Frontier AI Regulation: Safeguards Amid Rapid Progress
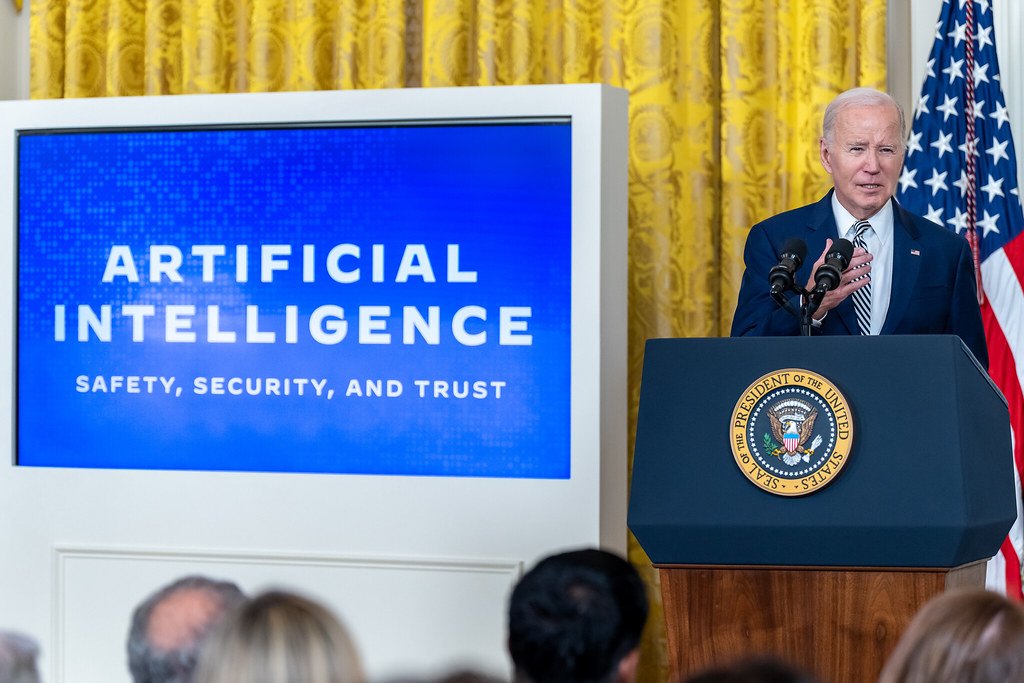
Published by The Lawfare Institute
in Cooperation With
The launch of ChatGPT in November 2022 caught the world off-guard. It sparked conversations across the globe about the enormous potential of artificial intelligence (AI) systems and triggered tremendous investments from tech giants, further accelerating AI progress. At the same time, it opened policymakers’ eyes to the technology’s potential harms—from power concentration to the risk of empowering bad actors’ attempts at disinformation, cyberattacks, and perhaps even acquiring biological weapons. Washington is still reeling, and concerns about these dangers have given rise to a flurry of new policy proposals. Moreover, the recent board turmoil at OpenAI highlighted the shortcomings of self-regulation and, more broadly, the challenges of private-sector efforts to govern the most powerful AI systems. In the coming year, a new generation of generally capable models similar to GPT-4—trained using record amounts of computational power—will likely hit the market. We need to be prepared.
Many of the challenges ahead stem from the development and deployment of the most capable and generally capable models. In a July 2023 white paper we co-authored with a broad coalition of experts from academia, think tanks, and industry, we introduced the term “frontier” AI models for highly capable foundation models that are at the frontier in terms of their capabilities and could have dangerous capacities, sufficient to severely threaten public safety and global security. Capabilities that would meet this standard may include significantly enabling the acquisition of weapons of mass destruction, exploiting vulnerabilities in safety-critical software systems, synthesizing persuasive disinformation at scale, or evading human control.
Although there is significant uncertainty, the next generation of foundation models—in particular, those trained using substantially greater computational resources than any model trained to date—may have these kinds of dangerous capabilities. We believe the likelihood for this is high enough to warrant their targeted regulation.
There is a growing global consensus that the most advanced AI systems require special attention. In July 2023, the Biden administration invited the leaders of seven frontier AI companies to the White House and had them voluntarily commit to a set of practices to increase the safety of their systems. At the U.K.’s AI Safety Summit in November, 28 governments—including the U.S. and China—signed the Bletchley Declaration, stating that “[t]here is potential for serious, even catastrophic, harm, either deliberate or unintentional, stemming from the most significant capabilities of [frontier] AI models.” In early December, the EU came to a provisional agreement on the long-awaited AI Act, introducing a special category for foundation models posing “systemic risk,” including those trained using roughly the same amount of computational power as today’s most powerful models.
Much recent progress in AI has stemmed from harnessing huge amounts of computational power to train a handful of systems. One analysis finds that the computing power (compute) employed to develop noteworthy AI systems has increased by 4.2 times every year. Over the past 13 years, the amount of compute used to train notable AI systems has increased by a factor of 350 million. Concurrently, the AI industry has moved toward more general models, capable of engaging in a wide range of tasks. Previous models focused on a specific modality, such as vision, and tended to be specialized in particular tasks. Today’s most widely used and advanced systems, by contrast, like Google’s recently announced Gemini, can see, hear, read, write, speak, code, and produce images.
AI researchers expect progress to continue. In a recent survey of over 2,700 researchers who have published at top AI conferences, the median researcher placed a 50 percent chance that human-level machine intelligence—where unaided machines outperform humans on all tasks—will be achieved by 2047. That’s 13 years earlier than the forecast of the same sample just a year ago.
To deal with the risks of these high-compute frontier AI systems, we must govern not only how they can be used but also how they are developed and made available to people in the first place. President Biden’s recent AI executive order serves as an important first step in this direction in the U.S. by introducing reporting requirements for advanced models, trained using more computational power than any to date. However, regulatory oversight must not stop there. There is currently more public oversight and accountability for constructing an apartment building than there is for building frontier AI models. As the stakes of this technology increase, the problems associated with this lack of oversight will become increasingly dire. Further efforts will be required to ensure models’ safety and security, addressing malicious misuse of AI, reducing bias and discrimination, boosting innovation, protecting workers, and more. And the public should have a say in such decisions.
Why Regulate Frontier AI Systems’ Development and Deployment?
Effectively regulating the use of frontier AI, intervening as close as possible to the harm, can address many of the relevant challenges. Many of AI’s harmful uses are already illegal, from criminal impersonation to cyberattacks and the release of dangerous pathogens. And there are certainly additional steps we can take to make it harder to use AI capabilities for ill. The AI executive order, for example, included a whole subsection about introducing standards for DNA synthesis companies to screen their orders for dangerous pathogens, thereby reducing the risk that AI systems are used to acquire bioweapons.
However, in certain cases, it will be necessary to intervene earlier in the AI value chain, at the stages where decisions are made to develop and deploy highly capable systems. To tackle AI-generated misinformation, model outputs should include watermarks, ensuring citizens can determine when they are presented with AI-generated content. To reduce the chance of bioterrorism attacks, access to systems that could identify novel pathogens may need to be restricted to vetted researchers. To ensure that safety-critical AI systems are built on solid foundations, reducing the chance of accidents, widely used foundation models should be designed with a particular focus on transparency and ensuring they behave predictably. To address cybersecurity risks, systems that can identify novel software vulnerabilities should be used to patch exploits before they are made available to hackers.
This kind of multilayered approach (regulating the development, deployment, and use of AI technologies) is how we deal with most safety-critical technologies. In aviation, the Federal Aviation Administration gives its approval before a new airplane is put in the sky, while there are also rules for who can fly the planes, how they should be maintained, how the passengers should behave, and where planes can land.
The recent executive order imposes certain productive safeguards for frontier AI development. The reporting requirements for models trained using more computational power than any model trained to date (10^26 operations) will give the government much-needed insight into the systems companies are developing and deploying, as well as the risks they might pose to safety and national security. Relying on Defense Production Act authorities, the order requires developers of such models to inform the federal government about their planned and ongoing development activities, how they intend to keep models from being stolen, the results of red-teaming efforts to identify the systems’ dangerous capabilities, and whether the systems adhere to relevant technical standards. Beyond increasing the government’s visibility into the frontier of AI, the executive order’s requirements might also provide incentives for companies to behave more responsibly: They would prefer to be able to answer yes to questions like, “Are you adhering to the National Institute of Standards and Technology’s [NIST’s] AI Risk Management Framework?“
But these requirements won’t be enough. The U.S. government still lacks many of the authorities needed to act on any concerning information it may receive. If the Department of Commerce were made aware that a model with significantly dangerous capabilities were to be deployed without adequate safeguards, it’s not clear what—if anything—the government could do to intervene. While there are some authorities that could potentially be used to halt the spread of dangerous capabilities—for example, emergency authorities of the president, the Federal Trade Commission’s powers to target unfair business practices, and Department of Energy authorities to prevent the distribution of weapons of mass destruction-related capabilities—none of them are designed for the purpose.
Effective frontier AI regulation would require that developers of the most capable systems make a substantial effort, using a significant amount of resources, to understand the risks their systems might pose—in particular by evaluating whether their systems have dangerous capabilities or are insufficiently controllable. These risk assessments should receive thorough external scrutiny from independent experts and researchers and inform regulatory decisions about whether new models are deployed and, if so, with what safeguards.
For the riskiest systems—such as those that could cause catastrophic damage if misused—we might need a “safety case” regime, where companies make an affirmative case to a regulator that these systems do not pose unacceptable risks to society. Much like in other safety-critical industries, such as pharmaceuticals, aviation, and nuclear power, it should be the companies’ responsibility to prove their products are safe enough, for example, via a broad range of safety evaluations. The role of regulators should be to probe the evidence presented to them and determine what risks are acceptable. Flipping the script—disallowing only those models that have been proved unsafe by the regulator—appears inappropriate, as the risks are high and industry has far more technical expertise than the regulator.
After deployment, companies and regulators should continually evaluate the harm caused by the systems, updating safeguards in light of new evidence and scientific developments. Other requirements will also be appropriate. For example, developers should adhere to high cybersecurity standards to thwart attempts by malicious actors to steal their systems.
Based on current trends, creating frontier AI models will likely soon cost upward of hundreds of millions of dollars in computational power and also require other scarce resources like relevant talent. The regulatory approach we describe would therefore likely target only the handful of well-resourced companies developing these models, while posing few or no burdens on other developers. Nonetheless, by increasing the burdens to those developing the most advanced systems, the market for such systems may become more concentrated. Governments should therefore subsidize smaller players—for example, via a National AI Research Resource—and wield antitrust powers to address excessive accumulation and abuse of market power.
What Are the Challenges for Frontier AI Regulation?
There are three core challenges for regulating frontier AI models: their unpredictable capabilities, the difficulty of stopping them from being used to cause harm, and the speed with which capabilities diffuse once released.
The Unpredictable Capabilities Problem
The capabilities of frontier AI models are not reliably predictable and are often difficult to fully understand even after intensive testing. Researchers have repeatedly observed unexpected capabilities in foundation models. It took nine months after GPT-3 was widely available to the research community before the effectiveness of chain-of-thought prompting—where the model is simply asked to “think step-by-step”—was discovered. Researchers have also regularly induced or discovered new capabilities after model training through techniques including fine tuning, tool use, and prompt engineering. Therefore, dangerous capabilities could arise unpredictably and—absent requirements to do intensive testing and evaluation pre- and post-deployment—could remain undetected and unaddressed until it is too late to avoid severe harm.
The Deployment Safety Problem
Harmful consequences from AI systems can ensue for several reasons, even if neither the user nor the developer intends harm. First, it is difficult to precisely specify what we want deep learning-based AI models to do, and to ensure that they behave in line with those specifications. In other words, reliably controlling AI models’ behavior remains a largely unsolved technical problem. Second, attempts to “bake in” misuse prevention features at the model level, such that the model reliably refuses to obey harmful instructions, have proved circumventable due to methods such as “jailbreaking.” For example, one class of jailbreaks uses role-playing to have the model ignore its safety instructions. In April 2023, a user found that ChatGPT would provide instructions for producing napalm when asked to pretend to be the user’s recently deceased grandmother who would recount the instructions as a bedtime story. Finally, distinguishing instances of harmful and beneficial use may depend heavily on context that is not visible to the developing company.
Even with the adoption of state-of-the-art deployment safeguards, robustly safe deployment is difficult to achieve and requires close attention and oversight. Further, developers can’t simply bolt on safety features after the fact; the model’s potential for harm must be considered at the development stage. To reduce risk, models may need to be intentionally developed so as to lack certain dangerous capabilities—for example, by removing certain data from the training data—and to be sufficiently controllable.
The Proliferation Problem
Once a frontier AI system is developed, its capabilities often diffuse quickly. OpenAI’s ChatGPT reached 100 million users in under two months. And it took just roughly two years after the release of GPT-3 for a similarly capable open-source model to be released. Such diffusion is generally a good thing: AI technologies widen opportunities, increase productivity, and boost innovation. But when an AI system can be used to cause significant harm, it poses regulatory challenges.
Since producing frontier AI systems is harder than using them, diffusion significantly lowers the barriers to the misuse of frontier AI. AI system flaws and vulnerabilities are relatively easy to find and manipulate, yet they are much more difficult to guard against and fix than in the case of traditional software. Non-proliferation of certain frontier AI models is therefore essential for safety; but it is difficult to achieve. As AI models become more useful in strategically important contexts, and the costs of producing the most advanced models increase, AI companies will face strong incentives to deploy their models widely—even without adequate safeguards—to recoup their significant upfront investment. But even if companies agree not to distribute their models, bad actors may launch increasingly sophisticated attempts to steal them.
Further, when models are open sourced, accessing or introducing dangerous capabilities becomes much easier. One recent study found that the safety filters in one of Meta’s open-sourced models could be removed with less than $200 worth of technical resources. While we believe that open sourcing of non-frontier AI models is currently an important public good, open sourcing frontier AI models should be approached with great restraint.
Together, these challenges underline why regulating the development of frontier AI, although difficult, is urgently needed.
What Could Safety Standards for Frontier AI Development Look Like?
While AI frontier development is a fast-evolving field, where best practices are yet to emerge and coalesce, there are concrete actions developers of frontier models could take to respond to these challenges and behave more responsibly, many of which are described in detail in the white paper referenced above. Companies should begin to adopt these standards proactively, though government intervention will ultimately be necessary for their effective implementation (more on that issue in the next section).
Conduct thorough risk assessments informed by evaluations of dangerous capabilities and controllability. This would reduce the risk that deployed models possess unknown dangerous capabilities or behave unpredictably, unreliably, or both.
Engage external experts to apply independent scrutiny to models. External scrutiny of the safety and risk profiles of models would both improve assessment rigor and promote accountability to the public. For such scrutiny to be effective, it must be independent, armed with sufficient resources, and take a “searching attitude”—actively seeking out model flaws, rather than mechanically testing against fixed benchmarks.
Commit not to deploy models in ways that would pose unacceptable risks to society and provide clarity about how they intend to do so. The results from risk assessments should determine whether and how a model is deployed and what safeguards are in place. Options could range from deploying the model without restriction to not deploying it at all until risks are sufficiently reduced. In many cases, an intermediate option—deployment with appropriate safeguards, such as restrictions on the ability of the model to respond to certain, potentially risk-inducing, instructions—will be appropriate. Determining what constitutes unacceptable risk is a considerable challenge. Presently, frontier AI companies themselves are trying to do so: Anthropic in its Responsible Scaling Policy and OpenAI in its Beta Preparedness Plan. However, ultimately these decisions will have to involve governments, external experts, and the affected parties.
Monitor and respond to new information on model capabilities. The risk of deployed frontier AI models may change over time due to new information about how it is used in the real world and the application of additional (potentially novel) post-deployment enhancement techniques, such as fine tuning. If significant information concerning a model’s capabilities is discovered after the model is deployed, companies should repeat risk assessments and update deployment safeguards accordingly.
Ensuring sufficient information security. As the value of frontier models increases, there will be more sophisticated attempts at stealing, compromising, or abusing them and their related intellectual property. Companies developing the most advanced AI systems should therefore uphold high information security standards, proportional to the capabilities of their models.
These standards should likely change substantially over time as we learn more about the risks from the most capable AI systems and the means of mitigating those risks. For example, smaller, potentially open-source, models could come to dominate the AI ecosystem, in which case regulatory focus may need to shift toward how systems are used; AI systems may prove less risky than anticipated, in which case the regulatory burdens may need to be reduced; the pace of AI development may slow, in which case policy could become more reactive, responding once more harms have materialized. Further, other standards would also likely be appropriate. For example, AI-generated images and videos should be watermarked to allow users to know when they are engaging with AI-generated content. Companies could also commit to making significant investments—say, spending at least 20 percent of their budgets—to improve the safety of their systems.
What Regulatory Building Blocks Are Needed for Frontier AI Regulation?
Government intervention will be needed to deal with the risks from frontier AI systems, likely coordinated and led by a new federal regulatory body. The body could be housed in the Department of Commerce, drawing heavily on the expertise in the recently announced AI Safety Institute. Self-regulation is a welcome and useful first step, but it is very unlikely to provide sufficient protection, especially as the capabilities of AI systems as well as the number of players and associated competitive pressures in the sector grow. Frontier AI development has moved from the lab to the marketplace.
A regulatory regime for frontier AI would require multiple building blocks:
Create and update standards for responsible frontier AI development and deployment. These should be developed via multi-stakeholder processes, perhaps convened by NIST, relying on expertise from academia, civil society, and industry. Developed standards may be relevant to foundation models overall—not just frontier AI. The standards would need to be rapidly iterated to keep pace with the technology.
Give regulators visibility into frontier AI developments. These mechanisms could include disclosure regimes (as introduced in the recent executive order), monitoring processes, whistleblower protections, and model registration. The goal would be to equip regulators—existing ones, as well as a new frontier AI-focused regulator—with the information they need to identify appropriate regulatory targets and design proportionate regulatory requirements. The information provided would pertain to qualifying frontier AI development processes, models, and applications. For example, it may initially apply only to the systems facing reporting requirements, as per the recent executive order. Further, regulators will need substantial expertise to understand the information they receive. Investing in government AI talent, in particular with respect to the most advanced models, is paramount.
Work to ensure compliance with safety standards. Self-regulation efforts, such as voluntary certification, while helpful, will ultimately be insufficient without government intervention. Intervention may involve empowering a government authority to translate standards into legally binding rules, identify and sanction noncompliance with rules, and establish and implement a licensing regime for the deployment and potentially the development of frontier AI models. Tort liability is another important tool, though holding companies liable for reckless deployment decisions would likely require passing new legislation.
Getting the regulatory target right for such a regime will be difficult. Targeting AI models trained using significant amounts of computational power—for example, 10^26 operations as in the executive order or 10^25 as in the prospective EU AI Act—is a first step. However, the target will need to be continually refined: The computational power used to train a system is just a proxy of its capabilities, which in turn is only a proxy of the risk the system poses. The threshold of computational power may need to be adjusted over time. Further, our understanding of what systems are capable of and what risks they pose are nascent. As our understanding improves, the regulatory target will need to be sensitive to what data the model is trained on, how widely the model is used, and whether it is likely to have certain dangerous capabilities of concern. This is already recognized in the recent executive order, which sets a lower training compute threshold, 10^23 operations, on systems trained on biological sequence data.
Designing a well-balanced frontier AI regulation regime may be the most challenging regulatory task in the history of technological regulation. Governments need to be sensitive to the risks of overregulation and stymieing innovation, on the one hand, and the risks of moving too slowly (relative to the pace of AI progress), on the other.
Conclusion
As frontier AI systems continue to rapidly advance, it is imperative to implement guardrails to ensure firms’ responsible development and deployment of these powerful technologies. This must be done in addition to efforts to restrain dangerous uses of the technology. Further, self-regulation alone will not suffice: Government intervention and oversight will be needed to mitigate risks—known and unknown—inherent in the most capable AI models. By establishing safety standards, improving visibility into frontier AI developments, and instituting compliance mechanisms, we can harness AI’s tremendous potential while safeguarding the public interest.