The Next Frontier in AI Regulation Is Procedure
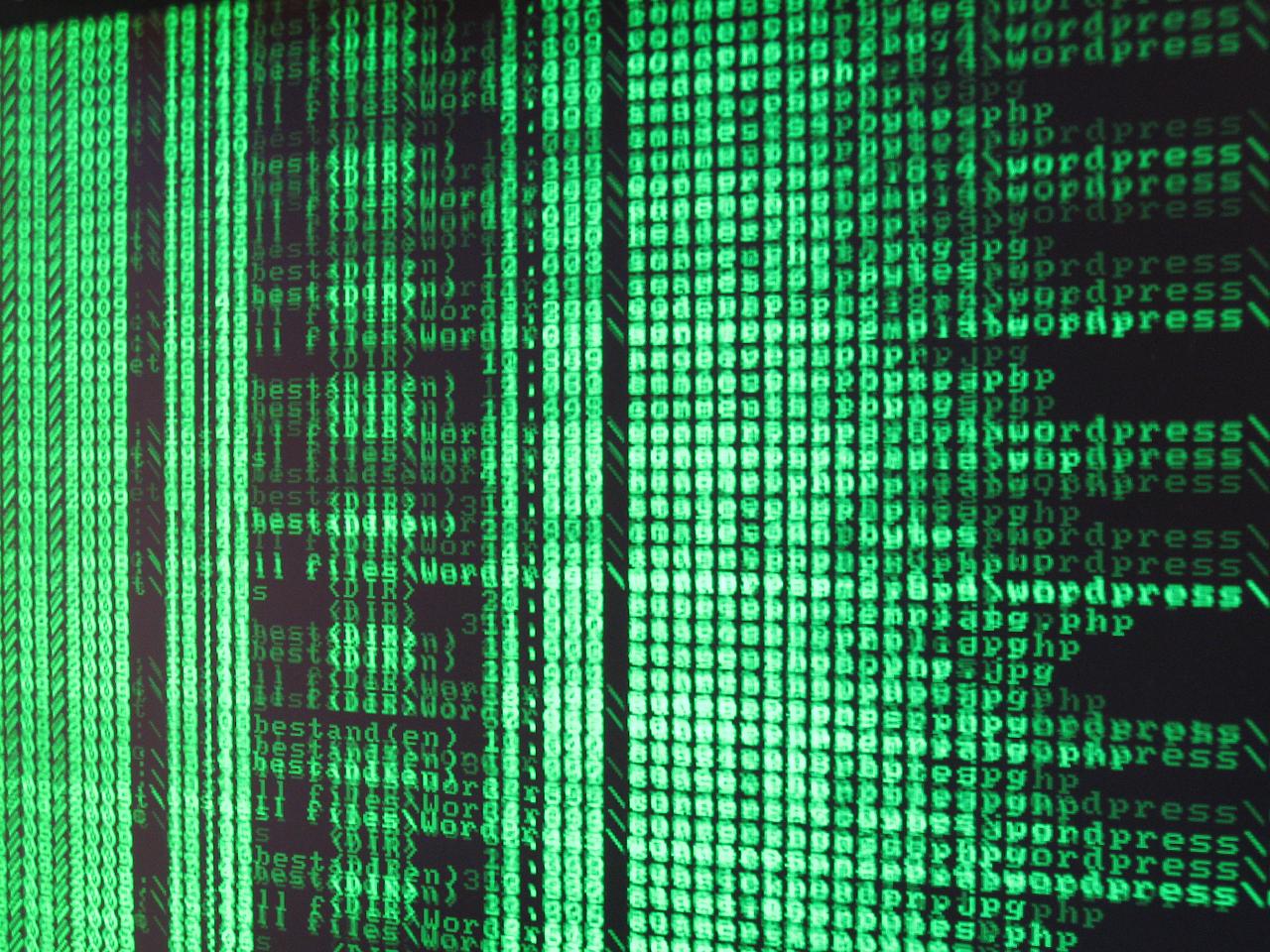
Published by The Lawfare Institute
in Cooperation With
As the promise and dangers of artificial intelligence (AI) grow more evident by the day, observers across the private sector, the media, academia, and government seem convinced that it’s more or less unregulated. Existing laws, they claim, have little to say about this new and radically unfamiliar technology; new ones must be built to control it.
Others beg to differ, especially when it’s the private sector making the argument. These skeptics think we already have plenty of AI laws. What’s really missing, according to them, is the willpower and resources to enforce them. On this view, private-sector calls for new laws sometime in the future seem disingenuous—a PR strategy to come across as responsible while diminishing harms already being caused and resisting any oversight in the present.
The skeptics have a point. Many existing laws do bear on AI, and they can and should play a major role in how society responds to the technology. But on the other hand, the real novelty and far-reaching implications of many aspects of modern machine learning, and the lack of major regulatory action on AI to date, both suggest that today’s laws, on their own, won’t be enough to tackle the massive challenges AI poses—no matter how many resources are committed to the task. Reforms and innovations are needed, and in any event, they’re likely coming, one way or another.
To make these new laws effective, we need to understand why existing laws aren’t likely to cut it alone. Many seem to assume it’s because these laws aren’t “about” AI; that is, they don’t address the specific ins and outs of current AI technology, like training data, large-scale computing, and problems like model bias and hallucination. But these AI-specific issues are relevant to governance only insofar as they relate to potential harms, like consumer deception, discrimination, or threats to safety. Those harms fall well within the purview of many existing laws—from the Federal Trade Commission Act, the Civil Rights Act of 1964, and other federal statutes to long-standing tort laws—and existing regulators and law enforcers, such as the federal and state regulatory agencies, public prosecutors, and private litigants.
If that’s the case, why haven’t these laws made a bigger impact on the AI sector? In part, the devil may be in the procedural details. Historically, outmoded enforcement and adjudication processes have often turned legal protections against the dangers of technology into dead letters. Accordingly, the history of technology regulation in the United States is rich with procedural innovations, from the development of an administrative system for workplace injury claims (as opposed to complex, costly lawsuits) in the 19th century to laws allowing private citizens to enforce environmental and telecommunication laws in the 20th.
Procedure could prove equally pivotal in regulating AI today. Tackling questions like “who enforces the law,” “which evidence counts,” and “who hears disputes” may be drier work than banning facial recognition or launching new global agencies, but history suggests they could make the difference between regulatory impact and irrelevance. Legislatures, regulatory agencies, and the courts alike should start working now to build new procedures and institutions ready for the AI era.
What the Law Already Covers
When many commentators talk about the need to “regulate AI,” they seem to imagine something that is specifically scoped to the technical components of an AI model, like its underlying data or training algorithm. This may help explain why a primary theme in AI regulatory proposals to date involves mandating “algorithmic audits” or data transparency. AI-specific rules and regulations adopted or proposed in New York City, the European Union, and even the U.S. Congress all emphasize these types of interventions. Others have suggested AI risks can best be curtailed by restricting access to or requiring the reporting of large quantities of computing power, another critical AI input.
All plausible enough. But it’s a mistake to assume that “AI regulation” has to have been written with AI’s specific inputs and elements in mind, like controls on algorithms or training runs. Artificial intelligence isn’t the first powerful new technology legislators have confronted. Long before the advent of machine learning, governments were developing rules and doctrines to tackle potentially harmful technologies. Examples include workers’ compensation laws enacted during America’s industrialization, new liability doctrines introduced as railways and automobiles spread, and the slew of federal statutes created in the environmental revolution of the 1960s and 1970s. These generally applicable laws, often radical in their time, have become utterly familiar today—so familiar, it seems, as to be easily forgotten as the sparks of the AI revolution begin to fly.
To see how much these laws still have to say, consider a stylized account of how AI typically unfolds in the real world. First, a company uses data and statistical methods to create an AI algorithm. It builds the algorithm into a product and sells the product to users. The users deploy the product to automate a task, perhaps related to the user’s business, research, or other important activities. In the process, the algorithm in the product may receive and process more data, which may be provided by or related to third parties.
The story of AI in the real world, in other words, is in many ways perfectly familiar. It’s a story about corporate activity, and marketing, and the development of commercial products; about the use and exchange of information; about the ways different kinds of organizations make decisions and take action. And viewed as such, it’s a story with plenty of openings for regulators today, using familiar laws and authorities long predating the rise of AI.
The Federal Trade Commission (FTC), for instance, has long-standing authority to regulate “unfair or deceptive” business practices—practices just as tempting to AI producers today as to the FTC’s targets a century ago. Indeed, the FTC recently announced an inquiry into industry leader OpenAI and, in a series of blog posts, emphasized that this authority covers AI products, especially with respect to models marketed with performance claims that cannot be substantiated, models that are discriminatory, and models that are themselves “effectively designed to deceive” by enabling the production of deepfakes or other deceptive content.
Similarly, the FTC and the U.S. Department of Justice have signaled an openness to using antitrust law against major AI companies. Modern generative AI systems rely on large troves of data and computational power, both of which may be accessible only to the largest tech companies. Acquisitions and cloud computing arrangements between big tech firms like Microsoft and AI startups like OpenAI have already sparked antitrust concerns, as have attempts by some search companies to restrict access to internet search data from rival companies trying to train their own AI models.
But existing legal tools may be thickest of all when it comes to AI’s specific, real-world “end uses.” Take something like discrimination in automated employment decisions. This topic has sparked a large body of AI-specific research and guidance dedicated to operationalizing “fairness metrics,” “debiasing” training data or finalized models, or otherwise intervening to reduce the bias of AI systems. But for purposes of enforcing anti-discrimination laws, it is generally irrelevant whether a human or an automated system is doing the discrimination. The Equal Employment Opportunity Commission uses a simple heuristic called the “four-fifths rule” in cases of employment discrimination: If any protected class passes through a selection process with less than 80 percent the success rate of any other group, the agency will view this as presumptive evidence of discrimination. AI-specific best practices and technical interventions can help keep AI systems from violating the constraint, but it applies to AI either way.
Medical device regulations are another case in point. The Food and Drug Administration (FDA) is charged to ensure these devices are safe and effective, no matter the particular technologies embedded in them. Earlier this year, the FDA used this existing authority to impose detailed testing and labeling conditions on algorithmic lesion detectors used in endoscopy. Additional examples might be drawn from copyright law, internet platform regulation, or other areas. Regardless, it’s clear that there’s already plenty of “AI law” on the books.
If the Law Already Covers AI, Where Is the Action?
With so many opportunities for regulation, why have we seen relatively few AI-specific enforcement actions to date? As a case study of some of the dynamics that may be in play, consider the FTC. With its broad authorities and history of regulating digital technologies, the FTC might seem in pole position to act on AI risks. But so far, despite its many blog posts, the agency has yet to actually claim that any specific AI company has violated the law, though its recent letter to OpenAI hints at possible action in the indefinite future. Gaps like this may help explain why so many observers believe that AI regulation is next to nonexistent.
There are several possible explanations. The FTC may simply be getting up to speed with the latest developments in AI, which in many cases are pushing the technology into meaningful real-world applications for the first time. As a sign of the FTC’s growing interest in AI, for example, the agency announced a new Office of Technology in February. It will be a while before the impact of this new office on the FTC’s actual enforcement behavior can be observed, and likely longer still before other agencies are similarly able to begin prioritizing AI-related issues without dedicated staff.
In addition, agencies tend to be cautious about applying older, often broadly phrased authorities to novel technology. For example, in 2002, the FTC for the first time claimed that egregious failures to secure consumers’ personal information could constitute an “unfair or deceptive” business practice under Section 5 of the FTC Act (the relevant text of which dates to 1938). But although the agency brought enforcement actions against dozens of companies over the following decade, it hesitated to issue formal data security regulations.
It wasn’t until 2015 that a court explicitly held that data security fell within the FTC’s jurisdiction. The FTC v. Wyndham court leaned on the FTC’s history of informal statements and past adjudications as providing “fair notice” to companies regarding their legal obligation to protect consumer data, even in the absence of a formalized regulation.
The FTC may currently be applying this lesson to AI: Before applying an existing law to an emerging technology, first produce a long record of blog posts and interpretive guidance, so that you can demonstrate companies were provided with fair notice. From the perspective of an executive agency like the FTC, this is a reasonable strategy. But it also delays any actual enforcement actions substantially, and may limit how far agencies are willing to apply laws on the books.
Pay Attention to Procedure
It’s understandable that regulators may be cautious to apply broad, existing statutes to new technologies, or need time to turn general authorities into AI-specific dos and don’ts. But we doubt these factors fully explain the dearth of regulatory action so far. Perhaps more important—and easier to overlook—are seemingly mundane procedural constraints that keep existing law from playing a meaningful role.
Here again, history is instructive. Just like the advent of real-world AI isn’t the first time regulators have confronted a new technology, it’s also not the first time the procedural design of existing laws and institutions (as opposed to their substantive scope) prevented them from effectively applying them to that technology and its effects. The procedural innovations that developed in response were often at least as consequential as “substantive” changes, such as explicitly bringing new technologies within the scope of the law.
Consider, for example, the apparently dry topic of standing to sue. Investigating legal violations and enforcing the law in court are costly, lengthy processes. In many cases, regulatory agencies must shoulder the burden alone, because their organic statutes give them alone the authority to sue violators. Some commentators argue that these agencies need more enforcement resources to tackle AI. They do. But as long as they’re the only enforcers in town, it will never be enough. There’s too much happening, too fast, for even the most lavishly resourced agencies to tackle alone.
Lawmakers in the 1970s faced a similar enforcement dilemma with the widespread pollution that America’s industrial development had created. They responded by creating the citizen suit, empowering private individuals to sue polluters who broke the Clean Air Act, Clean Water Act, and other key laws. In the 1980s, advances in telecommunications unleashed pollution of a different sort: endless robocalls and “junk faxes” threatening to swamp American consumers and the federal agencies meant to protect them. In 1991, the architects of the Telephone Consumer Protection Act (TCPA) imposed rules and technical standards on the telemarketing industry and—crucially—empowered individual consumers to enforce them in court. Today, both the TCPA and the environmental laws of the 1970s have significantly reduced the harms they were built to address (though new challenges have inevitably arisen over the years, from “non-criteria” climate emissions to VoIP robocalls). Citizen enforcement played an important role in this success, giving teeth to the law and driving change in how technology was developed and deployed.
The history of workplace safety regulation provides another case in point. Although employers had long been potentially liable for workers’ injuries, actually suing employers was such a burdensome process that the doctrines had little practical impact as late as the 19th century. But as industrialization drove a wave of grisly workplace accidents, state governments eventually swept away the old courtroom procedures in favor of (comparatively) streamlined administrative regimes that paid injured workers without litigation. This procedural shift, coupled with the move to no-fault compensation, helped make redress a reality for those injured in the industrial workplace. Could similar streamlining help vindicate the rights of AI victims without deep pockets—for example, people denied welfare benefits by faulty models, or artists claiming copyright infringement by generative AI?
There were other procedural barriers that kept existing laws from making much of a difference during this era. For example, injured victims in stagecoach crashes could theoretically sue the driver for damages under the common law of trespass. But until the mid-19th century, the laws of evidence blocked any witness with an interest in the outcome from testifying—including the victim, whose testimony would obviously be critical in many cases (especially when it came to harms like pain and suffering). Crash victims also faced byzantine filing requirements similar to those that discouraged worker injury lawsuits, and contingent-fee arrangements with lawyers were illegal at the time, meaning only relatively affluent victims could afford to sue in the first place. Small wonder that personal injury lawsuits were rare. It took reforming these procedural roadblocks, among other changes, to make meaningful recovery possible under the common law.
Considerations for the Way Forward
History shows how seemingly obscure procedural issues—such as who exactly is allowed to sue when the law is broken, whether claims are heard by a judge or an agency, and which evidence is heard—can shape how technology regulation plays out in the real world. In some cases, getting these questions right could matter more than creating new rules and limits on technology, or empowering new regulators to enforce them.
For AI policymakers, the lesson is clear: Sweat the structural and procedural details, both for new laws and for the many existing ones that speak to AI’s challenges. The unfolding deployment of AI will affect the whole of society, from workers whose jobs are changed or even eliminated by machine learning technologies, to already marginalized populations subjected to biased algorithms, to the public as a whole, placed at risk from future AI systems of potentially existential danger. Diverse interests are at stake, embodied in people and organizations with widely varying resources and constraints. It’s critical to think now about how our legal procedures and institutions can efficiently mobilize, protect, and adjudicate among these diverse interests and stakeholders. To that end, before drafting new substantive rules for AI—or at least in parallel to those efforts—policymakers need to think hard about process questions like:
- Who enforces limits on AI? Is the task left to federal agencies, or can state and local governments, private companies, nonprofits, or individual citizens join the fray? Which ones?
- What kind of relief can the enforcers get, and at what point in the process? Can enforcers obtain injunctive relief—for example, forcing AI companies to stop disputed practices—or are fines and monetary damages the main remedy? Do they have to wait until harm has occurred, or can they enforce prospectively?
- Is collective action possible? Can individual victims of AI-related harm “speak” for others similarly situated—and obtain and enforce penalties on their behalf? Or does each victim need to step up individually, potentially diminishing the force of regulation?
- Who has the burden of proof? How much evidence do AI regulators or litigants need to produce, before getting relief—or before the burden shifts to the AI companies or users being challenged? What tools should enforcers have (perhaps comparable to civil discovery or administrative subpoenas) to ensure that material, non-public evidence, such as disputed training data, comes to light?
- Who hears disputes? Are challenges to AI-related applications or outcomes heard in generalist federal or state courts, in administrative fora, or by private arbitrators? How much do these processes cost, how long do they take, and who pays? How knowledgeable are the judges about AI?
The right answers to these questions will vary depending on how AI is being deployed, by whom, and with what potential consequences. But it’s critical to ask these questions in the first place, rather than fixating on AI’s technical particulars. And it’s just as important to recognize that these questions apply equally to the many existing laws that speak to AI, not just hypothetical new ones.
These are questions for Congress and state legislatures first and foremost, as the institutions that create regulatory agencies and have the power to define their enforcement and adjudication procedures. That said, many laws leave agencies discretion to set their own procedures, creating openings for innovation that can be very significant: For example, some federal agencies have created their own class action mechanisms. The same is often true for the courts, particularly at the state level.
Who exactly has the power to act will vary from case to case, meaning that policymakers of all sorts have roles to play in making sure legal procedures are up to AI’s challenge. But none will be the first to confront the profound consequences of new technology. America’s history of technology regulation suggests that the rise of powerful AI is an opportunity for procedural experimentation and reform. Policymakers should seize that opportunity to make the most of the tools they already have and ensure the success of the new ones to come.