Our Robophobia
We are biased against robots, and it is killing us.
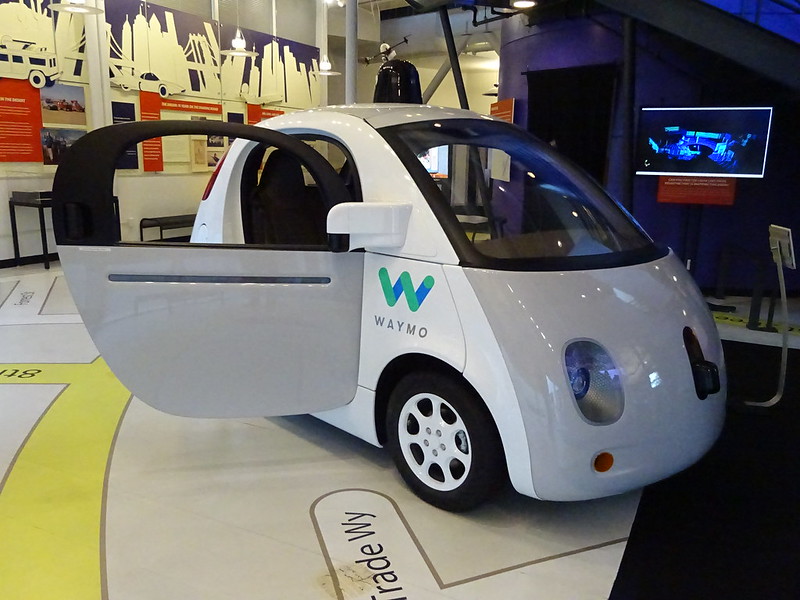
Published by The Lawfare Institute
in Cooperation With
If someone told you they were not vaccinating their children against measles because the vaccine carries some risk, you might be forgiven for raising an eyebrow. After all, everything is risky. The question is whether there is more risk of harm in vaccinating your child than in not vaccinating them. (The answer, of course, is that there is not.) Worse still, the costs of that risk are not internalized: When you choose not to vaccinate your child, you are choosing to put my child at risk of infection. The anti-vaxxer movement is irrational, and it runs contrary to nearly all medical advice and U.S. policy. It is rightly ridiculed as dangerous, medieval thinking that harms the innocent.
Yet this is how people often talk about robots. In a new law review article, I detail what I call “robophobia,” biased thinking about the costs and benefits of nonhuman decision-makers. I survey the surprisingly large empirical research drawn from around the world documenting human bias against robots, and I make the normative case against robophobia. I argue against thinking like the anti-vaxxers, which means: (a) comparing robots to the alternative, (b) debunking the idea that this is merely a matter of personal choice and (c) considering debiasing strategies to mitigate our anti-robot biases.
When a self-driving car crashes, when an algorithm makes the wrong bail determination, when an artificial intelligence-powered hiring tool discriminates: people panic. Newspapers run headlines decrying this new form of dangerous discrimination and policymakers call for new oversight. Commentators stress the risk of harm. Rarely do people ask, “How does the risk of using the robot compare to the risk of not using it?”
Yet that is the essential question.
Scholars and commentators are absolutely right to ask whether algorithms will be biased in one way or another. But why focus on the downside? If a central question for the current time is determining when and where to delegate decision-making authority to machines, then we should consider comparing human bias to machine bias. Anxiety about algorithmic bias is at a fever pitch; the sentiment, as Chief Justice John Roberts recently told a group of high school students, is “beware the robots.” And while legal scholars are consumed with the ways in which machines are biased against humans, there is next to no legal scholarship on the ways in which humans are biased against machines.
It turns out that people are deeply biased against machines in all sorts of interesting ways, and we write this bias into our laws and policies. An immense body of behavioral research (almost none of which is included in legal scholarship) suggests that people are simply unwilling to accept machine errors. For example: We are wary of self-driving cars and will not tolerate accidents, despite the fact that we tolerate more than a million deaths a year at the hands of human drivers; people prefer medical advice from a human, even when they are told a robot is more accurate; lawyers have more faith in human-generated evidence, even though automated discovery tools are more accurate; and in the military, a serious campaign is underway to stop autonomous weapons, even though they promise to significantly reduce mistakes and rule abuses.
Algorithms are already better than people at a huge array of tasks. Yet we reject them for not being perfect.
Of course, people do not reject all robots. Indeed, in 2020 it might seem odd to say that this society, which is so deeply high-tech, is biased against machines. Many of us live in a perpetual state of connectedness where we let algorithms make all sorts of important decisions for us, from the news stories we see to the dating profiles we review. In fact, personalized technology is so deeply embedded in our lives that many of us feel that we would be better off with less technology in many areas of daily life. So let me be clear that I am not arguing for more or less technology overall. Rather, I am arguing for a more thoughtful approach to when and how people deploy nonhuman decision-makers. We defer to robots uncritically in some areas, and then eschew them in other areas, following our intuitions instead of making a carefully considered choice.
As the head of Toyota’s self-driving car unit testified before Congress, people tolerate an enormous amount of harm at the hands of human drivers—“to err is human,” after all—but we “show nearly zero tolerance for injuries or deaths caused by flaws in a machine.” For example, when Uber’s self-driving car was involved in a deadly accident in Phoenix, Arizona’s governor shut down the test program. He said nothing about—and made no policy changes in response to—the more than 1,000 deaths on Arizona streets the same year, all at the hands of human drivers. When Tesla’s cars with semiautonomous technology are involved in accidents, people criticize Tesla for beta-testing its technology on public roads. There is barely a mention that the alternative to automation is a world in which more than a million people are driven to their deaths every year—a number that is only increasing. Autonomous technology promises to address the bulk of these accidents—a breakthrough that would constitute one of the most significant public health victories in a century. Yet the message communicated to manufacturers is, “Do not release it until it is perfect.”
Sitting on lifesaving technology merely because it is imperfect is unimaginably costly. A recent study by RAND Corp. shows that in the U.S. alone, hundreds of thousands of lives would be saved if Americans released self-driving cars onto public roads when they are 10 percent better than human drivers, as compared to waiting until they are 75 percent better than human drivers. This is a conservative estimate, because it is inconceivable that the public would even accept technology that is 75 percent better than human drivers.
The same might be said of any other areas where people decline to implement an imperfect machine that is better than the human alternative. This might be true in criminal justice, in hiring, in medicine, in autonomous weapons and so on.
One common response to this line of thinking is that it misses the biggest objection to nonhuman decision-makers: Some people simply have a preference for humans. You might choose to have a human doctor—who is less accurate than a robot—diagnose your medical condition merely because a human is relatable and familiar. This is also an argument the anti-vaxxers make: I should be able to make my choice, regardless of the consequences. Insofar as you are able to internalize the costs of that choice, I think that is fine.
But with self-driving cars, just as with vaccination, it seems unlikely that the costs of your choice will be fully internalized. Your choice negatively affects others. This is all the more true where the preference in question will become a matter of public policy. As I say in the paper:
There is a considerable difference between a patient’s decision to opt for a less effective human surgeon and a state policy barring autonomous vehicles from the road. The costs of robophobia on society are considerably higher in the latter scenario.
Robophobia is a decision-making bias: It interferes with our ability to make sensible policy choices. In the paper I outline a few strategies to “debias” our thinking. The first three are standard anti-biasing strategies: (a) framing devices, such as switching the default option to discourage biased decision-making; (b) education, to teach people to be aware of and less susceptible to their biases; and (c) design choices, such as making robots behave in ways that are more intuitively appealing. The last option is a bit more sweeping:
The fourth and most radical reform would be to remove humans entirely from human-automated systems, which can actually be more dangerous than purely automated or purely human systems. This is exactly the opposite of the standard human-robot interface proposal, where the norm is to insist that a human must remain “in the loop,” to supervise the robot’s performance.
It is odd that the human-in-the-loop prescription is the default solution to human-robot interfaces, given that there is compelling evidence that human-supervised robots can present the worst of both worlds. As I note in the paper, the most rational preference in many instances would be as follows:
Robot only > human only > human + robot
Putting this in the context of automation, partial autonomy may be the most dangerous because of the human-robot interaction effects (mostly problems with human attention), in which case the rational preference should run as follows:
Full autonomation > no autonomation > partial autonomation
If this is right, it would hardly make sense to insist that whatever the state of the technology, a human should always be kept in the loop. But people are terrified of handing control over to a nonhuman decider, so we insist on keeping imperfect humans in charge. Fear of loss of control is one of the key drivers behind robophobia. That helps to explain it, but it does nothing to justify it. We are entering an age when one of the most important policy questions will be how and where to deploy machine decision-makers. In doing so, we must be mindful of our own biases just as we are the biases of algorithms.