How Crowd-Forecasting Might Decrease the Cybersecurity Knowledge Deficit
Can we apply the techniques of crowd-forecasting for better cybersecurity?
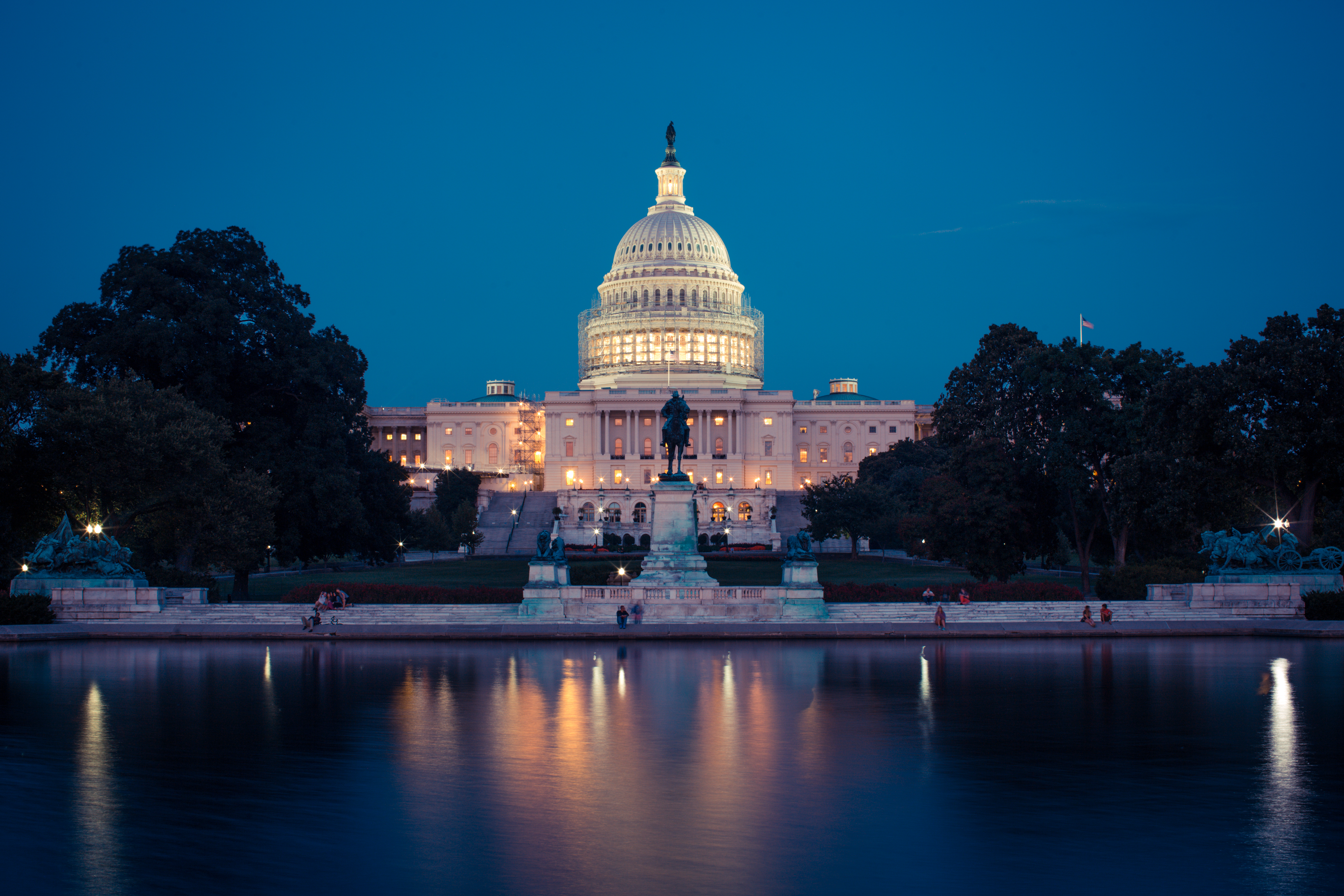
Published by The Lawfare Institute
in Cooperation With
What if it were possible to predict, with a measurable degree of accuracy, what actions the U.S. government and private sector will undertake over the course of the next year to harden the United States’ overall cybersecurity posture?
To answer this question, we might ask government officials, intelligence analysts or congressional staffers what they expect to see. We might analyze industry trends or statements by leading security teams. We might poll chief information security officers (CISOs) and policy experts. We might throw up our hands and maintain that speculating on questions like these is ultimately futile.
Or we might try to predict the future more directly. In recent years, crowd-forecasting has slowly edged its way into the decision-making space, as it offers the promise of predicting—with a fair degree of accuracy—what events might happen in the future. Though still somewhat novel and at times even controversial, the appeal of crowd-forecasting centers on the belief that sufficiently large groups of people who are incentivized to share expectations about future events will accurately predict outcomes. Crowd-forecasting may utilize different techniques and be directed at diverse outcomes, but ultimately it endeavors to ask and answer questions that may have profound implications for individuals, industry and institutions.
Given the spate of high-profile hacks and cyberattacks in recent years, now may be the optimal moment to apply these techniques to cybersecurity. Building on our earlier work, here we first provide an analysis of the ways in which the cybersecurity knowledge deficit harms security outcomes. We then ask how crowd-forecasting might apply to cybersecurity and what it could teach interested parties. In doing so, we focus on the ability of the crowd-forecasting platform to ask questions that are meaningful and actionable for decision-makers. We conclude by offering some ways that these questions can be shaped, and some examples of what this approach could look like.
A Knowledge Deficit in Cybersecurity
Information in cybersecurity is underdetermined. There are many things we—individuals, industry, the government—do not know about cybersecurity. And similarly, there are many things that only some people may know, but that are relatively unknown by the majority of people in the cybersecurity ecosystem.
This knowledge deficit makes it more difficult for the U.S. government to effectively diagnose and mitigate its current cybersecurity challenges. Ignorance hinders the ability to examine blind spots, verify assumptions, and grasp both the extent of existing problems and the capabilities to address them.
The question, then, is whether it is possible to do something about this deficit. For example, some observers believe that better measurements and the consolidation of existing traditional metrics will help address the deficit and are advocating for the creation of a “Bureau of Cyber Statistics.”
However, some aspects of this knowledge deficit likely go beyond what is currently possible to measure and quantify. It remains an open question whether the information that the cybersecurity and information security communities collect has actionable utility. It is also far from obvious that the government even knows the right questions to ask—that is, the questions with answers that might diagnose ongoing or imminent incidents with sufficient clarity to act on those events, or the policies and regulations that might best mitigate them. It is similarly difficult to ascertain the depth and “resolvability” of this knowledge deficit. Because relationships—between actors and targets, people and machines, technology and the environment—are dynamic and impossible to model fully, there are whole sections of cybersecurity, as in many other realms, that cannot ever be adequately examined.
In other words, there is likely a difference between what is fundamentally a knowable question, and what is practically a knowable question. The former might consider whether the traditional security concept of “deterrence” can be successfully applied to cybersecurity, while the latter might engage with whether current efforts to establish deterrence make a measurable impact.
Our focus here is practically knowable questions: things that could be known but are not—or not widely enough. This deficit exists because people are not doing certain things: asking the right questions, fully utilizing existing data, correctly interpreting data, nor sharing said data with those who most need it.
We believe that there is a wealth of existing cybersecurity information that could be found, shared, analyzed and acted upon—and that one way to elicit this missing information is through crowd-forecasting.
The Promise of Crowd-Forecasting Applied to Cybersecurity
In an earlier post, we posited that it would be worthwhile for a crowd-forecasting platform to identify trends in cybersecurity that could then be utilized by policymakers, CISOs, security researchers and other decision-makers. There is currently a robust landscape of prediction markets and other crowd-sourced forecasting options, and a fair amount of research into which techniques are most effective and why.
But before we make the effort to set up a market and test this hypothesis, we must first answer the question of efficacy. Is it worth it? Can relevant trends in cybersecurity and vulnerability be predicted using crowd-forecasting?
Our goal here is very specific: We want to ensure that we are evaluating trends that will make it easier for practitioners and policymakers to improve cybersecurity at a fundamental level. This focus underpins not only the type of questions we can ask but also how a crowd-forecasting platform would ultimately be structured. More importantly, this effort should help us decide whether these efforts could contribute meaningfully to cybersecurity.
Though perhaps not entirely conventional, crowd-forecasting to generate cybersecurity information has been explored by others. We have reason to believe that several private—and often classified—attempts have been made, the record of which is unsurprisingly difficult to pin down and exists mostly in anecdote and rumor.
Our goal in this post is to explore—and answer—the question of utility. We conclude (somewhat to our surprise) that the effort is at least testable in a practical way. In a companion piece, forthcoming in December from the R Street Institute, we will lay out the practical aspects of how such a market might work. Taken together, these two pieces suggest that a real-world beta test of the hypothesis is worth pursuing.
What Might Crowd-Forecasting Do for Cybersecurity?
Our goal is to create a cybersecurity forecasting platform that can support the following efforts:
- The platform could aggregate information, turning private information into collective public wisdom and enabling us to better understand the industry. We expect that the structure of the platform would incentivize new source collection and analysis, systematically bring together new information and insight, and encourage individuals and companies to fully utilize data they may already have but currently lack an incentive to process or use.
- In a crowd-forecasting platform, good information rises and bad information is penalized. As “noise” or information overload is a perennial problem for government and industry alike, the platform could serve to decrease the needed number and type of sources.
- The platform can provide a testing ground to verify the information. For example, conclusions generated via other methods can be compared to results from the crowd-sourced platform to see if similar results are achieved across techniques. Similarly, after an event has occurred, it should be possible to test the accuracy of all the techniques against the true outcome of the event, to determine the predictive capacity of the platform and to better understand how expectations from platform participants are shaped and in turn shape responses on the platform. This may help us reject or validate other ideas about what is actually useful.
Asking the Right Questions
In theory, a crowd-forecasting platform can ask virtually any question that is clearly defined and circumscribed, contains mutually exclusive possible outcomes or answers, is time constrained, and is knowable. But to optimize usefulness for practitioners and policymakers, the information generated needs to be robust, be applicable and have a broad aperture. Questions that deal in minutia may be interesting to market watchers but are less relevant for policymakers. In short, what are the questions that should be asked?
The life cycle of a good cybersecurity crowd-forecasting model involves a three-step process—identification, generation and operationalization. We describe each step more fully below.
Step One: Identification
If the premise for a prediction platform is generating usable cybersecurity forecasts, any questions asked should have broader relevance for the cybersecurity industry.
This means that the first and most important step is to decide what, ideally, the platform should tell us. The answer to this question will depend on what information is available to feed into the market and what information decision-makers want to see aggregated or generated. In our view, the potential questions will fall along a broad spectrum—some will be exceedingly technical, others will be industry focused, and others will be oriented toward policy preferences and outcomes. All are legitimate areas for domain inquiry.
For a question to be relevant, its answer should ideally provide information about the state of the industry in a way that the government or private sector can use or might be able to use in the future.
This can be put into practice by returning to the opening question of this post: What actions will the U.S. government and private sector undertake over the course of the next year to harden the United States’ overall cybersecurity posture?
As it is, this is not a question that can be asked of a prediction platform: It is far too vague and open ended, despite its value as a policy question. It is, however, possible to pare down to a more implementable level of questioning in the next step of the cycle.
For now, the important point is that this is the type of high-level question we fundamentally want to be answered: one that we believe has direct utility not only to government decision-makers but also to shareholders, CISOs, and legal and financial analysts alike. It’s fair to say that hardening one’s security posture should, ostensibly, make an entity more difficult to compromise in a ransomware operation—and also more difficult to extort for a ransom even in the event of a compromise. That is to say, this question could have relevance for those tracking the ebbs and flows of the ransomware crisis. Similarly, it could have utility for regulators, cyber insurance analysts, compliance officers, private security companies and more.
Step Two: Generation
This is the space in which the platform operates. Here the focus is: Of the questions identified in step one, which have answers that are mutually exclusive and promptly verifiable?
Some questions are easily answered and easily provable. Some are not. For example, while it is possible to answer the question of whether the U.S. intelligence community is willing to officially attribute the SolarWinds hack to the Russian government, this is a far different question than whether the Russians did commit the SolarWinds hack.
Next: What are the questions that should not be asked?
The platform must remain fairly high level as too much specificity can be dangerous. If the platform delves too deeply into examining specific products or markets, it could incentivize targeting of organizations that are perceived to be weaker by market participants—in essence, low-hanging fruit for criminal cyber actors. In short, questions of the form “Will it become publicly known that small company X’s systems were locked up by a ransomware attack in the coming 12 months?” should be avoided. (This problem is not unique to cybersecurity. In political prediction markets, this type of singling out is also a concern—for example, asking about the date of death of a prominent celebrity or politician could turn into an assassination market.)
The second step is to structure the relevant cybersecurity questions in step one, so that they can be asked on the platform. At this point, platform participants can issue their predictions.
Here are two exemplars (of many possibilities) for suitably structured questions that would address our earlier interest in the strengthening of cybersecurity measures:
- Will a U.S. incorporated insurance company decide to stop binding coverage for ransomware payments before Dec. 31, 2022?
- Will the U.S. government pass a know-your-customer law for cryptocurrencies before July 31, 2022?
These questions are clearly defined and scoped, and thus are of the type that can be asked of a crowd-forecasting platform. They are also relevant to cybersecurity, can be verified in a timely manner, and do not (seem to) raise red flags as questions that should not be asked.
We recognize that these are sample questions and that we would be required to more clearly build out what events might trigger the resolution of a question. For example, we would need to specify the features of a know-your-customer law, adding some nuances and precluding others. Observe, for example, the fine print in this existing platform question.
Step Three: Operationalization
The final step in this knowledge cycle—though perhaps not the last chronologically—is to ensure that the effort being expended is generating meaningful, accurate, and useful results, and that those results are usable and accessible for decision-makers.
Consider the opening question of this post, which is high level and policy oriented: Will the U.S. government and private sector take steps over the course of the next year to harden U.S. cybersecurity posture? As mentioned previously, it seems like a safe assumption to claim that hardening cybersecurity posture will make companies more impervious to ransomware operations—and thus usher in either a diminishing ransomware crisis or generate novel attempts at compromise on the parts of ransomware actors. The discrete questions asked in step two should be—when taken in combination—indicative of a broader trend about U.S. cyber defense, the ability of stakeholders to coordinate and work together, and new requirements or challenges to navigate.
But at the individual level, we also expect the questions to have value. Let’s say we did ask the above question—Will a U.S. incorporated insurance company decide to stop binding coverage for ransomware payments before Dec. 31, 2022?
What might we personally infer from the crowd’s position?
Of course, there is always a risk in imputing causality, and doing so is technically not part of the remit of the prediction platform itself (step two)—that is, how the information is used, not how it is generated. Nevertheless, a functional prediction platform would presumably not only solicit information but also offer a method of examining assumptions and causality. For that reason, we’ve sketched out below how the information gleaned from a market might be treated. By “strong” and “weak,” we are referring to the probability of likelihood assigned by most respondents; strong means a high probability, while weak suggests a low probability:
- What a “strong yes” could tell us: That losses exceed what the rate-paying market is willing to bear, and so individual insureds are likely to be on their own as insurers drop back.
- What a “weak yes” could tell us: That choosing an insurer has become crucial in any company’s risk mitigation plan as insurers clearly do not have an across-the-board handle on outcomes.
- What “ambivalence” could tell us: To watch like a hawk.
- What a “weak no” could tell us: That a discontinued insurance program is a management failure at that insurer, not an industry failure.
- What a “strong no” could tell us: That ransomware is pervasive enough that no insurer can dare stop covering it unless they plan to fold up their cyber insurance portfolio entirely.
Ultimately, however, the value of the platform we seek to promote lies in its usefulness to decision-makers—including their ability to trust the platform, their own openness toward information derived via crowd-forecasting methods, and their ability to ingest the information and make inferences and deductions. It is our belief that, while a simple yes or no answer on a question may at times be valuable—like, will candidate XX win the 2024 presidential election—the ability for decision-makers to use the information will ultimately depend on their own processes for information adjudication and resource allocation.
Conclusion
At this point, our theory of a platform for crowd-forecasting cybersecurity is articulated fairly robustly. Naturally, the question turns to implementation: What might the platform look like? What are the options for a beta version? Whose opinions should be solicited? What questions should be asked? Our forthcoming paper, “Betting on Cyber: An Analytical Framework for a Cybersecurity Crowd-Forecasting Platform,” to be published in early December through the R Street Institute, will offer answers to these practical concerns. Our conclusion is that the practical problems of creating a market are likely solvable.
Our next step is to go and test that belief. We hope to build and run a beta test of the concept with real-world experts and real-world questions.
In pursuing this venture, we are both optimistic and cautious. We think the concept of crowd-forecasting offers promise. We think it can be part of an effort to reduce the cybersecurity knowledge deficit. And, in the end, we think that asking and answering relevant cybersecurity questions can aid decision-makers across the cyberspace domain—and ultimately increase security, improve outcomes and facilitate resource allocation.
Anyone interested in participating in a cyber prediction beta can send a note to us at cyberpredictionbeta@gmail.com.