On AI-Generated Works, Artists, and Intellectual Property
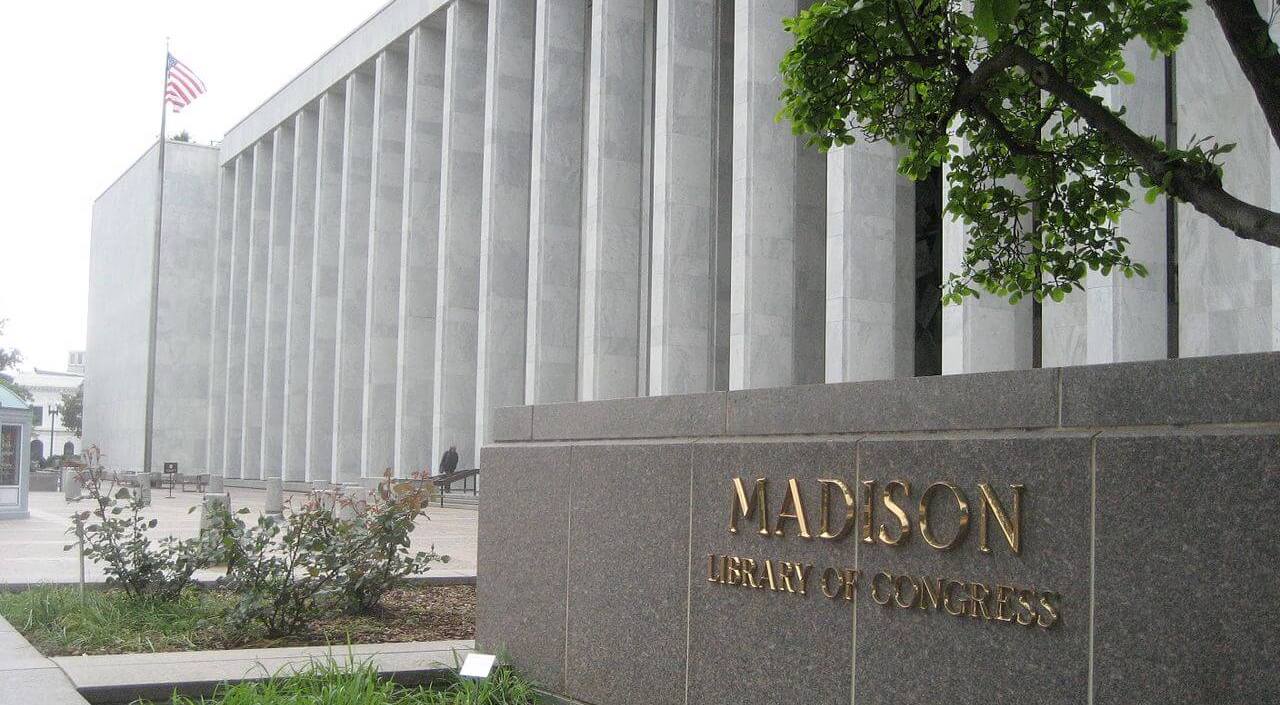
Published by The Lawfare Institute
in Cooperation With
As lawsuit-inspiring musicians go, you can’t do much better than 2 Live Crew. Infamous for their 1989 album, “As Nasty as They Wanna Be,” they were the first band to ever have an album deemed legally obscene (though the decision was later overturned), and they were sued successfully by George Lucas over their trademark-infringing label name, Skyywalker Records. But it was their worst-selling album that helped redefine an obscure element of copyright law that will be essential to the development of modern generative artificial intelligence (AI).
“As Clean as They Wanna Be,” released in 1991, was the much-less-titillating version of 2 Live Crew’s original “Nasty” hit album. The revision featured no explicit lyrics and covered up all of those skimpy bikinis on the cover art. It did, however, include a new parody of Roy Orbison’s classic “Pretty Woman.” Oribson’s label sued, and the case made its way to the Supreme Court, where the justices sided with the band and added one small but important bit of case law to U.S. copyright.
Justice David Souter wrote that the Court made its decision in part based on whether the new work “adds something new, with a further purpose or different character, altering the first with new expression, meaning, or message; it asks, in other words, whether and to what extent the new work is ‘transformative.’”
Nearly 30 years later, that decision stands as a turning point in copyright law, allowing everyone from artists to software developers to explore new and expansive uses of content belonging to other creators without permission.
Creative AI has arrived, and it will transform art, industry, and copyright forever. The opinion pieces and breathless Twitter threads on generative AI frame it as a heist thriller, but it’s not that simple. The story of generative AI is built on the historical undervaluing of art in our society, twisted and confused by the byzantine elements of copyright law, and torqued up to blockbuster-movie intensity by the looming reality that robots will soon be able to compete with, or even replace, human creativity.
While these market-destabilizing innovations demonstrate meaningful advancement and spectacular ingenuity in science, they also raise new questions about the nature of creativity in an age of machines that can make art. These new innovations will have impacts far beyond art and culture. They will reshape economies, pose new threats to national security, and force society to redefine the nature of trust and creativity itself.
There are a lot of trade-offs, and no easy answers. Artists and creators will be harmed economically, and it’s nearly certain that industries will be disrupted or even destroyed, although perhaps it’s too early to say which ones and how soon. It’s possible that the solution is right in front of our faces: Governments should restrict copyright to humans, and deny it to computer-generated works.
What Is Generative AI?
Generative AI is the process of training a machine learning model with existing content to create new works. Through millions of trials and errors, the model learns from the content that is fed into it and uses what it “knows” to generate a new piece of work based on written prompts by users.
The training data is largely pulled from the internet. If it’s online—articles, photos, drawings—it’s probably been scraped up and fed to an algorithm to teach it to write, speak, and make art. ChatGPT, developed by Microsoft-backed AI research company OpenAI, was trained on text-based content found on the internet—from the complete text of Wikipedia, to Reddit threads, blog posts, and more. The image-generating AIs like DALL-E and Stable Diffusion operate on a similar principle, trained on the huge corpus of images that can be viewed on the web. For example, the AI Stable Diffusion, which uses the LAION 5b database, contains references to more than 5.85 billion images.
How do they do it? They use automated systems to scrape the internet. All of it. The developers didn’t ask anyone for permission, but, to be fair, this practice of web crawling is how things like search engines have worked for years. Google crawls the web daily. It scans some sites, like Wikipedia, in nearly real-time; others, it visits over a cadence of weeks. The Internet Archive’s Wayback Machine crawls sites and takes snapshots of them as a public service. Common Crawl scrapes the web and makes its archives available for others to use. (Disclosure: When I led the development of CC Search, an open content search tool, now rebranded as Openverse as a part of Wordpress, at Creative Commons, we relied on the Common Crawl database to find nearly 2 billion openly licensed works on the web, including images across dozens of platforms and archives.)
The LAION database creators crawled the internet in the same way, looking for images, and then produced a massive list of URLs pointing to every one it found—on Flickr, on Wikipedia, etc. Then they fed those links to a computer vision algorithm that “viewed” the images and assigned descriptive text to them, creating word associations that go with the images. That’s called CLIP data: Contrastive Language-Image Pre-training. The algorithm looks at an image of a banana and assigns text like “banana,” “yellow,” “fruit,” etc. It might also assign more relational tags for things that go with bananas, like monkeys, or comedy. The deeper the list, the better the CLIP data, which will then be used to respond to the user’s prompts.
Diffusion is the technology that underpins the image-generating tools. Its model is trained on an iterative process in which random noise—static, or random pixels—is added to an image, and the algorithm is tasked with removing that noise to restore the original image. After thousands of iterations, the model learns how to remove noise efficiently. Each time, more noise is added until ultimately the software can return a suitable image from 100 percent noise. The developers can then direct the software to do that process in reverse, asking the algorithm to create an image starting from 100 percent noise. The software uses what it knows from all of those images with descriptive text to tell it what that final image should look like.
To generate images from text prompts, the AI deploys a series of tools (this is a great, though complex, visual explainer on how Stable Diffusion’s model works). In short:
- A user submits text through the interface.
- The model interprets the text and looks in its CLIP database (text descriptions of the images it has seen—some specific, some abstract).
- The model uses the diffusion process to begin with a noisy image, and diffuse it into something that matches the kinds of images associated with the CLIP data sets—the billions of images it has “seen.”
- The model “de-noises” those reference images to make something new and then refines it into an image, or set of images, that it presents to the user.
Copyright Belongs to All of Us
AI generators are trained using existing works, usually without asking permission, so the question of copyright is important. Some creators question whether the companies creating the machine learning models had a legal right to use their works. The answer turns on questions of fair use and a concept within it called transformative use.
Copyright, as it was originally intended, affords an exclusive right to the creator of an original work to exploit that work as they choose for a limited period of time. After the term of copyright expires, a work enters the public domain, which allows it to be used by anyone or any company, without restriction, permission, or attribution, for any purpose.
Today, copyright in most countries lasts the author’s entire lifetime, plus 70 years. So if you create a work at 30, and then live to be 60, it will be under copyright for you (and your heirs) for 100 years before it enters the public domain.
But there are a number of ways that individuals can use a copyrighted work without seeking permission. That was by design, to promote innovation and creativity. In the United States, the fair use doctrine allows the exploitation of a copyrighted work “for purposes such as criticism, comment, news reporting, teaching (including multiple copies for classroom use), scholarship, or research.” A concept within the fair use doctrine is something called transformative use. This means, broadly, that you can exploit a copyrighted work in a way that was not otherwise intended to do something new and novel like training an AI to make art.
Transformative use is central to any defense of the massive web-scraping activities that mine copyrighted materials for content to train algorithms, and it is the foundation on which most AI companies will likely build their legal arguments.
Fair use is not a mathematical calculation—it is a defense against accusations of infringement, but it often requires the analysis of a judge if challenged. Google used a fair use argument when it was sued for scanning books to create a search tool and displaying snippets of the texts in the results. Transformative use was at the heart of their winning argument, which set a precedent that no doubt many generative AI companies plan to rely on when they inevitably end up in court.
Case law holds that this kind of use—ingesting content or code without permission to create new tools—is a transformative use. And links to works that are hosted elsewhere online, as well as thumbnails of larger images, are also not considered infringement, which is how search tools can function without the platform having to pay the sites that are linked. That said, courts have not explicitly dealt with tools that create new content. Search is one thing; new art may be another.
Fair use and the public domain are legitimate and legal rights allowing creators to use another artist’s works without permission or payment; they are as much a part of copyright law as artists’ rights. They were intended to work together to balance the rights of the creator with the rights of the public. It was an acknowledgement that all art owes something to that which came before it, and also that copyright’s purpose was to advance creativity, not exclusively to drive compensation. This is an important point that is often ignored, perhaps because of our emotional connection to art, both as creators and consumers.
Fostering an ecosystem of creativity and innovation requires a balance of restrictions that protect creators and permissions that reward creators, while also enabling others to make new things; otherwise, we lose the potential of new art and creativity being held back by established creators and copyright holders. As Kirby Ferguson demonstrated so eloquently in his YouTube series “Everything is a Remix,” there isn’t a single creator on Earth who has not been influenced, inspired, incensed, or impressed by other artists.
Since copyright terms have become longer, those extra years of monopoly control have only made more money for the big conglomerates of rights holders. While Disney was deploying its lobbyists to built a bigger fence around Mickey Mouse (one that finally started to come down this year), the rest of the world became less gated and more collaborative, with billions of user-generated posts, pictures, and videos shared online, with the explosion of social media and the rise of collaborative online culture. What few people considered as they shared family photos and creative memes and blog posts and Wikipedia edits was whether the things they made and shared (both freely licensed and not), would become food for the algorithms to be trained and deployed.
Derivatives and the Ownership of Style
Separate from the legality of the machine learning algorithms and models, there is the question of their outputs. While computers can’t yet make copyrighted works, they can make derivative works of existing artists’ content, and those new works are capable of infringing on another artist’s copyrights.
In the class-action litigation filed by the Joseph Saveri Law Firm on behalf of several artists, the plaintiffs argue that the process of diffusion creates derivative works, “because it is generated exclusively from a combination of the conditioning data and the latent images, all of which are copies of copyrighted images. It is, in short, a 21st-century collage tool.” They argue that when the algorithm uses the “de-noising” process to generate images, it’s actually filling in that noise with bits of images lifted from its training data. The plaintiffs contend that since these “new works” are the result of the algorithm’s study and processing of their original art, those works are derivatives.
This is an important distinction in copyright. The U.S. copyright office describes derivative works this way:
A derivative work is a work based on or derived from one or more already existing works. Common derivative works include translations, musical arrangements, motion picture versions of literary material or plays, art reproductions, abridgments, and condensations of preexisting works. Another common type of derivative work is a “new edition” of a preexisting work in which the editorial revisions, annotations, elaborations, or other modifications represent, as a whole, an original work.
So, a translation of a novel from another language is a derivative. A drawing based on a photograph is a derivative, like Shepard Fairey’s famous Obama poster, based on an Associated Press photo. In each instance, the source material is clearly implicated in the new work. This isn’t always the case with the outputs of AI image generators. In addition, they have an impressive ability to create new works “in the style of” the artists they’ve studied. Many of the objections from authors and artists to the works of generative AI come from its ability to impersonate their style or to recreate famous works.
Is it infringement to copy an artist’s style? No, it probably isn’t.
The Legal Artist has a great blog post on this point. But “you can’t copyright style” is an overly simplified statement that merits a much more complicated explanation. To be fair, “style” can be an element of the evidence of infringement, but it can’t be the only element. In Dave Grossman Designs v. Bortin, the court said that:
The law of copyright is clear that only specific expressions of an idea may be copyrighted, that other parties may copy that idea, but that other parties may not copy that specific expression of the idea or portions thereof. For example, Picasso may be entitled to a copyright on his portrait of three women painted in his Cubist motif. Any artist, however, may paint a picture of any subject in the Cubist motif, including a portrait of three women, and not violate Picasso’s copyright so long as the second artist does not substantially copy Picasso’s specific expression of his idea.
Just last month, the Israeli Ministry of Justice issued a new opinion that evaluates whether copyrighted works can be used to train machine learning models. The short answer is yes, but their outputs are another thing altogether. The court makes a notable exception that its opinion applies only to the models, not to their outputs, which is to say, the process by which a new artistic rendering is generated may not infringe on the artists’ works, but the rendending itself still could. This is another important distinction.
One other case worth watching is the Andy Warhol Foundation for the Visual Arts v. Goldsmith case, which the Supreme Court heard last year and will likely result in a ruling soon. The case turns on an argument of transformative use under the fair use doctrine—in this case, Andy Warhol’s interpretations of a photographer’s images of the musician Prince. The decision in the case may lead to some dramatic (or not so dramatic) refinement of what transformative use means in art.
There will not likely be one clear answer on whether any algorithmically generated work is a derivative; rather, it will depend on the facts of each individual case. But similar questions will continue to be asked about the outputs of Stable Diffusion, Midjourney, DALL-E, and whatever comes after them, such as: Does it matter that the tools can reproduce their training images if given the right prompts? Did the algorithm make its images from the originals, or did it just “look at them to learn from them”? The difference will be hugely important and will require a deep dive into the underlying technology. We’re asking a lot of the courts to understand how these algorithms actually work. Honestly, that’s pretty much the whole ball game, and we should expect millions of dollars in legal fees to be spent to determine the answer.
What is clear is that the plaintiffs need the derivative, or collage, framing, because the alternative is that the generator is working “in the style of” the artists, after learning and studying their styles, which would be perfectly legal.
The Story of the Web Is Labor Theft
Even if the outputs of generative AI are not deemed to be derivative, no one disputes that the inputs that informed the machine learning algorithms originally belonged to artists and creators—and they still do, even after the machines have used them. Writing in Wired, Nick Vincent and Hanlin Li called generative AI the “largest theft of labor in history.” They argue that any uses or inspiration derived from a work deserves compensation.
By definition, theft requires you to deprive the property owner of their property. In this case, the original content is exactly where the owner left it. They still have it and can still sell, store, or even destroy it. But what if my use leaves your works intact but harms the value of your goods?
Creators make a case that these machine learning models will harm their livelihoods and devalue their work. For all but the most famous artists, they’re probably right. At the same time, these new tools have found ways to create unanticipated value by combining the works of millions of creators—not just art, but every post, tweet, comment, pic, and video. That new value might deserve to be shared with the creators whose work inspired it, but it’s not clear that their individual contributions have value beyond their role as part of the greater sum of creation.
So, is it labor theft if you find an unanticipated and unrealized value in something by combining it with other works and processing it? Conversely, is it fair to generate new wealth from the works of poor artists and then use your creation not only to generate profit but also to replace the artists with robots?
The story of the web is of companies benefiting from the unpaid labor of users, who often don’t know their content has value. And the truth about data is that most of it has little value until it’s combined with other data, processed, and analyzed. There’s an implicit trade-off of user labor and free web services in most of the web we use every day.
Furthermore, while a reading of fair use doctrine does consider economic impacts, it focuses narrowly on whether the infringing use denies the original creator the market for their specific works. It doesn’t care if the new works diminish the market for artist-created works overall. It also doesn’t care if the new works create unanticipated or previously unimagined value that is not shared with the original creators.
Many of these activities will likely be interpreted to be completely legal and permissible under current laws and precedents—part of the trade-off of copyright and public exceptions to “promote the Progress of Science and useful Arts,” as described in Article I of the U.S. Constitution.
What is less clear is what shape this new synthetic economy will look like, and who, if anyone, will own the outputs of generative AI. This is where we should focus our attention, as it will define the future of creativity.
Can Androids Copyright Electric Sheep?
The potential for generative AI-based technology is enormous. Investors are clamoring to find ways to build impenetrable moats around their product ideas. In a recent note to investors, venture capital firm Andreessen Horowitz noted that: “The potential size of this market is hard to grasp—somewhere between all software and all human endeavors—so we expect many, many players and healthy competition at all levels of the stack.”
There’s little doubt these new tools will reshape economies, but who will benefit and who will be left out? One key area of concern is copyright, since it’s how creativity is controlled and monetized. Deciding who can create it and who will retain it will define the market and its winners and losers.
Today’s laws allow only humans to create a new copyright. So far, the U.S. Patent and Trademark Office has trod lightly to avoid declaring any wholly computer-generated work eligible for copyright. In the coming years, there will be enormous pressure on lawmakers to allow computer-generated works to be eligible for copyright. If there is a line in the sand to be drawn, it’s between humans and computers. Rather than allow computer art to devalue human works, one solution might be to elevate human art and decide that AI art should never have equal value.
Legal frameworks worldwide rely on laws written sometimes hundreds of years ago before computers even existed. Beyond those laws, there are moral and ethical questions that remain unanswered. What is the good we hope to create, and what are the harms that might result? What kind of competitive market do we want, and who, or what, will we be competing with?
Throughout history, artists have studied the works of masters, and even copied them, to hone their craft. Artists being inspired by or even copying the styles of others is not just normal, it’s how art evolves. Quoting musicians is an homage, not theft. A poem in the style of Jorge Luis Borges evokes a smile, not ire. Producing even a passable copy of a great work of art takes time and talent.
So many things in society have structures that are built upon the friction of work. Copying a book used to require a group of monks. Later, you had to own a printing press. And while every artist can remix, it is slow and laborious. Today’s rules were written and negotiated in a time that required sweat and time to produce labor. Current laws weren’t written to contemplate a world in which “the artist” was a wall of servers that trained on 5 billion works and could produce new ones without effort in seconds, something that inherently has a destabilizing effect on the market that no single artist studying and working could ever have.
New works created by AI will always be a distillation of everything they have seen, but they are not (yet) capable of new thought or creativity or originality. They are, by the definition of their code and composition, always, at best, the analytical reduction of the masters (and miscreants) whose work they studied. The AI downcycles art and content. It has no original mind.
That absence of a capacity for true originality, coupled with the public’s enormous contribution to the training of these tools, makes a compelling case for an accommodation that benefits artists and the public. With that in mind, I’ll propose the following: New works created with generative AI should not be eligible for copyright. Yes, that’s a complicated rule to enforce, but the alternative is equally untenable. This is the collective bargain we should strike with the billions of writers, artists, posters, tweeters, commenters, and photographers whose work unwittingly and unwillingly informs the algorithms: No computer will ever make new copyright, especially if it does so from the work of humans.
That doesn’t mean that those works would have no value, or that they could not be sold. But it does mean that new AI-generated works won’t ever get the 100-plus years of monopoly protection that a human now enjoys when creating an original work. It means that everything AI makes would immediately enter the public domain and be available to every other creator to use, as they wish, in perpetuity and without permission.
If it sounds unfair, I’ll remind you that it’s exactly what the creators of the machine learning models did when they scraped the complete works of humanity to train their generative AI algorithms. They paid nothing for their raw materials.
I’ll add a warning: If we allow computers to make new copyrights, we should expect an AI-generated version of every melody and chord change possible to be authored and copyrighted, followed immediately by automated lawsuits to defend them. Every new hit will be followed immediately by a spate of lawsuits showing prior art. It could be the end of human creativity as we know it, and the rise of the AI copyright troll.
National Security Implications
Existing industries, leaders, and legislators are likely ill equipped to decide how to respond to the incredible pressure that will be put on them in the coming months and years. This is about much more than art and ideas.
The risks that governments must grapple with will extend beyond the commercial and will no doubt have international implications as well. Today’s debates around AI tools like ChatGPT are so focused on college essays and impersonating artists, they’ve yet to consider how the successful deployment of generative AI tools will force every country into a new arms race: creating an opportunity for advantage for nations that develop and exploit (and likely restrict or sell access to) AI, but also a looming national security risk for those that do not.
Beyond the veil of U.S. copyright, there is a complex web of international treaties and laws that govern how copyright is treated around the world. Sovereign states rely on those treaties—and negotiate new trade frameworks within them—to protect and promote their economic interests worldwide. These laws govern everything from feature films to pharmaceuticals and are the subject of constant negotiation within other multinational agreements. There are varying terms, rights, exceptions, and obligations, all existing in a complex reciprocal framework that ensures that an artist in Australia, for example, is able to assert their rights if they are infringed upon in Canada. They have their own arbitration and mediation center at the World Intellectual Property Organization to hear complaints. If copyright laws were bent to allow algorithms to become authors, it would upend one of the foundational principles of global intellectual property and just might unleash a torrent of new issues so overwhelming that it could spell the end of international treaties like the Berne Convention, already creaking under the weight of the internet.
The potential for these tools to reshuffle the deck of have and have not nations remains to be seen, but it will no doubt create new alliances and new rivalries (while also deepening existing ones). Perhaps more urgent than the end of global intellectual property treaties is the very real risk posed to nations that may soon be subject to a deluge of convincing fake content, limitless pools of falsified recordings (Microsoft claims it can make convincing imitations from just a few seconds of audio), and video able to impersonate public officials or fictionalize events from untraceable state-sponsored and freelance bad actors, making attribution difficult, if not impossible. The potential for high-quality, low-cost disinformation campaigns was already high; generative AI makes it a near certainty.
It’s true that copyright and intellectual property are just one piece of the global economy, but there’s enormous risk in ignoring what might happen if that one vital element were destabilized at a global scale. We can choose to ignore the difference between human originality and AI-powered distillation of creativity, but then we also choose to accept the harm it will inflict on creators, and the loss to society of their absent creativity—we’re choosing to trade technological innovation over a subset of human ingenuity and originality. But we will also have to accept the dramatic consequences of undermining the international structures that support the creation of intellectual property. Perhaps the right trade-off is to accept the innovation but acknowledge that its outputs do not, and should not, earn the same protections as the original creations of humanity.
Conclusion
At this point, there’s no going back. If we didn’t want our art and images to train AI, the time to act was 10 years ago, before the developers of generative AI systems started scraping and scanning artists’ works to train the algorithms.
The idea of artist compensation for the use of their works is an unfortunate fallacy. Individual human endeavor does not have an individual value once it is dissolved into the algorithm; the value is, at best, collective and would be, in the database of 5 billion works, miniscule and impossible to assign to any one human. The explosion of online content has also driven the reduction in the value of all human art, where anyone can now access the vast majority of recorded music for just $9.99 a month. Even if there is money to be had, nothing will ever replace the revenue that will undoubtedly be lost by artists when mainstream creative tools replace their labor.
No doubt someone will evoke Elinor Ostrom’s work on the tragedy of the commons concept, which brings us back to the notion of rival and nonrival goods. Ostrom won the Nobel Prize for her book “Governing the Commons,” an analysis of governance of common resources that are exploited and ultimately mismanaged into extinction. But the metaphor here is more complicated because, in our story, the “commons” remains unharmed and available to everyone, but its exploitation has potentially eliminated the need for the “farmers” altogether.
One unanswered question is where do we want human art to live in our society? We need to figure out how to resource human art in ways that acknowledge it as a public good and a humane endeavor worth preserving and protecting. Otherwise, art will have to compete economically with tireless machines capable of endless impersonation. If history has taught us anything, it’s that commerce is lousy at deciding what constitutes great art.