What Do Transparency and Data Sharing Really Mean?
Transparency reporting and data sharing aren’t the same. They aren’t even the right words.
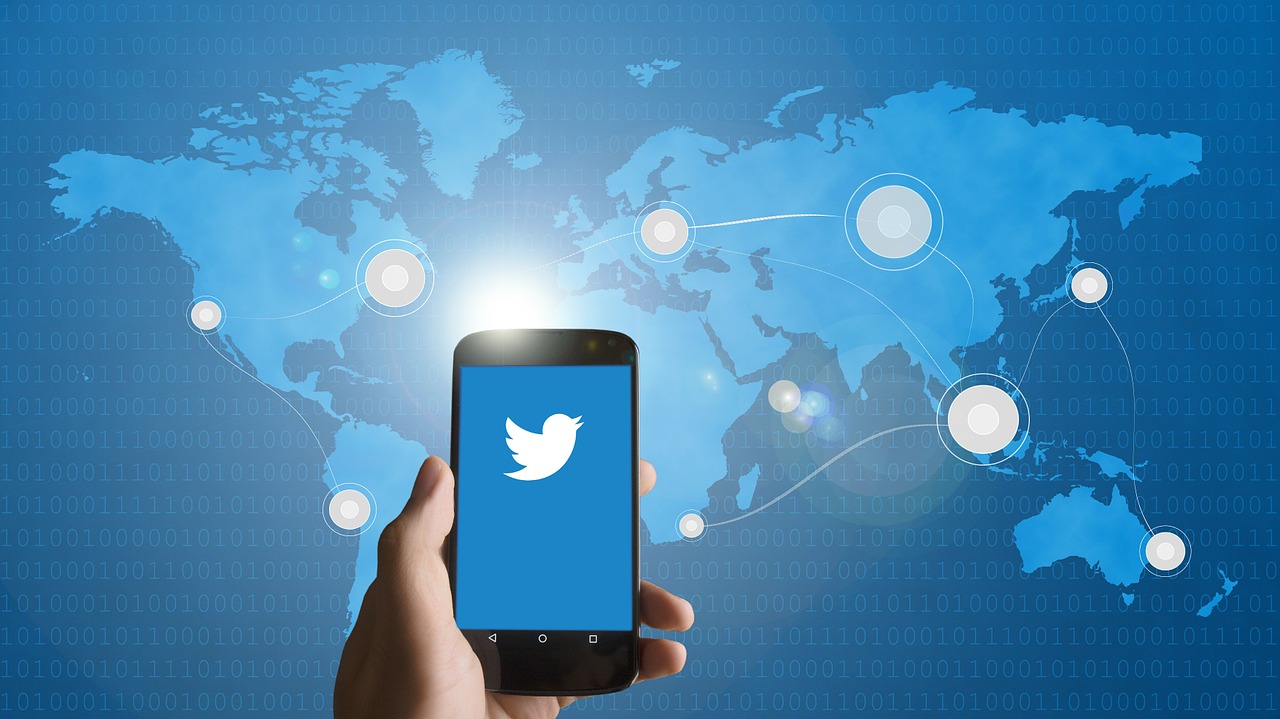
Published by The Lawfare Institute
in Cooperation With
Transparency reporting and data sharing are hot topics. Both are seen as holy grails to unlock the mysteries of the information environment. And both are often conflated with each other. This lack of clarity in definitions makes it challenging to draft much-need regulation to help drive either concept forward in practice. As regulations like the EU’s Digital Services Act (DSA) and the Platform Accountability and Transparency Act (PATA) in the United States move forward, perhaps it’s time to stop and ask—do we have the right words to succeed? Indeed, neither of these draft laws defines transparency, transparency reporting or qualified data. Turning to policy proposals is unsatisfying with a lack of consensus there as well. Further, the reporting on and sharing of data with researchers, policymakers or the public involves sharing information and data with varying degrees of sensitivity and privacy. Not all data can be made available to the public. Beyond definitions, processes must be developed to determine who should have access to what types of reporting and data and for what purposes.
“Transparency” is a loaded and widely contested term. For some, transparency reporting is narrowly tied to government disclosures. This type of reporting is aimed at accountability around government practices, such as how governments use platforms for civilian surveillance. For others, transparency reporting has become a means to hold big tech companies accountable. Often this viewpoint is tied inextricably to the act of data sharing, with the aim of giving more researchers access to data to better understand the influence of technology on humans or the effects of threats within the information environment. Given the messiness of these definitions, might a slight reframing help bring clarity?
Are We Actually Talking About Operational Reporting?
There are increasing calls for disclosure by companies about how policies are crafted and enforced or how algorithms function. While sharing information about such things would certainly increase transparency, these and many other categories proposed just shed light on the operations of tech companies. Thus, instead of transparency reporting, perhaps what is really needed is operational reporting, or the aggregated reporting by online services about aspects of their operations. Such reports would not offer up individual user data but, instead, would present both quantitative and qualitative analysis drawing from aggregated statistics to explain how a company and its services operate. Such reports would need to be issued on a regular and consistent basis (for example, annually) and provide the same types of information over time, ideally with guidance and oversight from a regulator or an independent third-party monitor. While operational reporting helps with transparency, an independent auditing and impact assessment process would be needed to verify the accuracy of such reports to rebuild much needed trust within the modern information environment.
Companies have been engaging in some operational reporting, but, of course, given the current climate of mistrust, more could be done. For instance, Meta routinely reports takedowns of coordinated inauthentic behavior (CIB) on its platforms. The latest report, published in January 2022, listed the number of CIB networks taken down. For each network, Meta listed the number of accounts, pages, and groups removed, the countries involved and targeted, specific actors to whom the CIB could be attributed, and the audience reached by each network. Twitter has released similar reports disclosing state-linked information operations on its platform. Similar to Meta, Twitter’s disclosures include the number of operations, the countries and actors involved, the number of associated accounts linked to an operation, and the intended purpose of an operation, when known.
While these are helpful first steps, a lot more could be included in these operational reports. For instance, both platforms mention sharing datasets associated with their takedowns with a select group of researchers. What they don’t share is how those researchers or institutions were selected as partners, how they chose what data to share with researchers, or whether data shared with research partners was different from that shared publicly. Twitter cites its platform manipulation and spam policies as the reason for takedowns. But they don’t discuss what parts of the policies were violated or how those policies were developed in the first place. They also fail to reveal whether the impacts of those associated enforcements were measured and, if so, what the findings were.
How often takedown reports are released varies by platform. Meta moved from releasing CIB reports only as networks were detected to issuing nearly monthly reports starting in 2020. Now, they’ll be publishing quarterly adversarial threat reports. Twitter, by contrast, still releases reports as and when it detects state-linked information operations on its platform. For meaningful operational reporting, platforms must commit to releasing their reports consistently, be it monthly, quarterly, semiannually or annually. Ideally, they’d also offer trends over time.
Free the Data!
While operational reporting over time might better inform policymakers and the wider research community on what data is available from companies, the concept is distinct from data access itself. “Data access” is an umbrella term covering the various means by which raw data is made available to researchers. “Raw data” refers to the actual posts, comments or threads on the platform of an online service, as well as data related to behaviors associated with that content or platform use. It could include engagement metrics (likes, shares, retweets, etc.) and aggregated demographic and location statistics. Adding demographic and location statistics to raw datasets, however, would amplify user-privacy concerns and would require additional safeguards to methods of data sharing.
Data access can be broken into two components: data sharing by online services and data acquisition. Companies engage in data sharing when they provide raw data that researchers can manipulate themselves for research purposes. Such data can be made available through a variety of means. It could be shared directly in regulated environments, though data is more often shared indirectly with researchers through public application programming interfaces (APIs) or other instruments for sharing large datasets. Twitter’s datasets of accounts and posts associated with state-linked influence operations, which include the posts, media, and engagement metrics, are an example of such raw data. Given how little data is currently shared, especially in comparison to increasing data access, companies should also engage in operational reporting by explaining the policies and processes behind how data is shared.
Researchers often resort to their own data acquisition, due to the aforementioned lack of data sharing by tech companies. “Data acquisition” refers to all the other means researchers can use to gather data. Internet users can donate their data directly to researchers, at times by simply adding a browser extension. For example, New York University’s Ad Observer allows users to install a browser extension that enables researchers to collect ads that users see on their Facebook and YouTube timelines. Often researchers gather data on their own by scraping posts and comments from social media platforms, or they enlist third-party service providers that gather the data for them. One such service provider is Pushshift, which collects comments and submissions from Reddit and makes that data available to researchers. Indeed, much data is already made available by third-party social media monitoring or listening companies.
These third-party service providers offer their customers user-level and aggregated demographic, location and content data. Brandwatch is another example of this kind of provider, gathering data mostly from Twitter and Reddit. Talkwalker, a social listening company, provides its customers with Twitter and Weibo users’ demographic data, such as their age, gender, and family status, as well as content data, such as interests, pictures, and videos.
The Devil Is in the Detail
These companies use this social media data to provide market and consumer research tools to their customers. Similarly, many of the social media companies themselves enable advertisers to target users by demographics, location, interests and conversation topics. A question often arises: If third-party services and advertisers can access this data, why can’t researchers? Data access for researchers could involve sharing similar types of raw data—posts, threads, comments, and associated anonymized demographics and locations of users engaging with those posts. Privacy and security are often raised as reasons not to share such data with more researchers, which only reinforces the need to gain more clarity around definitions related to operational reporting, data access, and rules for facilitating both.
Some information and data are more sensitive than others. It is possible that not all operational reporting or types of data can or should be made publicly available. Balancing users’ privacy with data sharing that enables research on the information environment is imperative. Appropriate vetting of researchers who access social media data, tiered access to data based on sensitivity of the data, anonymization of data, and use restrictions are some mechanisms that can help strike that balance. Similar categorizations for operations reporting might also be required. Developing processes for this tiering and vetting is no small feat, but to achieve greater accountability and a deeper understanding of how the information environment works, a detailed regime is needed. And given the gaps in existing regulation, those details were needed yesterday.
Indeed, researchers have been calling for tiered access to sensitive data for several years. Margaret Levenstein, Allison Tyler, Johanna Bleckman and Nyanza Cook at the University of Michigan suggested creating a “data passport” that determines the level of sensitive data a researcher can access. Similarly, Latanya Sweeney, Mercè Crosas and Michael Bar-Sinai recommend “datatags” to rate data from a scale of public to maximally restricted. The more sensitive or private the data is, the greater the restrictions needed in terms of who has access and for what reasons. For example, sharing data related to fake accounts used to run influence operations should be easier to share than data about real users. Similarly, hashed data, a process Twitter uses to hide certain identifying details of users, could be more widely accessible to researchers than unhashed data. This tiering of access, however, requires the development of rules by an independent body to ensure fair and inclusive access for an array of researchers.
Similarly, operational reporting might fall along a range of access. Some reporting could readily be made public, such as on how terms of services are generated and updates are made, or on advertising, outlining what types of advertisers are buying what kinds of ads, targeting which audiences, where and in what languages. However, some reporting, for example, about how the platform is designed and works, might be available only to regulators or vetted researchers, given concerns with both competitive intelligence and how threat actors might attempt to game those services with that knowledge. Again, such a regime requires details, which—given the implications for wider society—cannot be sorted out in a single article or by any single stakeholder. They require consultations with a clear and focused outcome aimed at developing a process.
Distinguishing between operational reporting and data access is important for industry, policymakers and researchers alike. With a clear conception of what operational reporting and data access entail, regulators and policymakers can adopt those definitions into legislation. Common definitions and approaches could be adopted in multiple regulations, helping harmonize approaches across countries and legal jurisdictions. Such a move would increase the likelihood of success in implementation, reducing the burden on companies to comply with various laws around the world and removing an excuse for them not to do so. These definitions can open a path forward to developing processes to make more data available for researchers and policymakers to develop evidence-based policy for countering threats within the information environment. But this requires clarity and goes beyond vague calls for more transparency, to more nuanced thinking through how such a system would work.