AI Liability for Intellectual Property Harms
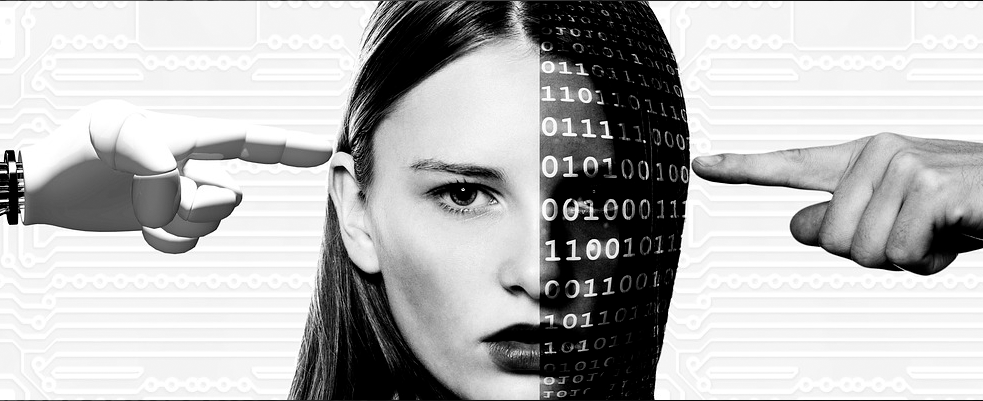
Published by The Lawfare Institute
in Cooperation With
Editor’s note: This essay is part of a series on liability in the AI ecosystem, from Lawfare and the Georgetown Institute for Technology Law and Policy.
By now, you’ve probably seen the images of President Trump being arrested or Pope Francis wearing a puffer jacket. All of those images were AI generated, which is to say, they’re fake. They’re hyperrealistic images of events that never occurred. But they were generated by AI models that were trained on real images of the Pope and real images of President Trump. Do the owners of the original images have a claim against the model developer, or the user who prompted the model? This article will disentangle the thorny intellectual property issues around generative AI, beginning with copyright infringement and then moving on to trademark law and the right of publicity.
Copyright Infringement
“Generative AI” is a broad umbrella term that refers to any computational system that “learns” how to generate output in a specific modality (text, image, video) by identifying patterns and structures within its training data. ChatGPT, for example, is a large language model that has been trained on a vast corpus of content, written and otherwise. Midjourney is a text-to-image generator that has been trained on large image datasets. Suno generates music after being trained on millions of compositions and sound recordings. Each of these systems is able to produce high-quality synthetic media that look or sound like they were produced by a human. Naturally, many of the authors, artists, and musicians who own the underlying training data are really mad. AI models have been trained on their works without their permission and without compensation. As a result of this training, these models are able to generate outputs that compete with human-authored works and, in some cases, substitute for them.
So, unsurprisingly, the owners of copyrighted training data are taking AI vendors to court. At least 28 lawsuits have now been filed against OpenAI, Stability AI, Anthropic, and others. Most plaintiffs make at least one of the following arguments: first, that training generative models on copyrighted works without the owner’s permission is copyright infringement; second, that the outputs themselves are infringing; and finally, that the removal of copyright management information from copyrighted training data violates the Digital Millennium Copyright Act. Let’s examine each of these arguments in turn.
Unlicensed training is infringing.
Copyright law protects the original expression reflected by your creative choices. It grants authors exclusive rights to reproduce and distribute that expression to ensure that creative works are produced at optimal levels. However, it does not protect the facts or ideas contained within that expression. For example, if you take a photo of a dog, you can’t stop other people from taking a photo of the same dog (the idea of photographing the dog is unprotectable), but you can stop someone from making a complete or partial copy of the particular way in which you photographed the dog (for example, the camera angle, lighting, composition, and background).
Why might it be a violation of the copyright owner’s exclusive rights to train a computational system on the internal structure of an image or a sentence? The simplest reason is that in order to understand the internal structure of a creative work, the system first has to make a copy of it. The Copyright Act says that only the author of a copyrighted work can make copies of it, and anyone else has to get the author’s permission. This restriction was designed to protect the author’s market from the sale of unauthorized copies. But it seems strange to extend that restriction to incidental copies made in the belly of the machine that never see the light of day. These copies don’t communicate the author’s creative expression to a new audience, and so prohibiting their creation does not serve the original purpose of copyright law.
The theory of “non-expressive” fair use says that a “copy-reliant” technology (which makes automatic and indiscriminate copies of copyrighted works in the performance of its functions) does not infringe those works if the copies are used for a non-expressive purpose. For example, although search engines display fragments of web pages as part of their search results, this is designed to facilitate effective web search (by directing users to relevant websites), not to compete with the original creator by communicating the content of those web pages to a new audience. Similarly, the display of thumbnails in Google Image Search, or Snippet View in Google Books, is designed to help users identify relevant image and book resources, rather than to substitute for their original expression.
The theory of non-expressive fair use is a little harder to apply in the context of generative AI. Generative models rely on copies of copyrighted training data in order to “learn” unprotectable facts and other statistics about those works, for example, the relationship between pixels. This extraction of uncopyrightable metadata is considered to be a non-expressive use unless the model uses this information to generate output that reproduces the underlying training data, in which case the model is communicating the copyrighted training data to a new audience.
Copyright scholars are divided over whether AI training is fair use. Some scholars are adamant that unlicensed training is non-expressive because models rarely generate output that is substantially similar to (or a perfect copy of) their underlying training data. And model designers can take active steps to reduce the risk of “memorization” (where a model generates verbatim copies of training data). However, other scholars emphasize that the purpose of training is to generate outputs that imitate, and therefore compete with, the expressive features of copyrighted training data. This distinguishes generative AI from Google Books, which was designed to help users find existing works, rather than to generate competing content.
Courts evaluate whether the copy-reliant technology sufficiently “transforms” the original work. A searchable digital catalog of books is “transformative” in the sense that it adds something new, with a further purpose or different character, and does not substitute for the original work. On the one hand, large language models like ChatGPT are not dissimilar from search engines; individuals frequently use them for information-gathering purposes. This creates a strong argument in favor of treating training datasets as informal archives that mediate access to knowledge, especially where AI outputs refer users to their original source content. On the other hand, image-generating models like Midjourney can reproduce copyrighted training data with only minor alterations. It’s difficult to argue that these near-verbatim copies are transformative when they serve the same purpose as the underlying works, for example, as decorative art, illustrations in a book, or marketing media. In its most recent application of the transformativeness standard, the Supreme Court held that Andy Warhol’s use of Lynn Goldsmith’s photograph was not transformative, at least in part because it served the same purpose as the original photograph (to depict Prince in a magazine story about Prince).
Also relevant to the courts’ analysis of whether unlicensed training is fair use is the issue of market harm. If owners of copyrighted training data can show that unlicensed training is affecting the market for their works, such evidence of market harm will weigh against a finding of fair use. In evaluating whether copyright owners have suffered market harm, courts generally take industry practices into account. The recent spate of licensing agreements between copyright owners and AI vendors suggests that there is a market for training licenses, and this may help copyright owners argue that they should have been paid for the use of their works as training data (and therefore that unlicensed training is not fair use).
Separately, some scholars have argued that generative models should be allowed to “learn” how to write, and paint, and sing from copyrighted works, in the same way that humans develop these skills from reading books, viewing paintings, and listening to music. Aspiring artists regularly mimic famous works to practice or pay homage. But, while it’s true that I can learn how to sing by listening to Taylor Swift, that doesn’t entitle me to torrent her latest album, rather than accessing it through lawful means. Humans generally have to pay to access copyrighted works, even if they’re using them for educational purposes. Although the copyright statute authorizes the use of copyrighted works for teaching, scholarship, and research, a use that is fair at the level of the individual may no longer be fair when carried out at scale. Scientists who photocopied journal articles for research purposes, for example, were held liable for copyright infringement. Universities that copied too many textbook chapters for their students were successfully sued by academic publishers.
If AI models were able to “learn” from copyrighted works without paying for them first, this would extend a privilege to machines that is not currently afforded to humans. Eight years ago, long before the introduction of generative AI, James Grimmelmann warned about the denigrating effects of a “two-tracked copyright law” in which human readers were subject to strict scrutiny while robotic readers were given a free pass. If copyright law made it cheaper “to train machines than to educate human authors to perform similar tasks,” this would dramatically accelerate the displacement of human labor. At this critical juncture, policymakers have two choices: Either give AI models a special “fair learning” exception in light of the upfront investment required to sustain AI innovation, or use this opportunity as a bargaining chip, and refuse to extend fair use to shield AI training unless and until a similar exemption is made for human learning.
AI-generated outputs are infringing.
The second argument frequently made by copyright owners is that AI-generated outputs are themselves infringing derivative works. This argument is less controversial than the first. Copyright scholars generally agree that AI-generated outputs are infringing if they are “substantially similar” to copyrighted training data. Courts have developed a few different tests to measure the similarity between works, but they generally ask whether an “ ordinary observer” would regard the secondary work as “substantially similar” to protected expression within the original work.
Copyright protects a very broad range of subject matter, from sounds to software, so legal tests developed to evaluate the similarity between literary texts often struggle to evaluate the similarities between nontextual works. The difficulty of this task is compounded by the need to differentiate between copyrightable and uncopyrightable parts of a work, that is, between original creative expression (protectable, such as the specific photo of a dog) and ideas, concepts, or methods (unprotectable, such as the idea of a photo of a dog). This means that a court evaluating whether an AI-generated image is “substantially similar” to a copyrighted training image will first need to identify which parts of the image are actually protected by copyright law (as opposed to uncopyrightable ideas) and then compare this expression to the underlying expression in the copyrighted training image.
It is difficult to cleave the unprotectable elements of a visual work from its protectable expression, while also identifying copyrightable combinations of otherwise unprotectable elements. For example, what is the unprotectable “idea” conveyed by a work of art, when each observer might interpret it differently, depending on how the idea was expressed? Courts often regress to cataloging descriptive features, such as shapes and colors, or deferring to an artist’s description of their process, which can result in the protection of artistic techniques, rather than original expression. This is an undesirable result because those techniques should be available for all artists to use, and copyright explicitly denies protection to processes, systems, and methods of operation.
Already courts are struggling to apply their own tests to nontextual works in ongoing AI litigation. Visual artists have struggled to show substantial similarity between AI-generated outputs and copyrighted training data, whereas authors have more persuasively shown that AI models generate near-verbatim copies of textual works. The New York Times’s complaint against OpenAI contains several compelling examples of memorized text.
Another element of a creative work that is generally uncopyrightable is an artist’s distinctive style. The uncopyrightability of style has featured heavily in public debates about the copyright status of AI outputs. Generally speaking, an artist’s signature aesthetic is not protected by copyright law, for a few reasons. First, allowing an artist to monopolize a particular mode of expression would be inconsistent with the idea-expression dichotomy (which denies copyright protection to unprotectable ideas). As courts have explained:
Picasso may be entitled to a copyright on his portrait of three women painted in his Cubist motif. Any artist, however, may paint a picture of any subject in the Cubist motif, including a portrait of three women, and not violate Picasso’s copyright so long as the second artist does not substantially copy Picasso’s specific expression of his idea.
Second, an artist’s distinctive style is generally discernible only across a portfolio of works. Providing protection to creative elements across multiple works would be inconsistent with copyright’s protection for singular works.
Although style is generally uncopyrightable, the degree to which AI outputs mimic distinctive styles is relevant to a legal analysis of whether the AI output is substantially similar to the original. Additionally, although copyright doctrine affirms the unprotectability of style, there are several cases where courts have allowed plaintiffs to protect distinctive stylistic elements of their work.
Stripping copyright metadata violates the DMCA.
Although unlicensed training and memorized outputs have dominated news headlines, there is a third, lesser-known claim frequently leveled against AI vendors that, if successful, is equally capable of bringing these firms to their knees.
Section 1202 of the Digital Millennium Copyright Act (DMCA) prohibits the modification or removal of copyright management information (CMI) such as a work’s title, its author, and the terms and conditions of its use. This prohibition was designed to thwart would-be infringers from concealing their infringement, for example, by cropping metadata from a copyrighted photograph. In the preparation of training datasets, CMI is often removed from copyrighted works. As a result, many plaintiffs allege that AI vendors violated § 1202 both in the preparation of their datasets and in the “distribution” of CMI-free works via memorized outputs.
These allegations are significant for a few reasons. First, there’s no registration requirement to sue under § 1202, which opens the door to content owners who may not have registered their works and therefore cannot sue for infringement. Second, violations of § 1202 can give rise to significant statutory damages, ranging from $2,500 to $25,000 per violation. Given the staggering number of works used as training data, statutory damages for CMI removal could be crippling for AI firms. The lawsuit against GitHub estimates that statutory damages for violations of § 1202 will exceed $9 million. (Software programmers who contributed their code to GitHub, a software development platform, are suing the creators of Copilot, a code-completion AI model, that was trained on copyrighted code that was published on GitHub under open-source licenses.)
Despite this, proving a violation of § 1202 is not without difficulty. It has two knowledge requirements: first, that the AI vendor knew (or ought to have known) that CMI had been altered or removed, and second, that they knew (or ought to have known) that this would induce, enable, or conceal infringement. In other words, stripping CMI is not enough; plaintiffs must show a nexus to infringement. If AI vendors can successfully argue that removal or alteration of CMI was the unintended result of an automated process, and that they reasonably believed that unlicensed training was fair use and that AI outputs were noninfringing, it may be hard for plaintiffs to satisfy these intent elements. In addition, plaintiffs have to catalog the specific CMI that was removed from their works—an onerous and time-consuming process. Courts have already dismissed claims where CMI removal was under-specified. This creates considerable uncertainty around the success of DMCA claims.
The Distribution of Liability
It is often financially prudent for a copyright owner to sue a deep-pocketed technological intermediary (whose actions facilitated infringement) rather than the individual who actually “pressed the button.” For example, the entertainment companies that were concerned about people recording publicly broadcast movies and TV shows at home sued Sony, the manufacturer and distributor of VCRs, rather than the users themselves.
Copyright law distinguishes between direct and indirect infringers. Direct infringers are most causally proximate to the infringing copy, for example, the person recording several seasons of their favorite TV show on VHS. Direct liability does not require proof of intent to infringe (someone can infringe subconsciously), but it does require intent to make the relevant copy (as opposed to accidentally pressing the button on the copy machine). This is known as volition. Indirect infringers include any other party that contributes to the infringement, for example, a web platform that facilitates the online exchange of pirated music files.
Indirect copyright liability is governed by two different doctrines: vicarious liability (where you had the power to prevent the infringement but chose not to) and contributory liability (where you knowingly and materially contributed to the infringement). The New York Times complaint alleges that Microsoft is contributorily liable for OpenAI’s infringement because it provided the computing infrastructure and resources to build and store training datasets, and to host, operate, and commercialize generative models. It also alleges that Microsoft is vicariously liable because it controls and directs the supercomputing platform used to store, process, and reproduce training datasets, and it profited from the incorporation of generative models into its own product offerings, including Bing Chat.
If an author (let’s call her Rachel) discovers that she can prompt ChatGPT to generate verbatim chunks of her copyrighted books, how would a court allocate responsibility for this outcome between the various parties in the generative AI supply chain? Let’s start at the beginning.
First, the creator of the training dataset might be directly liable for the unauthorized copies of Rachel’s books that appear within the dataset. This party chose which works to include in the dataset and therefore assumed the risk that some of those works might be copyright-protected. (If the dataset is used to train a non-generative model, the dataset creator might be able to argue that the creation of the dataset is transformative.) If the dataset creator makes the dataset available to model trainers, they could also be directly liable for violating Rachel’s exclusive right to distribute her work. Additionally, a dataset creator could be indirectly liable for infringing model outputs if the party contributes data to a model knowing that it will be used for infringement, such as one known or designed to produce memorized outputs (where the model generates verbatim copies of its training data). This might constitute a material contribution to the infringement.
Second, the model trainer might be directly liable for the model itself as an unauthorized copy or derivative work of its training data. Although the training data is not perceptible to humans while it is encoded within the model, the model constitutes a “copy” of every work it is “ capable of generating” because the statute defines a copy as a “material object ... in which a work is fixed ... and from which the work can be perceived ... either directly or with the aid of a machine or device.” Making the model available for download by third parties might also violate the distribution right. The model trainer could also be indirectly liable for the model’s infringing outputs because the trainer could have taken steps to reduce the likelihood of model memorization (for example, prompt modifiers or output filters).
Third, parties that fine-tune or align models could be indirectly liable for the model’s infringing outputs for failing to take steps to reduce the likelihood of memorization. Like model trainers, these parties have some control over model outputs; therefore, they can take steps to nudge models away from infringement.
Fourth, a party could deploy an application that incorporates a generative model without adjusting any of its parameters or establishing guardrails to avoid copyright violations. This party would not be responsible for the underlying dataset, but they could be indirectly liable for infringing model outputs. However, if they advertise or encourage infringing uses of the model, they could be indirectly or directly liable for those generations.
It may be difficult for model deployers to escape liability by arguing that even if their models produce some infringing output, they are also capable of substantial noninfringing uses. Manufacturers of physical devices (like VCRs) can persuasively argue that bans on the distribution of those devices will preclude both infringing and noninfringing uses. But model deployers retain ongoing control over their models, which means they can take steps to reduce the risk of infringement while also preserving use of the model for noninfringing uses. They can help the model “forget” certain content, or automatically block prompts that ask for copyrighted works. Banning model versions that fail to take these precautions would not chill noninfringing uses of AI the way banning the manufacture and distribution of VCRs would chill noninfringing uses of these devices.
Finally, a user who prompts a model to generate copies of its training data might be directly or indirectly liable for those infringing generations, depending on the circumstances. A user who enters an “innocent” prompt (for example, “create an image of a male superhero”) may not be liable where the model returns infringing content (for example, a copyrighted image of Captain America). Although some models allow users to upload their own content, most commercially available models come preloaded with “embedded representations of copyrighted works.” This means that infringing output is significantly influenced by the pre-prompt characteristics of the model, rather than the content of the user’s prompt. Having said that, a user who “tricks” a model into bypassing its guardrails and generating copyrighted content might be directly liable for those infringing generations.
As mentioned, copyright owners may prefer to hold AI vendors responsible for infringing AI outputs because they are identifiable, deep-pocketed, and sophisticated legal actors, unlike users, who tend to be anonymous and highly distributed. However, lawsuits against individual users are not beyond the realm of possibility, as the history of file-sharing litigation demonstrates.
The foregoing discussion strongly suggests that risk-averse parties within the generative AI supply chain should license copyrighted training data, avoid model distribution to downstream infringers, and reduce the risk of model memorization. However, there are also reasons to be wary of this approach. AI vendors that limit the capabilities of their models (to avoid copyright infringement liability) also risk upsetting the delicate balance between author’s economic interests and users’ creative freedoms. If I ask ChatGPT to reimagine Captain America as a queer Black man from Brooklyn, the model refuses, citing “content policy restrictions.” If I ask the model to describe its restrictions on image generation, it says that it cannot depict any copyrighted character in a manner that is “contrary” to its established image. Queer-ing Captain America, however, is paradigmatic fair use. This means that AI vendors, by adopting these restrictions, are preventing users from engaging in lawful uses of copyrighted works. This undermines the capacity of generative AI to promote a democratic and participatory culture by allowing users (without any artistic training or skills) to remix cultural works.
Trademark Infringement
Another type of intellectual property that is affected by generative AI is trademark law. Whereas copyright law is designed to correct the underproduction of public goods (non-rivalrous and non-excludable creative works) by providing authors with time-limited monopolies, trademark law is designed to prevent consumer confusion by protecting brands and logos that connect companies to specific goods and services.
Trademark law intersects with generative AI in a few different ways.
First, some AI vendors have been accused of trademark infringement (unauthorized use of a mark in a way that would confuse consumers) and dilution (blurring or tarnishment of a famous mark) because their models reproduce trademarks attached to their training data. For example, Getty Images’s complaint against Stability AI alleges that some of the model’s outputs contain a modified version of the Getty Images watermark, which creates a false association with Getty Images in the minds of consumers, and dilutes the quality of its marks, especially where they appear on offensive or low-quality AI outputs. Similarly, the New York Times’s complaint against OpenAI alleges that the unauthorized use of the Times’s trademarks in low-quality and inaccurate ChatGPT outputs dilutes the quality of those marks.
For the plaintiffs to succeed in their claims, they must show (a) use, (b) in commerce, and (c) a likelihood of confusion. It may be difficult to show that the appearance of marks in AI-generated output represents a use “in commerce,” that is, in connection with the sale or advertising of goods or services. Additionally, it may be difficult to demonstrate a likelihood of confusion on the part of consumers as to the affiliation, connection, or association of the defendant with the model outputs, especially where the AI-generated marks are distorted.
Additionally, visual artists have alleged that AI-generated outputs infringe their trade dress when they replicate the distinctive aesthetic of their works. “Trade dress” refers to the distinctive look and feel of a product or service, for example, the distinctive packaging of a product or décor of a store. It serves the same source-identifying function as a trademark. The plaintiffs in Andersen v. Stability AI (visual artists) claim that Midjourney controls, and profits from, trade dress imitations by encouraging users to generate outputs that reproduce the recurring visual elements and artistic techniques that characterize their work. Trade dress may offer visual artists some protection for their distinctive style, which is generally uncopyrightable.
Right of Publicity
Generally speaking, the right of publicity gives an individual control over the use of their name, voice, signature, photograph, or likeness in commerce. If a famous actress declines to lend her voice to an AI-powered chatbot assistant, the AI firm can’t just hire an actor to imitate her voice. (Ford tried this with Bette Midler in the 1980s, and it didn’t work out so well for them.) Similarly, if someone trains an AI model to simulate the vocals of two famous artists, those artists can sue for violation of their publicity rights.
While requirements vary by jurisdiction, a plaintiff generally has to prove at least two elements in order to establish a right of publicity violation: first, that the defendant has used the plaintiff’s persona in an identifiable way without the plaintiff’s permission; and second, that the defendant’s use is likely to cause damage to the commercial value of that persona.
Publicity rights are governed by a patchwork of state laws with idiosyncratic requirements, which creates confusion and information costs for litigants. Pursuing bad actors through multiple state court systems is tedious and expensive. There are increasing calls for a federal right of publicity, and bills to this effect have been proposed. Although the right of publicity emerged from the right to privacy (and a desire to protect individuals from dignitarian harm), it has evolved into a property-like interest that encompasses almost any unauthorized use of an individual’s name or likeness. It is a trademark-adjacent protection because it prevents false and misleading representations of endorsement.
Would President Trump or Pope Francis be able to sue the creators of their deepfake personas for violating their publicity rights? Probably not. First, those deepfakes were created for noncommercial purposes, and many state publicity laws have a commerciality requirement. Second, the deepfakes would likely be protected by the First Amendment as a transformative expressive use. Given the risk that individuals may use publicity rights to suppress any unflattering portrayals of themselves, at the expense of valuable expression, courts try to resolve the tension between free speech and publicity rights by asking whether the secondary work “adds significant creative elements” that take it beyond “a mere celebrity likeness or imitation.” Since the test for transformative use of publicity rights was imported from copyright law, there is a chance that it will be affected by the Supreme Court’s narrowing of transformativeness in Warhol.
Could the appropriation of an artist’s distinctive style by an AI model constitute a right of publicity violation? Maybe. To avoid preemption by federal copyright law, a state right of publicity claim must either protect different subject matter or include an extra element beyond those required for copyright infringement to make the claim qualitatively distinct from copyright. This is not an easy hurdle to clear, as previous cases have shown.
The AI-generated song “Heart on My Sleeve” showcases the complex intersection of intellectual property rights implicated by generative AI. The creator (a songwriter operating under the pseudonym Ghostwriter977) explained that he imitated the vocals of Drake and The Weeknd (using so-called AI vocal filters) in order to highlight the undervalued contributions of songwriters. The song’s resonance with fans, despite no involvement by recording artists, demonstrates the value that songwriters bring to creative projects.
To create “Heart on My Sleeve,” Ghostwriter977 presumably trained an AI model on copyrighted songs by Drake and The Weeknd in order to generate sound-alike vocals. The creation of those training copies may attract copyright liability if courts ultimately find that unlicensed training is not fair use. The AI-generated output may also attract liability if Drake and The Weeknd can show that it’s substantially similar to their works. However, this may be hard to prove. Ghostwriter977 wrote his own melody and lyrics, and copyright protection for sound recordings does not extend to deliberate imitations. The musicians’ strongest claim is that the simulation of their voices represents a violation of their publicity rights. However, there is no DMCA takedown remedy for publicity rights, and filing a state claim is a slower process. In order to have the song removed, Universal Music Group reportedly had to reference the unauthorized sampling of a producer tag.
As generative AI raises public awareness of publicity rights, there are signs that AI vendors may be more cautious about licensing the use of celebrity voices for their AI products in the future.
Looking Forward
In their efforts to decelerate the pace of AI innovation, and remedy the labor displacement associated with generative AI, artists, authors, and other rights holders have asked the courts to find both AI inputs and outputs to be copyright infringing. As this article has shown, however, the copyright status of unlicensed training and AI-generated outputs remains unclear. If the Google Books litigation is anything to go by, it could be a decade before courts provide definitive guidance on these issues. In the meantime, risk-averse parties within the generative AI supply chain will continue to license copyrighted training data and limit the capabilities of their models in order to reduce their liability exposure. Trademark and right of publicity claims will also remain relevant, but the largest remedies are likely to be found in statutory damages for copyright infringement.