Time to Act: Building the Technical and Institutional Foundations for AI Assurance
AI assurance requires agreement among governments that systems are behaving appropriately. Existing international standards institutions can help.
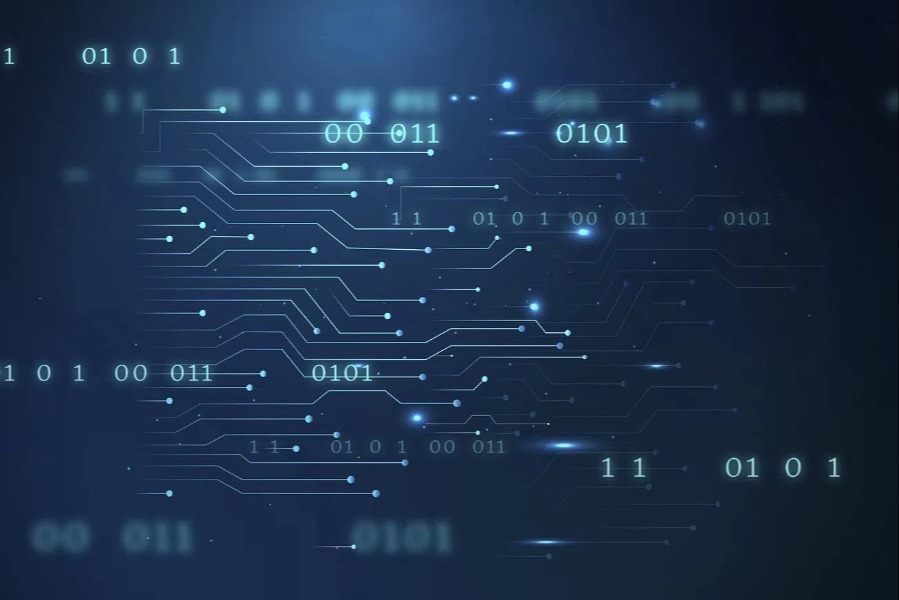
Published by The Lawfare Institute
in Cooperation With
In March 2022, an Indian BrahMos missile launched from Sirsa landed in a sparsely populated region of Mian Channu in Pakistan. The episode, which India described as an accident, fortunately, caused no casualties but led to fears that Pakistan might respond in kind, fueling escalation. A few months later, similar uncertainty surrounding the origin of a missile that exploded in Przewodów, Poland, during a particularly acute period of Russian bombardment of Ukrainian energy facilities triggered fears that NATO might find itself at war. And perhaps the most famous close call was the nuclear false alarm in 1983 when a Soviet early warning system detected the launch of five U.S. intercontinental ballistic missiles. Stanislav Petrov’s decision to ignore that warning is credited with avoiding nuclear war. The integration of artificial intelligence (AI) tools for targeting and engagement, as well as early warning and decision support functions, might make these close calls more common. In light of this increased risk, efforts to increase confidence that AI-enabled systems are acting as designed become increasingly important.
While the challenges facing policymakers in the realm of AI governance are significant, they are not insurmountable.
Over the past several years, analyses of potential future conflicts have stressed the growing role that autonomous systems will play across domains and functions. As these systems are integrated into global militaries, it is increasingly likely that accidents could lead to escalation (inadvertent or intentional) in the face of improperly tested and evaluated platforms. Collaboration with both allies and adversaries on testing and evaluation has the potential to reduce these accidents and the consequent escalation of conflicts, driving compliance with international law. Establishing international standards and norms about the employment of AI in safety-critical contexts is the prudent way forward for this collaboration.
Various governance efforts, from the current U.K. summit to various UN processes (e.g., in the UN General Assembly and First Committee) to U.S.-China Track 1 dialogues, offer states opportunities to address these risks. As governments across the globe struggle to address the new dangers posed by AI technologies—see most recently the White House’s Executive Order on the Safe, Secure, and Trustworthy Development and Use of Artificial Intelligence—and as various governance proposals—from the OECD AI Principles to the G7 Hiroshima Process—struggle to gain traction, we argue for a focus on how AI assurance might be achieved by leveraging existing international standards bodies, involving both governments and firms.
AI Assurance
Assurance for AI systems presents unique challenges. While traditional software allows engineers to develop mental models of its behavior, guiding testing and evaluation procedures, AI often includes corner cases, where a system behaves in an unexpected way when presented with new situations, which can be difficult to predict and incorporate into a testing regime. For example, developers and testers of large language models (LLMs) have found that “adversarial suffixes,” appended to LLM prompts and often appearing like gibberish, enable users to bypass guardrails and access dangerous information. Even in smaller AI systems, like those for image recognition, unexpected (and possibly malicious) inputs can lead to incorrect outputs that degrade performance and lead to dangerous behaviors.
These barriers and other similar ones have led major AI companies to establish teams focused on AI safety, employing practices like red teaming to discover unexpected behaviors and develop fixes prior to deployment. It is important to distinguish this work—ensuring correct performance in the face of novel operating conditions—from capability development, where the goal is to maximize the achievement of some goal using a particular AI system. These two aims often overlap but represent distinct objectives.
As one of the authors has noted elsewhere,
This landscape is further challenged by typical state behavior at the international level. States are often reluctant to engage in cooperative security agreements that require too much transparency into national capabilities. They are even less likely to place limits on the development of their own capabilities in the absence of any guarantees that their adversaries will do the same. However, because performance and safety research are two different research streams, it is possible to coordinate on security while limiting availability of research into performance improvements. This unintended silver-lining is known to AI labs, which is why commercial labs are often willing to open-source safety research into evaluations and provenance technologies.
Fortunately, AI models in particular, and algorithms in general, provide a level of abstraction that enables the development of assurance techniques that can be applied across systems employed in unique use cases. Neural networks, one architecture for machine learning that is helpful in recognizing structure in data, can be applied in a range of tasks from medical diagnostics to intelligence analysis, and their fundamental structure enables techniques used for testing them in one context to be adapted for others. It is this property that permits a capability to be separated from its assurance procedure, allowing scholarly work to disclose novel methods for evaluation without disclosing the methods (in training and engineering) that enable performance.
AI companies have recognized this fact, publishing safety research readily while closely guarding information about model structure and training data. This property applies well beyond the LLMs that have grabbed significant public (and investor) attention, spanning across the aerospace, biomedical, and defense industries. Critically, the transferability of techniques that improve AI assurance across domains can permit procedures developed in one use case (such as for assurance of biomedical devices) to apply to other, distinct use cases (such as intelligence analysis), when the AI techniques underpinning these uses overlap.
One of the greatest challenges in developing assurance for complex safety-critical systems lies in specifying the properties that a system is expected to uphold, distilling high-level goals into low-level rules that bound permitted behavior (the “specification problem”). For autonomous systems, this becomes even more complex because of vague requirements—like “fairness” and compliance with international law—which lack common and rigorous mathematical definitions that are amenable to traditional testing and evaluation. While low-level specifications may be closely coupled with goals for performance (such as decision-making that will maximize some advantage), high-level specifications are more agnostic to these objectives and focus instead on complying with ethical and responsible AI guidelines. Collaboration on these high-level “rules of the road” might create safer and more compliant AI systems, while avoiding disclosure of the engineering enablers that yield advantages in conflicts.
While governments face distinct challenges as they strive to develop AI supremacy across both military and civilian domains, AI firms present a useful analogy in understanding the distinction between collaboration on safety engineering and capability development. Despite their competition, companies like OpenAI, Anthropic, and Microsoft have been open to sharing information about the safety measures they employ in developing their AI models, providing assurance that they have stress tested their systems prior to deployment and thereby contributing to the research ecosystem by pushing forward novel and potentially useful techniques for enhancing safety.
A similar dilemma faces state actors: While exposing the enablers of capability development has the potential to enhance the performance of adversary systems, discussing assurance techniques can instill confidence in the safety of a state’s platforms and establish norms for the level of rigorous verification that is required to employ AI in safety-critical use cases. These potential norms have a parallel in the initial set of voluntary contributions made by leading AI firms in the United States in recent months, which may drive the rest of the industry (at least domestically) to follow suit in establishing rigorous standards for the development and release of AI technologies.
With the United States and China leading the ongoing “AI race” among states, collaboration between Washington and Beijing on rigorous methods for testing and evaluation, beyond LLMs and extending into the range of safety-critical use cases of AI, has similar potential to establish a precedent for the international community about the safe employment of AI—particularly in the military context. While it may be tempting to disregard China’s contributions in this discussion and expect one-sided information sharing on safety from the United States, contributions by Chinese scholars in this area suggest that U.S. firms and government developers can glean useful insights by exchanging technical information. Although it remains an open question how these standards and principles are being operationalized in Chinese commercial and military AI, both government documents and scholarly work suggest that AI safety remains at least a nominal priority in much of the Chinese AI ecosystem. Moreover, both ecosystems stand to benefit from the creation of standards that offer assurance that AI systems are behaving appropriately and from venues positioned to address crises in which incidents involving AI systems occur. Recent announcements of Chinese participation in global AI safety discussions as well as bilateral dialogues related to arms control should generate some optimism in these contexts.
The Nuclear Analogy: Information Sharing
The challenges associated with building institutional arrangements that are responsive to the specifications above are significant, but information-sharing arrangements and the use of technical standards to manage risk have a long history—even among adversaries. Below, we outline various historical cooperative security arrangements where states have sought to limit the proliferation and application of technologies, even as they are being developed.
Throughout the nascent AI governance debates, the nuclear analogy has loomed large—in some cases usefully, and in other cases less so. With that said, there are less-discussed examples from the nuclear past that might be worth considering. Here, we examine three examples that demonstrate the value and innovation in information-sharing regimes over time.
The first, the Threshold Test Ban Treaty (1974), followed the Limited Test Ban Treaty (1963) signed by the Soviet Union, the United States, and the United Kingdom, and prohibited nuclear weapon testing in the atmosphere, outer space, underwater, and underground. The Threshold Test Ban Treaty sought to prohibit testing above a specific “threshold” (150 kilotons). This threshold has a strategic imperative, effectively reducing the yield of reliable, tested warheads. To confirm adherence, the agreement includes a protocol in which technical data is exchanged between states parties, including detailed information related to the geographical and geological characteristics of testing areas. In 1990, provisions that included seismic monitoring and on-site inspections were added to facilitate U.S. ratification of the treaty.
The INF Treaty, which required the destruction of U.S. and Soviet ground-launched ballistic and cruise missiles with ranges of between 500 and 5,500 kilometers, also provided for information and data exchanges in addition to on-site inspections. This arrangement formalized data exchanges at six-month intervals and institutionalized information sharing within Nuclear Risk Reduction Centers—an innovation that has also been discussed in an AI context.
Finally, the recently collapsed New START Treaty further institutionalized biannual nuclear data exchanges and the creation of a database of treaty-required disclosures to serve as a clearinghouse for the numbers, locations, and technical characteristics of weapons systems and facilities. After the treaty’s collapse, the United States has continued “to provide more general public information on its arsenal and daily notifications on the basing location of treaty-accountable missiles and upcoming strategic exercises,” and Russia has pledged to “continue to send notifications of launches of intercontinental ballistic missiles and sea-launched ballistic missiles under an ongoing 1988 U.S.-Soviet agreement.” These arrangements can be persistent—even in the face of treaty collapse and broader geopolitical tensions.
Taken together, these examples suggest that there is hope for information-sharing regimes, even among adversaries. But there are important differences between nuclear and AI technologies, not least that the proliferation of the latter is likely to be far more widespread and involve entirely new sets of actors.
The Nuclear Analogy: Getting the IAEA Right
Given the proliferation of AI technologies and as the notion of an “IAEA for compute” gains traction, it is also worth noting the various mechanisms through which an intergovernmental organization can support the setting and dissemination of technical standards. While we share some of the skepticism about the broader “IAEA for compute” analogy—preferring an institution that has universal membership, does not have a history of state withdrawal, and does not identify “haves” and “have nots” at the outset—there are useful arrangements within the IAEA architecture that get lost in the contemporary debate. Below, we consider three examples of institutions within the nuclear architecture that offer productive models for addressing the challenges outlined above.
First, the Unified System for Information Exchange in Incidents and Emergencies (USIE) maintained by the IAEA enables countries to “exchange urgent notifications and follow-up information” during a crisis or emergency with over 1,000 users from over 150 member countries registered in the USIE system. The USIE also allows member countries to “request information or international assistance” during an emergency. As commercial players in both the cybersecurity and AI spaces have already noted, incident-sharing agreements serve the dual role of providing notification that a system is malfunctioning (to avoid unintended escalation, especially in the face of a cascading failure across systems) as well as warnings about how other systems might be vulnerable to the same attacks or failures. In the latter case, distinct AI systems have already demonstrated sufficient similarity in engineering to enable vulnerabilities to be exploited across them.
Another useful model is the Incident and Emergency Centre (IEC), which seeks to “inform many different official designated actors around the world as quickly as possible whenever a nuclear or radiological emergency occurs.” The IEC serves as an independent arbiter and, ideally, provides a reliable information flow during crises. In our view, any applications of the USIE or IEC models in AI governance would need to consider seriously the role of private industry. Significant commercial development of AI technologies has already convinced many in government and the military that they will likely be adapting commercial tools, rather than developing their own (as in the Defense Department’s adoption of the “fast follower” approach to AI development).
Finally, the Incident and Trafficking Database (ITDB) offers a more passive mechanism for participating member states and international organizations to exchange information to “combat illicit nuclear trafficking and strengthen nuclear security.” Data submitted to the ITDB includes illegal trade or movement of nuclear material, unauthorized acquisition, loss of material, and discovery of uncontrolled material. The purpose of these submissions is to identify patterns in the data to deter “potential security threats and vulnerabilities.” Tracking the development of significant AI systems employed for malicious purposes through a similar mechanism may provide comparable benefits to states, such as when AI is employed by a non-state actor for purposes like the large-scale dispersal of misinformation or disinformation.
Weighing Multistakeholder-ism
The multistakeholder model of internet governance provides another useful framework for the development of collaborative initiatives to promote AI safeguards. This model has evolved as a dynamic and inclusive approach to address the intricate challenges of the global digital landscape. With its emphasis on open participation, transparency, and consensus-based decision-making, it has fostered an environment where stakeholders from diverse backgrounds—including technical experts, governments, and civil society—come together to shape the internet’s future. This engagement has fostered the collaborative development of technical standards, policies, and solutions that protect the internet while accommodating a diverse set of interests—including those of users, internet service providers, commercial firms, and governments. A variety of institutional arrangements have been created to support this burgeoning governance regime.
The Internet Corporation for Assigned Names and Numbers (ICANN) is charged (originally the Internet Assigned Numbers Authority (IANA) under the auspices of the U.S. government) with managing the traffic of the internet via the management of the global domain name system via its stewardship of top-level domains (e.g., .com, .org., or .co.uk). Today, ICANN is a global platform for internet policy and coordination, emphasizing diversity and the multistakeholder model. ICANN’s multistakeholder approach involves consultation with governments, businesses, technical experts, and civil society organizations—and has recently become a venue for attempts by governments to grow their role via ICANN’s Governmental Advisory Committee that communicates the interests of governments and intergovernmental organizations. Like ICANN, the Internet Engineering Task Force (IETF) was founded as a multistakeholder institution in 1986 to create technical standards through open, consensus-based processes. Past successes include the development of protocols like HTTP/1.1, IPv6, and TLS, each of which has had a significant impact on the internet’s architecture and security.
As opposed to the arrangements above that include many different types of actors, the International Telecommunications Union is primarily a state-based entity (the UN body responsible for information and communication technology). Traditionally, the ITU has managed global radio spectrum and satellite orbits but has recently become more involved in internet governance discussions—with some states increasingly supportive of bringing some of the decision-making apparatus in the entities above into the ITU orbit, where governments are likely to have a more significant voice. This shift would, of course, have significant downstream consequences, not least given that “one country, one vote” decision-making might shift the priorities of internet governance discussions considerably. Any AI governance regime that seeks to play at the intergovernmental level ought to consider the voting structure.
Notably for our argument that these types of regimes should be deployed in AI contexts, these regimes were developed by a variety of individuals and entities—scientists, engineers, academics, private firms, and government actors. Additionally, both internet governance and AI are layered domains, with regimes (and their membership) ultimately fighting for primacy with one another. Taken together, these examples of nuclear and internet governance collaboration demonstrate that international cooperation focused on technical standards is possible even in competitive contexts, but what might it look like when applied to AI specifically?
Toward Technical Standards
There are already international institutions that might be relevant to this mission. The International Organization for Standardization (ISO) helps to shape information-sharing regimes related to AI technologies on a global scale. Its efforts in developing AI standards—measures agreed upon by subject matter experts and codified—have far-reaching implications for the industry, as they facilitate interoperability and compatibility among AI systems and enable data and knowledge sharing across borders and industries via certification. ISO standards attempt to foster innovation, reduce barriers to entry, and promote the responsible and ethical use of AI. They address concerns surrounding data privacy, security, and ethical considerations, contributing to a more harmonized and trustworthy AI ecosystem.
Of immediate relevance to AI assurance, the ISO/IEC JTC 1/SC 42 - Artificial Intelligence serves as a standardization subcommittee established by the Joint Technical Committee ISO/IEC JTC 1 of the International Standards Organization and the International Electrotechnical Commission. The subcommittee’s standards prescribe specific procedures for the assessment of machine learning performance, ensuring data quality for analytics and addressing the complete data life cycle. The subcommittee’s work focuses in part on critical themes that have become important aspects of broader AI governance debates—including explainability, transparency, and controllability of AI systems. Importantly, the ISO, and the national-level standards bodies of which it is composed, serve as a vehicle for the voices of scientists and engineers to shape regulation and offer a venue where technical discussions are already the norm.
The Time Is Now
At the top of this piece, we outlined various scenarios that might have led to disaster and noted the possibility that these scenarios might be more likely to occur with the proliferation of AI capabilities. Tackling this risk requires a combination of assurance mechanisms that enhance the safety of AI-enabled platforms. Establishing international standards and norms surrounding the employment of AI in safety-critical contexts reduces the likelihood of these mishaps occurring and drives (in the case of military systems) greater compliance with international law. Meanwhile, incident reporting mechanisms would also enable states to receive a quick and reliable signal that an AI system has malfunctioned, facilitating deescalation.
The dual-use nature of AI, as well as the major impact of commercial systems on military platforms, prevents us from simply focusing on the most glaring, military-specific use cases. It is beyond time for policymakers (and AI scientists and engineers) to formulate precisely the international institutional mechanisms and domestic and international policies that can enhance AI safety and trust, before catastrophe strikes.