that they were going to build super intelligent AGI that's better than humans at everything, and that this would massively transform the world, and that this could lead to amazing utopia for everybody, or that it could lead to human extinction. These ideas were there at the founding of these companies and they're part of the motivation for why so many people join these companies. And within these companies this conversation has been ongoing for years.
And then similarly outside the companies, there's a small but growing, you know, literature on the topic. There's people trying to forecast timelines until AGI. There's people trying to forecast takeoff speeds, which is sort of like, what will the, what will the rate of growth look like in various metrics, once we have powerful AI systems that are able to automate all or most of, of the work.
There's a growing literature on this topic, but one thing that seemed to be missing from the literature, to me, was a, like, concrete story of how it's all supposed to come together, right? There's, there's lots of stuff you can go read about, like here's my estimate for how long it will be. Here's my probability distribution for, for the arrival time of artificial general intelligence. And here's my definitions of what artificial general intelligence is. And here's like some essay about why I think we should wake up the U.S. government and get the government involved. And here's someone else's essay about why they think that's bad.
Like, there's all, there's all this discourse. But one thing that seemed to be notably lacking was this sort of like, so how is this all supposed to go? Like what, what's the actual picture? How does it all come together? So we set out to write that.
I think of it as a compliment to all that discourse rather than a substitute. I'm not saying you should do scenarios and not do that other stuff. You should totally be doing the other stuff. You should be, you should be extrapolating trends, making bets, forecasts about things—you know, all that stuff is great, but then it helps to have these sort of concrete scenarios to sort of focus the discussion and also sort of like stress test your ideas.
Because I think a very common experience that many people have had, including myself, is realizing that the sort of vague sense of how they thought things were going to go doesn't actually, it's not even internally consistent. And if you try to write it out in detail, you realize that your, your own vague sense was actually just incoherent.
So, so Eli and I have run these scenario exercises occasionally over the last year or two where we, you know, get a bunch of people in a room and say you're gonna spend the next couple hours writing up like a scenario that represents how you think the next couple decades are gonna go in the history of AI.
And people have found them helpful. For example, multiple different people have tried this and then realize that their timelines have to shorten because they like, you know, wrote out the advancements that they expect in the near term. And then like wrote do dot, dot, dot, and then 15 years later we get super intelligence or something, and then they're like, wait a minute. Like given all the things that have happened up to here, it just shouldn't take that long, you know.
So, so people find them useful. We found them useful and we're hoping that it will inspire lots of other people to start asking the right questions and thinking more seriously about these topics and hopefully writing alternative scenarios.
Kevin Frazier: Yeah, I, I gotta say one of my favorite parts that you all include in the report is this openness hey, if you disagree, give us your scenario, right? This sort of invitation for fanfiction—for lack of better phrase, I'll come up with a better term when, when I'm feeling more creative—but this fanfiction of, okay, hey, great, if you think we're gonna branch off in a different direction. Tell us when. Tell us why, and let's keep this conversation going.
And to your credit, there are so many folks, and this isn't necessarily a product of their own doing, but we've reduced a lot of the conversation about the future of AI to what's your P-doom, which doesn't do anything for anyone of just saying this is my probability that AI will result in some extinction level event for humanity. But this sort of exercise really forces everyone to say, okay, what are the tangible steps that may occur in the near future that could alter the course of human history, or at least the course of AI development.
And Eli, I guess one thing that stood out to me was this focus as Daniel pointed out on an AGI for super coding, basically a, a, a super coder, and why that's so important for the future of AI development. So, you know, I'm just a humble law professor, so if you could describe to me what, why is it so important for an AGI researcher, a a super coder to develop with respect to the overall takeoff path that we can see for AI's future.
Eli Lifland: Yeah, I guess let's kind of start by talking a bit about the sort of like AI R&D process.
So we're gonna simplify it a bit here, but, you know, kind of at a high level, there's like a few phases. You know, the first phase is like, you kind of choose what experiment you wanna run. You know, you have like hypothesis that you want to test, you have a direction, you wanna see how well it works, and then you, you know, code up this experiment.
And so this is like—a big part of the coding is just kind of like, okay, you have this experiment idea. Now I'm gonna actually code this up. And then you run the experiment, right? And you like see what the results are and then, you know, you have this kind of cycle of like, you know, making different adjustments to the code, then rerunning and then, and you, and you're like monitoring the experiments, etc.
And so, you know, at a high level we can think of their as being kind of two types of skills that are involved here. One is like experiment prioritization or we sometimes call it research testing, which is like deciding which experiments to run and like how to interpret the results of experiments. And then the other kind of skill is like implementing the experiments. And so this is like the coding basically, you know, that you need to do to test out, you know, maybe you have some hypothesis like, you know, what if we, what if we try to, you know, alter the structure of the neural network a bit in this way? And then, and then you, and then you kind of need to test it out.
And so what we hypothesize is that if you have this super, what we call a superhuman coder—which is like a, you know, an AI system that is as good at the, as the best human coder, except much faster and cheaper as well—that this would kind of like, in various ways improve the research productivity by a significant amount.
You know, one example is that there might be just experiments that, they're very valuable to run, but they currently take a long time to code, right? And so because of that, right now, like they don't get run or like if they do get run, you have to invest a really a lot of time into, you know, actually coding up the experiment to sort of like test how it, how it, how it goes. But once you have the superhuman coder, you're able to sort of like unlock these new types of, these new experiments for much cheaper that are very, you know, valuable.
And yeah, and maybe a bit more context here, is that kind of like the, the way to think about this is that, you know, there's two kind of inputs here. There's like the labor from the, from the researchers, like for example, who are doing the coding. And then there's like the compute—basically, you know, the, the sort of like amount of computing power that is like, needed to run these experiments.
And so like basically what we do in our kind of forecast is we think about different ways that having these superhuman coders would allow you to more efficiently use the compute in experiments to get better, to sort of like run more valuable experiments and get better results.
And yeah, the one I gave was just like, sort of like one example, but just, you know, generally, for example, like there are various other things that the AI is like, maybe they're like better at writing code which doesn't have bugs. You know, like maybe there's oftentimes right now, like, you know, you run an experiment and then you realize you weren't actually testing what you thought you were testing. And you know, if you had these superhuman coders who were like pouring over the code, like, you know, before, its sort of like the experiment even started and also monitoring it, you know, constantly, like this is something that could, you know, allow you to sort of like reduce the amount of the bugs that you have.
And so we list various ways that having the coder could, could kind of like, speed up the AI R&D process. And then we see like how long it'll take to go from coding to, you know, the next step of like a fully superhuman AI researcher. And then we kind of go on, go on from there. And, you know, correspondingly with each milestone we estimate how much it would speed up the AI R&D process. And then we estimate, you know, what that would sort of imply for how long it took to get from that milestone to the next one.
And one, one more thing to note here about the coding is that the AI companies are already focusing a lot on coding, you know, to varying degrees, but there's already a lot of focus there. So that's something that is not fully even a prediction, but just an observation, you know, that, that AI companies are really putting a lot of effort into making their AIs better at coding already.
Kevin Frazier: Yeah. This idea of just a constantly improving AI system, it's, it's pretty obvious even for someone like me with less technical experience to see why, if you were an AI lab, you would wanna bet as much as you can on that strategy because you're going to jump ahead your, of your competitors if you get there first.
And I think just to ground this report a little bit further, I'll go over a quick except from, a couple quick high points. So we focus here on OpenBrain, a fictional AI company set in the United States that builds a powerful AI system known as Agent One. China then quote, wakes up and doubles down in a big way on its own AI efforts, stockpiling and concentrating compute which we all know is a core ingredient of AI development. On the domestic front, we see AI lead to some layoffs; some folks begin to get upset about AI's integration into the economy.
Then, great plot twist: China steals one of the advanced AI models from OpenBrain. What does the U.S. president do? Naturally responds with a cyber attack. The U.S. DOD and OpenBrain then reach an extensive contract with one another. We see continual tech breakthroughs and developments, a few setbacks from time to time, but then ultimately we see that self-improving AI is a attained as soon as June 2027, which leads to a huge upswing in AI progress.
So Daniel with this just initial set of circumstances that we could see develop in the next two years, can you walk through your degree of uncertainty with respect to some of these developments? Obviously, like you said, if you had all, all the time in the world, you would've outlined a whole bunch of alternative scenarios.
You're no, no stranger to coming up with some really astute, really accurate AI predictions. What gives you a sense that these scenarios in particular may be on the table or are worthy of study by folks who are thinking about AI governance right now?
Daniel Kokotajlo: The way that we like to think about it is by breaking down into these milestones of capability. So we sort of chopped it up into superhuman coder, superhuman AI researcher, which is sort of AGI, but for AI research, at least it can do the whole thing. And then beyond that, we have a couple other levels beyond that, and eventually you get to super intelligence.
So we, we chopped it up into these levels and each of these levels is a sort of separate intellectual question of like, how long do we have until some company builds a system that qualifies as a superhuman coder. And then separately how long do we have from that point until some company has a system that qualifies as a superhuman AI researcher. And then separately from that point, you know, etc. So to answer your question, I sort of have to sort of talk about all those things separately, but I won't bore you with all of that. You should go read the actual thing. But I'll try to give a lightning summary.
For the superhuman milestone, there, it’s like, well, that's the first, that's the, the closest milestone and it's the thing we have the most evidence for to try to guess at. We're still not confident in it, but almost around the same time that we published AI 2027, some benchmarks came out that we like quite a lot, such as those produced by METR. And then also OpenAI has a paper replication benchmark basically.
Companies and nonprofits and so forth are trying to make, are starting to make these benchmarks that are not like traditional benchmarks, which are basically multiple choice questions, but are instead like you plug the AI into some set of GPUs and you give it internet access and do tell it you have eight hours to, you know, make progress on this engineering problem. And then it just like interacts with the GPUs and runs experiments on them and basically like does the whole loop by itself.
So they, they're, they're starting to get, you know, benchmarks that are with those sorts of relatively challenging tasks, and those benchmarks I think are like starting to get roughly in the vicinity of what we would actually want it to be measuring for the superhuman coder milestone.
It's still not quite there yet; they're still, you know, the, the, the first system that crushes these benchmarks will still not be a superhuman coder, probably because they're only, you know, eight hour long tasks, right, and they're like relatively well scoped, right. But it's a, it's, it's moving pretty fast in terms of performance on these benchmarks. Every, every couple months, they are getting new state of the art performance on these benchmarks.
And if you assess the trends, it looks like in a, in a year or two, they'll be crushing these benchmarks and so they'll be routinely able to do reliably, you know, eight hour long coding tasks, right fully autonomously.
So it's that sort of evidence that we point to—and you can read about it on the website—that's the sort of evidence that we point to for why we think the superhuman coder milestone could arrive as early as 2027. Our actual credence distribution, of course, is still, you know, pretty spread out.
So we think maybe it's gonna go a lot faster than we think could happen by the end of the year. But probably not, you know, maybe it'll take longer than we think and it'll be, you know, 2029, 2030, 2031 before we get the superhuman coder. But I think my 50% mark is like early 2028, and I think Eli has like a different 50% mark, but not that different for something.
Yeah. So that's all like like expression of uncertainty about the first milestone, and then for the second milestone, it's like, well, we also have uncertainty about that. And you can read about this on the website. We have our takeoff speeds research page that talks about how we quantified our uncertainty there. So, so you can go, go read about that way, but obvious—there's just a lot of uncertainty. But, you know, that's, that's where it is.
Kevin Frazier: Well, and Eli, to, to give us a sense of this actual process, because I think folks will be fascinated to learn—who is actually writing this report? Who were you all consulting? There is a ton about international relations going on here. There's obviously big technical questions, there's legal questions, there's political questions. Who was involved beyond the two of you and, and how did you all try to build additional expertise into this process to try to make the narrative as compelling and accurate as possible?
Eli Lifland: So in terms of who is involved besides Daniel and me, so there's one other person, Thomas Larson, who is kind of a full-time contributor to the report, he has experience both the technical AI safety and some experience with AI policy. And then Scott Alexander mostly helped rewrite the report. But you know, he also, has thought a lot about AI over the last many years. You know, he has a lot of experience with that as well.
And then one other person, Romeo Dean, was a contributor and you know, in Romeo's case he wrote the, the kind of compute and security supplements. So for example, he was thinking about the questions of like, would China be able to steal these, these weights and why? So we started, actually for background, we started writing the scenario actually 15 months ago in January 2024. And I think Romeo, you know, he focused a lot on the compute and security experts, and I think over the course of the report, he talked to a lot, a lot of the best, you know, experts in both of these fields and kind of became an expert himself, I, I would say in many ways.
And then in kind of the other aspects, you know, we, we, we kind of did our best, especially as I'd say on the technical level. You know, we, we, we sort of, we sent out the draft to hundreds of people for feedback. We had multiple iterations of drafts. We probably received—
Daniel Kokotajlo: We got feedback from more than a hundred people.
Eli Lifland: Yeah. I think over a hundred people commented on, around a hundred people commented on the last draft. And definitely like over a hundred people commented on at least one of our drafts.
A lot of them were, were kind of coming from a technical background. Yeah. But we also, you know, had some, some people with policy. We, we tried to get some feedback. You know, we kind of, we, we had this sort of like—we weren't sure kind of how we wanted to write, for example, the slow down ending, what the geopolitical relations should be like in that, so we sent out, you know, this document to, to some people who have you know, some, some expertise in that to sort of inform that.
And then the other thing I'd say is, yeah, so we, we did, we also did a few kind of sessions with various experts where we would just kind of like, you know, have kind of a whiteboarding session about a particular aspect of the scenario.
The other thing is these war games. So, you know, we, these, kind of like tabletop exercises, we've, we've run about 30 of them now and, you know, they're with varying groups of people, you know, have with varying levels of expertise and types of expertise. But we did cover, you know, a decent amount.
So, you know, we had some people who have, you know, been in the government, who were playing the U.S. government, for example; some experts, you know, some technical experts were like often playing, you know, the AIs, we, you know, some, some, some—we have a player that plays the AIs and we have a player that plays, you know, the leading company.
And anyways, so, you know, we kind of tried to sort of like do as best as we could in terms of using, using those and using the expertise that came out of that to inform the scenario as well.
Kevin Frazier: Well, I'm, I'm thinking just as a side note, we should probably release a AI 2027 board game of sorts. You just war game everywhere. I wanna walk into an arcade bar and find this tabletop exercise, but we'll, we'll scheme that out later.
Daniel, you've already shared this in a number of different forums, including getting coverage in the New York Times. Part of that writeup was from Ali Farhadi, the chief executive of the Allen Institute for AI, and he had this to say about the report. Quote, I'm all for projections and forecasts, but this forecast doesn't seem to be grounded in scientific evidence or the reality of how things are evolving in AI.
Not exactly a ringing endorsement. What's your response to Ali? You just walked through hundreds of comments, lots of round tables. Why do you think he reached this conclusion that it wasn't grounded in scientific evidence? And what perhaps would you say he was missing in reaching that conclusion?
Daniel Kokotajlo: Yeah, I mean, I'm glad he said he is all for scenario projections. I would say can you point to anything else that's remotely as good? I think the answer is no. This is a very sort of undersupplied thing that we're doing, and that's why we're, that's why we're doing it.
We want to see other people write their own counter scenarios and, you know, say why they think it's going to go like this instead of like this, and here's the reasons for it. That's like part of the hope that we have for this. You know, you can see our thing on the website. You can see our reasoning and our research behind it on the website. If you have objections to it, you can talk about them. You can message us.
We actually have a bounty; we're, we're gonna be giving out small prizes, monetary prizes, couple thousand dollars for the objections and bugs reports that we find most compelling. And we're also going to be giving out prizes to the alternative scenarios that people write that seem, seem good to us. So, you know, we'll see.
Kevin Frazier: Yeah. Well, hopefully he takes you up on that offer and writes his own alternative history.
Daniel Kokotajlo: Yeah. Again, I think it's important to mention that like these companies are trying to build super intelligence. It says so on their website, like the CEOs talk about it, like who knows what the future is going to look like, but if we do get something like super intelligence, it's probably gonna look crazy.
There's a lot to think about and a lot's gonna happen really fast. And not enough people are talking about this and not enough people are thinking about it. And a very small set of people are like thinking about it, specifically using the medium of actual concrete stories, and we're hoping to change that.
Kevin Frazier: What I appreciate about your analysis as well is one of the key factors you're tracing throughout this story is what is the public's concern? What is the public's level of concern about AI and by extension, what is the level of public awareness about this issue?
And there was just a Pew poll that came out a few days ago showing a huge disparity in expert evaluations of AI and whether, whether it's going to be for good or for ill, and then the general public's own evaluation of AI, and they're wildly different. And so I think having these more accessible stories and approaches that are—admittedly there are parts of the, the report where I had to, you know, rewind on my podcast, go back, do that mile again, and, and listen back to it—but far more accessible than a lot of technical reports.
So, Eli, can you share a little bit more about the reception you've had so far? Are you getting calls from your old high school buddies saying, just read AI 2027, can't wait to write my own fanfiction. What's the reception been like so far?
Eli Lifland: I think it's been overall great. Better than my expectations. Yeah. In terms of like, old friends reaching out, I've, I've had a few—
Daniel Kokotajlo: When Eli says better than his expectations, that really means something. He made forecast quantitatively beforehand about like various metrics. So—
Kevin Frazier: Forecast on forecast, it's just forecast to the nth degree. This is great.
Eli Lifland: Yeah. Yeah. That is true. I, it did, it did, it did surpass my, my forecast. I think in terms of like the Twitter views, it was like. Maybe 70, 75th percentile or something like that, which is pretty good.
And I think overall, yeah, I've had, you know, a few, a few friends reach out, generally, generally pretty positive. I mean, unsurprisingly from friends of course, but I think in terms of the reception of what I've seen, you know, I think the Twitter, for example, the discourse on Twitter has been overall, overall pretty good.
We've been, we've been hearing as well, you know, good things from—it seems like, you know, it's, it's generating a lot of discussion and in, in lots of different places. Like in, you know, for example, in the, within the AI companies, within some you know, government or government adjacent you know, think tanks, etc.
And yeah, so overall I'm quite, I'm quite happy, you know, obviously not every comment has been positive, but that's to be expected and I think. I've been quite heartened—I'm, I, one other thing I'll say is that I've been hardened to see, you know, people who, even if they strongly disagree with this scenario, some people have been saying, you know, they found it very valuable. You know, for example, Dean Ball, who, you know, disagrees with us about sort of like, at least the takeoff speeds, I think, and maybe also the timelines and, and, and some other things, said he still finds it very, very valuable to read through.
Kevin Frazier: Yeah. Well, and Daniel, I, I'm coming to think of you of sorts as a Nate Silver of AI, you know, putting your reputation on the line, making some bold predictions. Is this gonna become a habit from you? Are we gonna see every two years a new AI 2029 and AI 2031? Is this something we should expect to be a, a recurring product?
Daniel Kokotajlo: Plausibly. So we, we are gonna have a team retreat in a few weeks and decide like what we're gonna do next, and we have a lot of exciting options. For example, we might turn the tabletop exercise into more of a thing as you were hoping for, and making it like our main product and making it really good instead of this side show. We, we did the tabletop exercise basically for ourselves to give us ideas for how to write the story, but it's turned out to be way more popular than we expected. And anyhow, so one idea is to lean into that.
Another idea is to keep doing more of these things on a regular cadence like once a year or something like that, because the evidence is gonna keep rolling in. The arguments are gonna keep becoming better and more advanced and more nuanced. And so we are gonna be updating our beliefs and our sense of how the future is gonna go is probably going to change substantially every year. And so we should keep writing about it and, you know, so yeah, there's tons of ideas for what we could be doing, but that's one of them.
Kevin Frazier: That's excellent. Well, before I let you all go I'll, I'll turn to each of you for a sort of rapid, rapid question here. What's one thing you really want folks to take away from this report?
Is it a sort of we have agency over how AI's gonna go, we can see this slow down? Or we can see this race where as Daniel mentioned, we could have really, really bad outcomes or really, really good outcomes. Is it general awareness? What's, what's one thing you hope people take away from this?
Eli Lifland: Yeah, for sure. I mean, so obviously it depends on—different people have kind of like different things they can do, you know, in terms of action. I think the main thing that I am excited about is people kind of like having more of a sense that, wow, this could something like this crazy could actually happen, you know, maybe on this timescale, maybe on a longer timescale, who knows, maybe even on a shorter timescale as Daniel mentioned. But just understanding that exactly how important this topic is and really getting that on a gut level is I think something that I think is important.
And I hope that basically it spurs, you know, some direct action, but also, you know, some reaction of, wow, you know, there aren't, there really isn't enough going into this for its importance. You know, like there should be more things like this scenario; you know, government should be investing much more in like, understanding what's going on, more like emergency preparedness.
Daniel has, you know, written up some proposals with, with Dean Ball about transparency, so like, you know, the public and the government has a better understanding of what's going on inside these AGI companies. So I think that's something that I'm hoping will at least be one reaction is people thinking, wow, this is really important. We need to, you know, invest more in various ways and kind of understanding the likelihood of this and, and what to do about it.
Kevin Frazier: Excellent. Daniel, how about yourself?
Daniel Kokotajlo: I think it's important to mention that there isn't any one particular thing that we were hoping. Like by its nature, this is a sort of like a comprehensive, holistic scenario about how we think things might go and what people take away from it is going to be different for different people.
Some people might come away from it being like, oh, wow, like, yeah, I can totally see how superhuman AGI could happen. Actually, previously I thought that like that was just complete sci-fi, but now I can see a sort of like step-by-step pathway to it, wow.
Other people might be like, oh yeah, I already thought that, obviously. Like I work at, you know, OpenAI, but, but I hadn't really taken into account the like power grab risk before. Like I hadn't really realized like, even if like alignment is not really an issue and we can easily control the AIs, there's this important political question of who gets to control the AIs. And like currently it's going to be like a CEO or something, you know, like, so like that's a, that's like another example of something that someone might take away from it.
Other people might have already been concerned about that, you know, raw, AI constitution of power, etc., but then they read the section on the alignment stuff and they're like, oh yeah, these things are neural nets. I guess I feel dumb for not noting that before, but wow. Like, yeah, neural nets means we can't actually like necessarily see what they're thinking, you know, like that means maybe they're not actually controlled, you know.
Like, like, so it's gonna be different for different people. And, and, you know, and that's part of what's exciting about this is that we're, you know, we're getting feedback from all these people, people rolling in, being like, oh, like this footnote was really interesting.
Or like, oh, I disagree with this part here. And like, it's, it's really a sort of this broad push and it's, and it's gonna be different for different people. There's not any one particular thing that we're targeting.
Kevin Frazier: Well folks, we're gonna have to leave it there. Be sure to check out your local library for a new section called Ai-fi. I'm just kidding; that's my, my own coinage for this new category of AI development.
But really exciting, AI 2027 report—-Daniel Eli, thank you so much for joining. We'll have to leave it there. Until next time.
Daniel Kokotajlo: Thanks, Kevin.
Kevin Frazier: The Lawfare Podcast is produced in cooperation with the Brookings Institution. You can get ad-free versions of this and other Lawfare podcasts by becoming a Lawfare material supporter at our website, lawfaremedia.org/support. You'll also get access to special events and other content available only to our supporters.
Please rate and review us wherever you get your podcasts. Look for our other podcasts, including Rational Security, Allies, The Aftermath, and Escalation, our latest Lawfare Presents podcast series about the war in Ukraine. Check out our written work at lawfaremedia.org.
The podcast is edited by Jen Patja. Our theme song is from Alibi Music. As always, thank you for listening.