The Pros and Cons of California's Proposed SB-1047 AI Safety Law
Creators of frontier AI models should be strictly liable for the harms those models cause.
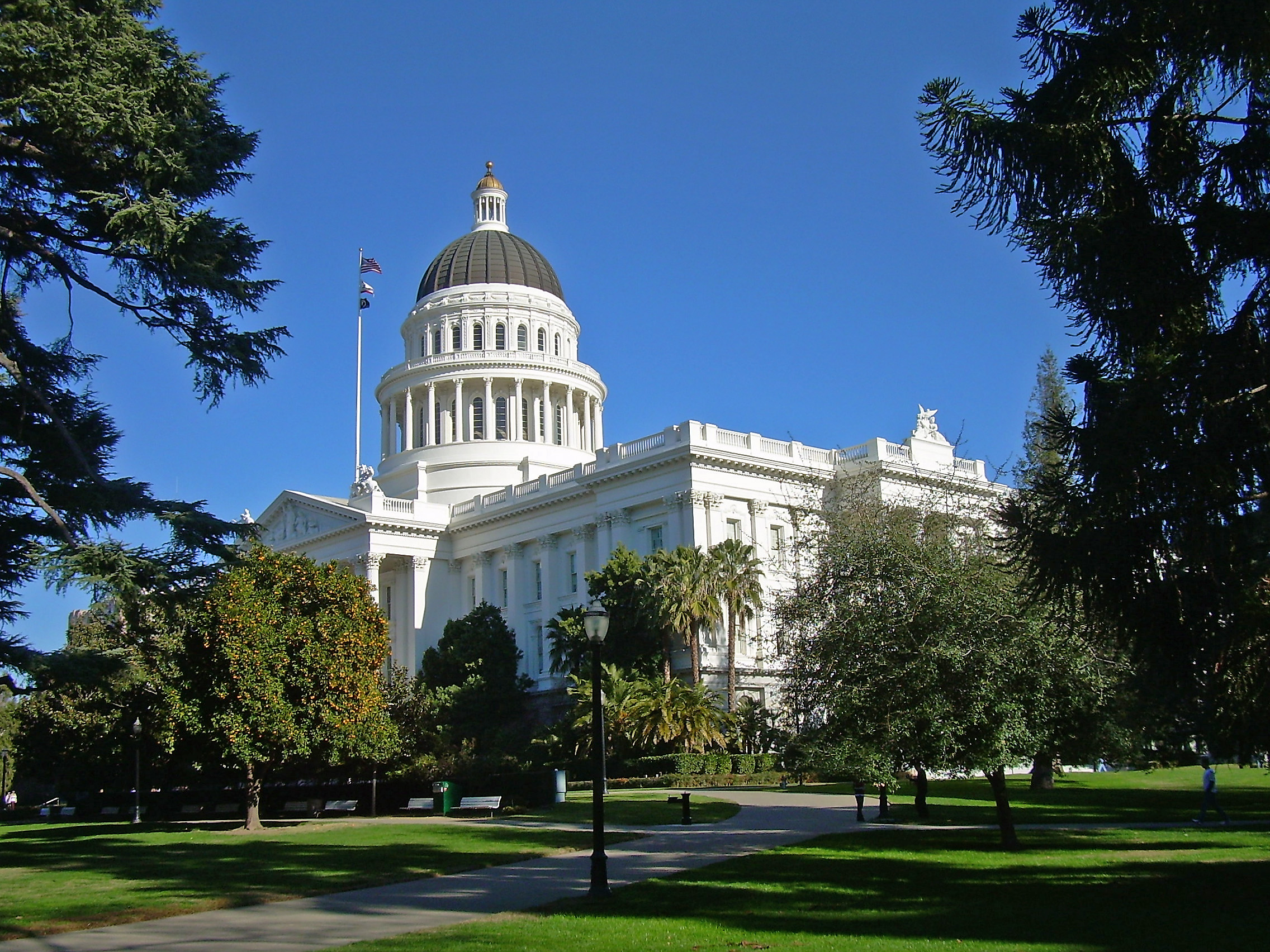
Published by The Lawfare Institute
in Cooperation With
California SB-1047, the Safe and Secure Innovation for Frontier Artificial Intelligence Models Act, was introduced by Sen. Scott Weiner in February and passed through the Judiciary Committee and the Committee on Governmental Organization in April. This legislation represents an important first step toward protecting humanity from the risks of advanced artificial intelligence (AI) systems. Weiner and his co-sponsors deserve praise for taking on this critical and neglected issue. Nonetheless, the bill falls short of its promise to protect public safety from the risks posed by frontier AI systems in a few key respects.
These shortcomings all relate to one central fact: AI safety remains unsolved as a technical problem. The best way to encourage frontier AI developers to continually push forward the safety frontier is to make them bear the risk that systems will cause harm. SB-1047 holds AI developers liable only when they fail to adopt specific precautionary measures laid out in the statute. Policymakers should not be confident that these precautionary measures will provide adequate protection for public safety.
SB-1047 would create a new regulatory framework for frontier AI systems, defined as models trained with more than 1026 floating-point operations (FLOP) of compute. This is the same compute threshold used in the Biden administration’s Executive Order on the Safe, Secure, and Trustworthy Development and Use of Artificial Intelligence. No AI system has yet been trained with 1026 FLOP, but it is expected that the next generation of frontier models will exceed this figure.
Unlike the federal executive order, however, the California bill also covers systems trained with enough compute that they could reasonably be expected to perform as well as a model trained with 1026 FLOP in 2024. This provision is an important measure to account for potential improvements in algorithmic efficiency that would allow potentially quite powerful and dangerous models to be trained with less than 1026 FLOP.
Under the bill, covered systems would have two compliance pathways. First, the developer can certify that the system qualifies for a “limited duty exemption,” which applies to systems for which the “developer can reasonably exclude the possibility that” it has or might plausibly develop certain specified hazardous capabilities. These hazardous capabilities include substantially facilitating the creation or use of weapons of mass destruction, cyberattacks or other criminal activity resulting in at least $500 million in damage, or other comparably severe threats to public safety.
It makes sense to focus regulatory attention on the most potentially dangerous systems. My only concern with this compliance pathway is that if developers rely, in good faith, on a reasonable, but erroneous, conclusion that their system qualifies for this exemption, they will not be deemed in violation of this statute. This puts a lot of weight on an assessment of the reasonableness of AI labs’ conclusion that their models lack dangerous capabilities. Public safety would be better served by a regulatory regime that holds AI developers liable for the harms caused by their systems, even in cases where it cannot be proved that specific dangerous capabilities were foreseeable. This would shift the onus to the AI labs, which best understand the risks posed by their systems, to make sure they are safe. I have similar concerns about the second compliance pathway.
For models that do not qualify for the limited duty exemption, developers must implement various safeguards. These safeguards include “appropriate” cybersecurity protections to prevent unauthorized access to or modification of the system, inclusion of a “kill switch,” implementation of voluntary standards promulgated by the National Institute of Standards and Technology (NIST) and industry best practices, implementation of a written safety and security protocol that “provides reasonable assurance” that it will prevent “critical harms from the exercise of a hazardous capability in a covered model,” and refraining from training models that pose an unreasonable risk of being used to cause critical harm.
These safeguards are valuable. Their implementation would substantially mitigate the risks posed by frontier AI systems. They will also have some costs. In particular, open-source AI development may be substantially inhibited by a concern that regulators will deem the practice “inappropriate.” But the risks posed by open sourcing models with potentially dangerous capabilities justify this precaution. If the developers of a frontier AI system cannot be confident that it lacks the capacity to cause enormous damage in the wrong hands, then it makes sense to require the developers to impose appropriate access restrictions. It also does not make sense for the U.S. to justify large public investments in AI on the basis of the need to compete with China while also giving China and other rivals open-source access to some of the most powerful U.S.-developed AI systems.
Indeed, I think California can and should go further. If frontier AI systems cause harm, their developers should pay. That is, as I argue at length elsewhere, they should be held strictly liable. The fact is that, right now, no one knows how to build highly reliable frontier AI systems. So far, that has been okay because GPT-4 level systems lack the sort of hazardous capabilities that SB-1047 targets. But, as this legislation envisions, frontier AI labs may soon start training and deploying systems with catastrophically dangerous capabilities. These labs need to face consequences if their systems cause a catastrophe or cause more limited harms in ways that suggest that catastrophic harm was reasonably likely. And they need to face those consequences regardless of whether the attorney general of California can prove that the safety plan the developer implemented failed to “provide reasonable assurance” that it would prevent critical harm. When faced with safety-critical decisions, we want AI labs to be asking what steps they need to take to make their systems safe, not just what boxes they have to check in order to prevent the California attorney general from being able to prove they fall short of their regulatory commitments.
The strongest argument against strict liability is that it would inhibit innovation. But all strict liability does is make AI developers pay for the harm they cause. If frontier AI systems can be expected to generate more social value than social cost, it should be profitable to invest in building them. Failing to hold AI developers for the harm they cause allows them to externalize the risks generated by their products. Such a policy tends to produce innovations that generate less net social value. Seen in this light, strict liability is rightly viewed as a policy that is well calibrated to encourage responsible innovation.
The rules imposed by SB-1047 on frontier AI model developers are reasonable. Failure to comply with them should be discouraged through the sort of appropriate penalties included in the bill. But AI labs also need to be held strictly liable for the harms caused by their AI systems, at least when those harms arise from the inherently dangerous nature of building systems with unpredictable capabilities and uncontrollable behavior. Strict liability would not be some unfair imposition on AI developers; it would merely require them to pay for the harm they cause and the risks they generate. Standard economic theory tells us that activities that generate negative externalities, like the risk of catastrophic harm from advanced AI systems, will tend to be overproduced. Strict liability, that is, liability that does not depend on whether the AI developer failed to adopt some specific precautionary measure prescribed by legislation or identified by judges in prior cases, would compel AI developers to internalize the risks they generate. Only then will those developers have adequate incentives to take the steps necessary to make their systems safe.
Moreover, strict liability for the harms generated by frontier AI systems is well grounded in the tort law doctrine of abnormally dangerous activities, which applies to high-energy activities like blasting and some fireworks displays, crop dusting, and hazardous waste disposal. According to the Restatement (Third) of Torts, strict liability should attach when “(1) the activity creates a foreseeable and highly significant risk of physical harm even when reasonable care is exercised by all actors; and (2) the activity is not one of common usage.” Training AI models with more than 1026 FLOP of compute is clearly not an activity of common usage. Moreover, while courts may be reluctant to recognize a subset of software development as abnormally dangerous, it seems hard to argue that building AI systems that could plausibly cause more than $500 million of damage, and which we don’t know how to make safe, does not create “a foreseeable and highly significant risk of physical harm even when reasonable care is exercised by all actors.”
That said, to address those cases where a violation of the safeguard requirements is provable, the legislation does have reasonable enforcement provisions. For violations that present an imminent threat to public safety, the bill calls for preventive restraints in the form of restraining orders or injunctions. For violations that result in actual harm, the bill provides not only for compensatory damages but also for punitive damages and shutdown orders. Finally, the legislation provides for civil penalties, capped at 10 percent of the nonlabor costs of training the system for the first violation; this limit rises to 30 percent for subsequent violations.
The inclusion of punitive damages, which was added during the Judiciary Committee markup, greatly strengthens incentives for developers to comply, by enabling harsh penalties for systems that have not yet caused catastrophic harm. A major challenge for AI governance is that frontier AI systems generate uninsurable risks. They might cause harm so great that a compensatory damages award that fully compensates the victims would bankrupt the company and exceed any plausible insurance policy. Accordingly, the threat of compensatory damages is likely to be insufficient to induce AI developers to pay the costs, which may include substantially delaying deployment in addition to financial costs, of making their systems safe.
However, the punitive damages clause lacks specific guidance regarding conditions under which punitive damages would be available or the potential size of those damages. I would like to see this provision strengthened by clarifying that punitive damages calculation should be based on the magnitude of the uninsurable risks generated by training and deploying the system at issue, which may warrant punitive damages many times larger damages needed to compensate the harmed parties. I argue for this punitive damages formulation at length elsewhere.
Similarly, it’s unclear why the civil penalty is tied to the costs of training the system. To be sure, training costs provide an easily calculable indication of the scale of incentive needed to influence developer behavior, but influence should be calibrated according to risk. Indexing penalties to training costs is choosing a precise but conceptually ill-suited target over the uncertain but more meaningful metric of risk. What policymakers should care about is the risk that the system will harm people, particularly the risk that the system will cause catastrophic harms that the law will not be able to adequately hold them accountable for after the fact. These risks may be only weakly correlated with model training costs. Accordingly, the civil penalty provision could also be improved by tying the penalties to some measure of the risks generated by the system, rather than the cost of training it.
Finally, one additional provision I would like to see in this bill is liability insurance requirements that scale with hazardous system capabilities. That is, developers of systems that do not qualify for the limited duty exemption could be required to take out insurance to cover the sort of harms their systems might cause. This would have two beneficial effects.
First, it would expand the range of harms for which compensatory damages can offer effective accountability by mitigating the problem of judgment-proof defendants. Without liability insurance requirements, AI developers would have little incentive to abate risks beyond the level at which a liability judgment would push them into bankruptcy. To be sure, there will still be some limit on the magnitude of a feasible insurance policy, but mandating insurance coverage can at least expand the effectiveness of compensatory damages up to this limit. Then, punitive damages in near-miss cases can be used to internalize the insurable risks.
Second, liability insurance requirements would provide an extra layer of protection for public safety by introducing a more cautious decision-maker into the loop on potentially risky training and deployment decisions. While some AI labs take the risks of catastrophic harms seriously, others have been remarkably cavalier about these risks. Liability insurance mandates would restrain these less cautious developers by requiring them to convince an insurance company to write them a policy they can afford that satisfies the coverage minimum set by a state agency before they can deploy a system with potentially hazardous capabilities. This would provide developers with additional incentives to make their systems safe and deter the deployment of systems that generate risks larger than their social benefits.
Sen. Weiner and his colleagues should be applauded for crafting and championing legislation that would require AI developers to adopt a series of eminently reasonable precautions designed to make their systems safe. However, as things stand, no one knows how to make AI systems safe. Even adopting all the standards promulgated by NIST or recognized as industry best practices will not ensure safety. A key goal of any frontier AI governance legislation should be to push forward the frontier of safety by shifting the onus to the labs themselves to come up with new and better safety practices. Merely requiring them to take enough precautions so that it cannot be proved that they failed to follow industry best practices or “provide reasonable assurance” that their systems are safe will not do this. Proving that safety measures failed to provide reasonable assurance will be extraordinarily difficult in cases where no one had yet thought of a better safety strategy at the time of the alleged violation. As I emphasized above, the best way to encourage frontier AI developers to continually push forward the safety frontier is to make them bear the risk that systems will cause harm. This means imposing strict liability for all harms arising from the inherently dangerous nature of these systems. It also means forcing the developers to internalize the uninsurable risks generated by their systems via punitive damages and civil penalties that seek to pull forward the expected liability associated with those risks.