Lawfare Daily: Ben Brooks on the Rise of Open Source AI
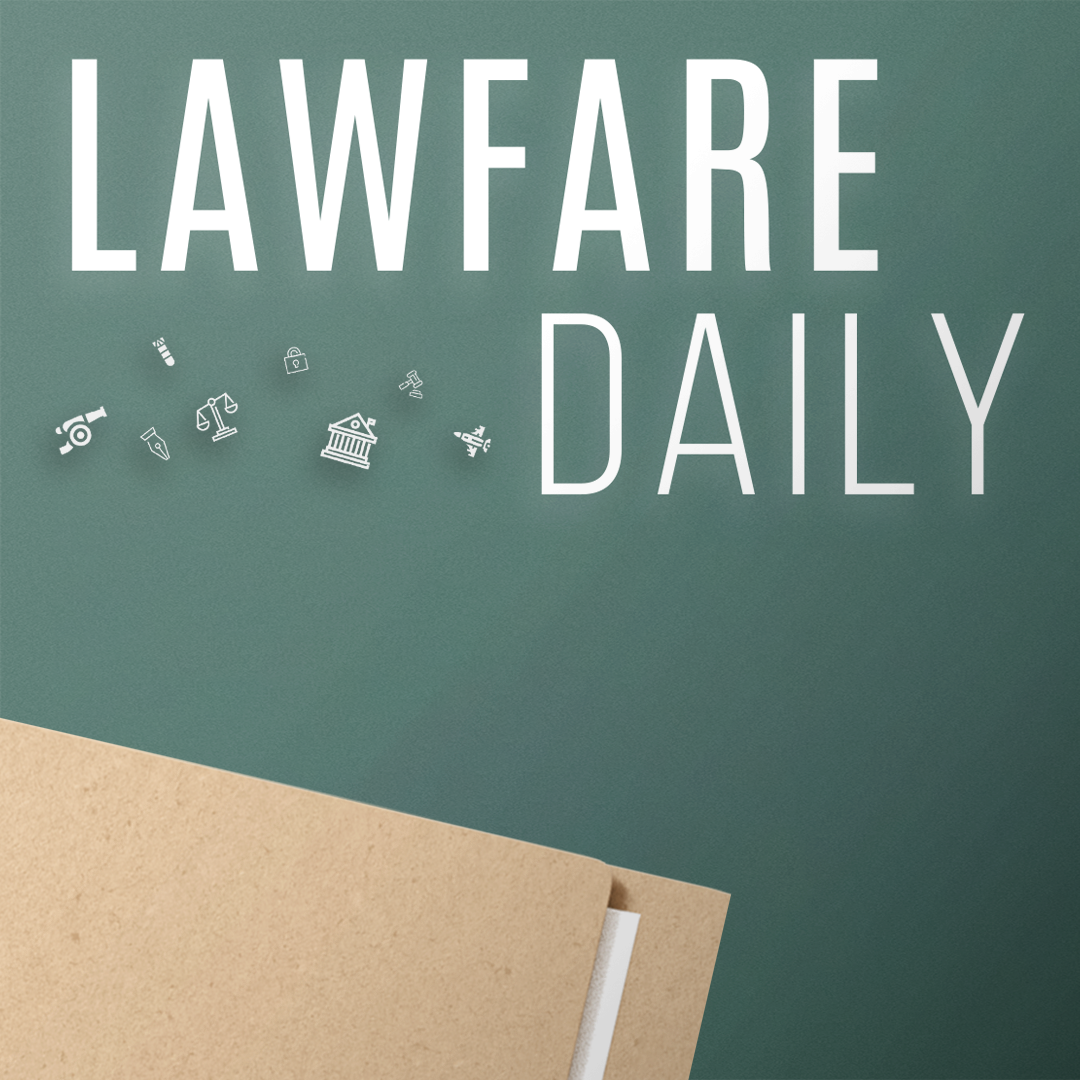
Published by The Lawfare Institute
in Cooperation With
Ben Brooks, a fellow at Harvard's Berkman Klein Center and former head of public policy for Stability AI, joins Kevin Frazier, AI Innovation and Law Fellow at Texas Law and Contributing Editor at Lawfare, to discuss a sudden and significant shift toward open-sourcing leading AI models and the ramifications of that pivot for AI governance at home and abroad. Ben and Kevin specifically review OpenAI’s announced plans to release a new open-weights model.
Coverage of OpenAI announcement: https://techcrunch.com/2025/03/31/openai-plans-to-release-a-new-open-language-model-in-the-coming-months/
To receive ad-free podcasts, become a Lawfare Material Supporter at www.patreon.com/lawfare. You can also support Lawfare by making a one-time donation at https://givebutter.com/lawfare-institute.
Click the button below to view a transcript of this podcast. Please note that the transcript was auto-generated and may contain errors.
Transcript
[Intro]
Ben Brooks: Maybe China is going to use open weight models to essentially ship their technology, their intangible technology around the world and create dependencies on Chinese industry. The challenge is if these models have embedded vulnerabilities, then what, what does that mean for the future of, of the, the digital economy?
Kevin Frazier: It's the Lawfare Podcast. I'm Kevin Frazier, the AI Innovation and Law Fellow at Texas Law, and a contributing editor at Lawfare, joined by Ben Brooks, a fellow at Harvard's Berkman Klein Center, and former head of Public Policy for Stability AI.
Ben Brooks: If the U.S. withdraws, if it, if it pulls up the drawbridge, if it tries to, to cut off the flow of this open technology through either through enhanced chip controls or through controls on intangible technology, then there's a vacuum there and, and China will decouple and other jurisdictions will start to fill that, that vacuum.
Kevin Frazier: Today we're chatting about open source AI models, a hot topic that is top of mind for everyone from Open AI's, Sam Altman, to leading national security groups like the Center for a New American Security.
[Main podcast]
Alright, so Ben, open source, like responsible AI or human-centered tech is the sort of phrase that generally has positive connotations, but is capable of nearly endless definition.
And so I just wanna set the stage for listeners who perhaps aren't deep in the weeds of open source AI. What does that actually mean? And perhaps most critically, why should we care what open source AI means?
Ben Brooks: I mean, to your point Kevin, that you know, you ask a million people to define open source, you'll have a million answers. There is this fascinating and very important tribal war taking place around the definition of open source. The Open Source Initiative, which is kind of the, the custodian of the canonical definition of open source is a very particular definition, which we can get into.
But broadly speaking, from a policymaker and a and a regulatory perspective, I think what matters is simply the idea that the weights, the distinctive settings or parameters for the model, are publicly available, which means that a developer can come along, download those weights, integrate that model into their own system, modify the model, and inspect the model. And, and I think for, for government and for the public authorities, that's what matters the most.
Kevin Frazier: The reason why this open source approach is so controversial, why we have tribes that exist for a open source approach to AI or a closed model approach. Can you highlight what these dividing lines are? What are the general 10,000 foot level concerns about some of the economic concerns that may arise from open versus closed, and some of the national security implications that may arise from open versus closed?
Ben Brooks: Yeah. So I think, and, and without wanting to, to tire anyone with too broad a brush, I think you can, you can characterize the three buckets that have emerged in this, in this debate, right?
So at one end of the spectrum, you have people, civil society groups, researchers, who believe we don’t know enough about these architectures in these models. We can't predict their capabilities. We shouldn't be developing them in the first place, let alone releasing them openly. I have a lot of respect for that position. I think it makes sense within its own system of values and assumptions.
At the other end of the spectrum, you've got the kind of effective accelerationist who say, you know, not only should we develop this, but we should absolutely release these models and these technologies openly. You know, patron saint Marc Andreessen. It is found favor in this administration or parts of this administration. And again, and I have a lot of sympathy with that position. I think that that position makes a lot of sense within its own system of values.
I think there's this very sort of interesting middle ground that's emerged where you have a set of companies, civil society orgs, researchers who say, we should develop these models by all means. But we should be very cautious about releasing them and making them available openly. Just say making the weights available openly. And I think that position deserves a lot of scrutiny and that's where I spend most of my time and, and attention.
And, and, and I think if you break that down, there's a sort of underlying assumption there that limiting or restricting access to models or restricting the capabilities of models is the primary and maybe the only effective mitigation against the worst risks. We're talking about catastrophic risks or misuse, the risk of sort of accidental or runaway behaviors. And, and I think folks in that bucket tend to look at the model as being the primary choke point for those interventions.
Kevin Frazier: And so I kind of think of this open source debate as a debate reserved for AI insiders, for lack of a better phrase, it's like those who know, know about open source and everyone else is just talking about X risk or acceleration of AI. But the folks really in the weeds are, are having this conversation, but all of a sudden we see headlines talking about open source AI. We see national security folks talking about open source.
So can you walk us through this evolution of open source being a sort of issue that only the folks who are nerdy enough to talk about AI on a podcast at 10:00 AM on a Tuesday would care about versus having this general conversation? Why, why is this such an important issue now? And how are we seeing it become even more of an issue, both from a national security perspective and in terms of this more general domestic competition among AI labs conversation?
Ben Brooks: Well, there's, there's always been this, this kind of latent debate in the regulatory and national security space around, right, dual use technology.
And so if you rewind back to the nineties and even before that, you see this fascinating line of debates- ultimately ending up in litigation, much of it unresolved- around, you know, what, what are the limitations on government restricting access to useful and capable intangible technology. So not talking about hardware and chips and parts of the physical infrastructure. We talking about access to research, software, data and lastly, weights.
This kind of, you know, bubbled along in different ways. You know, a copyright space, criminal law, financial regulation. There have occasionally been moments where government is kind of confronted with that question: what do we do about capable software or intangible systems that can be used to do good things, but can also be used to do bad things?
And then when you suddenly have this explosion of interest in generative AI, these concerns really started to dominate the conversation, right? Because what do you have? We unpack generative AI. What does that mean? It means you have a versatile system that can do lots of things. Some of them intended, some of them unintended.
They are relatively opaque. We haven't solved interpretability. We can observe the system, but we don't necessarily understand how an input yields an output. And they're fundamentally non robust, which is to say that if you have access to the model and the underlying model weights, you can modify that model for specific purposes or specific tasks. That's a good thing. You can talk more about that, but you can also unwind refusal behaviors and you can direct those behaviors in a more malicious direction.
So when I, you know, for, for me, my first sort of introduction to the changing phase of that debate was, was when I led public policy for one model developer stable, Stability AI, which developed Stable Diffusion. Stable Diffusion was an image generating model, very popular. At one point, it, it amounted to maybe 80% of all AI generated imagery.
But when the very first iteration of that model went out into the ether on GitHub and on Hugging Face, you had members of Congress writing to the then national security advisor saying, this model is a threat to national security and public safety. You need to use your export control authority to prevent the distribution of these models.
And so, so there was, there was a kind of a moment around the time of Stable Diffusion coming out and and ChatGPT rising to prominence where policy makers started to think about this in the context of models.
Kevin Frazier: So pause there for, for a quick second. Fortunately of the, the family members, I've been able to cajole to listen to this podcast, my dad's among them, and he's about as technical as a nail which is to say not very technical.
So what is the technical basis for these national security concerns? So you, you mentioned that even something like Stable Diffusion, which you're not immediately think, oh no, people can generate images of pigs flying over the moon, watch out national security concern. What, why is that a technically a matter that folks concerned about national security even brought up in these sorts of debates about how to regulate AI?
Ben Brooks: Just to put a finer point on that, I think there isn't really consensus among policymakers about the risks that ought to justify these kind of interventions, right?
So if you think back to the last session of Congress, right, 350 bills on AI. We've had under the Biden administration, the longest executive order in U.S. history on AI. And if you and, and, and you know, 700 and say 50 something bills at a state level. If you unpack all of that, the motivations are very different policymaking to policymaker.
So I think if you talk to national security folks in the Biden and the Trump administration, the concern is fundamentally about CBRN and cyber risks. So chemical, biological, radiological, nuclear, weaponization risks. Could AI, could these models be used to accelerate the production of a, a catastrophic weapon, a weapon of mass destruction? Could they be used to accelerate an offensive cyber attack at massive scale? Those are fundamentally the bread and public concerns of the, the executive national security community.
Kevin Frazier: And the concern there in particular for open source is that—as opposed to a closed model where we don't have the weights made publicly available—in open source model—and here, correct me if I'm wrong—we're going to have higher odds of, let's say bad actors and in particular, non-state bad actors who are even harder to monitor and police. That's the, the grave concern, I'm guessing with respect to open source.
Ben Brooks: That that's right, that's right. If you have, if you have access to the models parameters, three risks become more prominent, right. One is misuse. You can, you can integrate that model into your own applications. You can deploy those applications and the upstream developer has very little visibility or control over what they're doing.
You also raise the prospect of modification. So someone can take a capable base model, they can modify that model through fine tuning, reinforcement, learning, again integration with other systems and other tools. And that modification can expose undesirable or unsafe capabilities that may not have been in the model off the shelf when it was first released on GitHub or Hugging Face, but can be exposed through that modification.
And then I suppose the, the third, and, and you know, equally important point is this prospect of a mishap, right? Maybe, maybe the model has some capability or some affordance, it can be used in a certain way that wasn't clear at the time it was released. Goes out there into the ether. And once people start using the model in that way, or once the model's behaviors become apparent, it's too late to do anything about it. You can't withdraw that model very easily. It's a digital file that's being downloaded by millions of people around the world.
So, so that was fundamentally the concern for, for those national security folks. It's the same in in the online safety space as well, which was really what animated concerns about image, video, and voice generation. Is the concern, not that you're going to create a weapon mass destruction, but that you'll be able to create compelling deep fakes, and you use those deep fakes for abusive, fraudulent, or politically misleading and deceptive purposes.
You think about the, the Biden robocall, right? So there was this scare around, you know, image, video and voice models being used to throw the U.S., EU, and Indian elections. And, and fundamentally for a lot of po, particularly for a lot of legislators, that is their biggest concern. They're not so concerned about CBRN and cyber risk. They're concerned about these more quotidian online safety risks.
And I think that’s part of the challenge. It’s that what motivates these debates and these concerns changes depending on who you're talking to and changes month to month. I think if you rewound three months, the national security conversation around open weight models and AI in general was catastrophic risk, CBRN, and cyber risk, predominantly by non-state actors to your point, Kevin.
And you fast forward to post DeepSeek R1, and the consensus just China, China, China, right. It's, it's, it's strategic competition. It's, it's misuse by state actors. And, and I think we should pay attention, why, why is the, this sort of unevenness in, in what motivates these, these objections.
Kevin Frazier: Yeah. So I, I love for you to, to walk through three, what I'll call kind of critical points or junctures in this debate.
So early on conversations around Llama, Llama is Meta's open source model. And there were a lot of folks who were saying, oh my gosh, you know, Meta you are basically facilitating Chinese advancement. You're making it easier for Chinese labs to keep pace with leading AI companies. And there was a lot of heat, I think, on Meta. So we, we had that paradigm, which I'd love to start with.
Then as you mentioned, we had this DeepSeek moment, so I'd love to analyze what does that mean for open source.
And now the third point I want to talk about is suddenly, famously OpenAI was kind of the, the, the champions of closed models. I'll just, I'm sure they wouldn't describe themselves as such, but I'm, I'm giving them a new trophy: the champions of the closed model. All the sudden Sam Altman saying, Hey, watch out. We are going to be releasing not only an open weight model soon, but you can expect us to continue to kind of balance open models and closed models going forward.
So let's start with that Llama period and the kind of Meta hate, I'll say, for being so, such fervent champions of open source. What was that period like? What were the defining attributes of that initial debate over, oh my gosh, anyone who's doing open source hates America and loves our adversaries.
Ben Brooks: Yeah. So I think the, the initial release of Llama stood for just one proposition, right? Which is, wow, there is someone out there who is willing to release a capable and expensive model openly.
The challenge is that open source already had a bunch of headwinds, right? It's, it's a distributed community. It's kind of difficult to mobilize. It can be misused and modified in all the ways that we've discussed. There was already, I think, a very low awareness among policy makers, particularly legislators, less so the administration.
But kind of low awareness about the importance of open source, the open source. Sits in all our data centers. It powers most of the world's smartphones. It's in flight control systems. For our rockets, it's, it's on our nuclear submarines, right? Open source is good because it can be inspected, it can be modified, and it can be secured.
So there were already these headwinds. And then the first really big player to come out there with a really good frontier or semi frontier model happened today, one of the least trusted companies in America, you know, Meta. And, and I think they're probably quite open about this, had a really challenging reputation at that time in the, in the Biden administration.
And so for them to come out with this fairly provocative release, raised eyebrows. And, and there was, you know, pretty swiftly a, a sort of angry letter, a bipartisan letter from senators, Senators Hawley and Blumenthal saying, you know what, what are you doing? We, we, we get that open source is important, but what you're doing is reckless. There doesn't appear to be any sort of systematic process in place to evaluate risks, mitigate those risks before release. What do you have to say about that?
But, but broadly speaking, I think that was, that was it. It was finally this watershed moment where you say someone is willing to spend tens or hundreds of millions of dollars releasing the underlying weights for these models. My view is that is that is predominantly a very good thing.
I, I think, you know, we had up to that point and right through to the present day, a bunch of people telling us this is, this is, this is revolutionary technology. It's gonna transform the economy and the idea that three or four Bay Area companies should be pay walling this transformative technology and that we shouldn't have a capable, open alternative alongside those models. That's a, that's a very scary world. Right? That's a, a concentration of power and control in the digital economy and the real economy that we, we, we hadn't seen for a very long time.
So I think, I think the Llama release showed that there was a fork in the road and that there are good open alternatives in parallel.
Kevin Frazier: Yeah. And I, I think what was fascinating too about that moment was somewhat of a failure to include a more robust analysis of the economics of open sourcing a model.
So Meta from a a, a strategic economic perspective, there are a lot of incentives that come with open sourcing a model. If you are the model that is the, the most common one, the most ubiquitous, getting more data, getting more uptake, getting more user adoption, all of those things can be incredibly valuable, especially given that we know having access to data and quality data is so essential for improving these models.
So we can talk more about those economics in a second. But it is fascinating to see that this initial period of the open source debate was very much a, do you love America or do you wanna help our adversaries kind of black and white conversation.
And then DeepSeek happened. Why was that so important in disrupting this conversation about the pros and cons and adding some nuance to this open source debate?
Ben Brooks: Yeah, I mean, there, there's so much to say about DeepSeek in general. But I think if you, if you really focus on the material facts for policy makers, the, the DeepSeek R1 release kind of showed three things.
One, you can get a lot of performance and a lot of efficiency through, you know, a series of familiar innovations in the training and development pipeline. Right. So, you know, and we can go into greater detail, but the, the R1 model and the V1, sort of base model that DeepSeek released a couple of months prior.
You know, it brought together a, a, a bunch of techniques that had been pioneered by different researchers and different companies earlier. But they, they assembled it into a really interesting pipeline, mixture of expert architecture, reinforcement learning, you know, so that the model learns to reason, you know, the model learns to explain its reasoning through chain of thought, a number of techniques to improve efficiency in, in inference.
And, and, and they yielded, you know, this, this model that was, you know, 671 billion parameters, only a small number of those 30 something billion were active at any one time. And this model was yielding, you know, state of the art or near state of the art performance on certain benchmarks.
So for policy makers, the question there is like, wow. how much of a moat does U.S. industry actually have? Right? May, maybe the moat isn't even compute, maybe it isn't even money. They did this, you know, allegedly with, with $6 million or at least a marginal cost of $6 million. So what does that mean for, for national security policy and industrial policy going forward? How much of these sort of breakthroughs are going to come through your efficiency and through familiar techniques and familiar innovations.
I think the second, the second piece was the possibility that DeepSeek developed this largely independently of export controlled U.S. hardware, technology, and infrastructure. So, DeepSeek says that they developed this model with 2000 H800 chips. At the time that they procured those chips, they were not export controlled. They were subsequently export controlled by the Biden administration.
And as I said, DeepSeek, you know, maintains that they, they spent, you know, just under $6 million on the development of, of these models. So if we take them at face value, the question then for policy makers is, you know, to what extent are export controls on hardware and then chips an effective choke point. Like, will that, will that work going forward?
And there's a lot of pushback on that, right? Like there's, there's, you know, Anthropic, Musk, and, and others have, have come out quite strongly. SemiAnalysis has some, some great material on this to the effect that, that, hey, they actually had tens of thousands of, of A100, H100, H20 chips. Some of which they may have obtained legitimately, others may be less legitimately. And, and their overhead cost, their total expenditure would've been much higher than $6 million.
But, but that's sort of a big, a big wake up moment for policy makers.
Kevin Frazier: How much of it was a concern as well that we've seen China in other contexts kind of dominate new technological paradigms and dominate new efforts at expanding its sphere of influence by being the sort of core infrastructure upon which other countries build?
So when you think about the ports it's helping build in Africa, when you help, think about the roads it's building in Southeast Asia, so on and so forth. Can we think of their leaning into open source as a sort of means of becoming the default AI of the world? Is that a concern that may be animating folks to, to also adjust their perspective on open source domestically
Ben Brooks: Yeah. Com, completely right. So I think, I think really the third and, and maybe biggest bucket of concerns for policy makers was this, this point that maybe China is going to use open weight models to essentially ship their technology, their intangible technology around the world and create dependencies on Chinese industry.
That's one thing that's, that's important for strategic competition, but the challenge is if these models have embedded vulnerabilities, if famously these models are, are censored on, on questions pertaining to the Chinese Communist Party and the, on Beijing's regulations, then what, what does that mean for the future of, of the, the digital economy?
Right? Like the example I give is, is imagine if outside of the U.S. and Europe, the next default search engine isn't Google and it isn't Complexity. It is some app that is powered by a model developed by Idu or by Alibaba and, and Tencent and, and DeepSeek, right? A model that can't answer questions about Taiwan and Uyghurs and, and, and maybe has all sorts of other embedded vulnerability. Some of the more obvious, some of them less obvious. But that's, that's the kind of concern that I think policy makers ought to have.
Now, the, the question then is, well, what do you do about that? So within a couple of days, 48 hours of DeepSeek R1 being released, you had the House Select Committee on the Chinese Communist Party coming out and saying, this censored open weight model again is a threat to national security. You had Senator Hawley in the Senate actually drafted a bill that would expand export controls in a way that essentially criminalizes someone uploading an open weight model if it were available to a national in China, would criminalize someone downloading, importing, intangible AI technology from China, something like DeepSeek, the DeepSeek model.
And would prohibit. Research collaboration with Chinese nationals, which is to say that a machine learning researcher would not be able to go to Europe's ICNL and, and, and share what they've worked on the previous year. Right? So, so that's sort of the extreme hawkish version of the response.
I think the better response is to say, hey, we see what's happening here. Open weight models is not only a way to potentially accelerate adoption in AI across our economy. But it is also a way to create dependencies on U.S. industry and to make sure that U.S. trained, U.S. regulated, US aligned models, end up powering the world's AI applications.
And that's why I think I, I think the worst response to, to the DeepSeek and just to say we should stop releasing capable open models. I think China is starting to understand that this, to your point about belt and road, right? This is a way to project soft power abroad. And if the U.S. withdraws, if it, if it pulls up the drawbridge, if it tries to, to cut off the flow of this open technology through either through enhanced chip controls or through controls on intangible technology, then there's a vacuum there and, and China will decouple and other jurisdictions will start to fill that, that vacuum.
Kevin Frazier: And so now fast forwarding to present day or near present day, we're talking in early April, and as you mentioned, it sounds like folks con, con, congratulations, Ben, are heeding your advice and saying the best response isn't to, or at least among industry folks, the best response isn't to quash open source models, but instead to lean into it.
So as I hinted at OpenAI, which famously has avoided releasing an open weight model for a long time. Sam Altman has now announced a sort of pivot of the company policy. What does this pivot mean for the development of AI in the U.S.? Should we expect that open source may become the sort of new model?
And if that's the case, one concern I have, you mentioned earlier about, the issue with respect to concentration of AI labs, where if it is just a handful of companies in Silicon Valley leading this AI effort. We've seen this playbook before, right? Let's just watch The Social Network again and see the, the faults that manifested. What does this mean for the economics and larger AI ecosystem if open source starts to become the new default, or at least a greater mix of this AI portfolio going forward?
Ben Brooks: Part of what maybe motivated the announcement is a growing awareness that the models, the, the model layer is becoming commoditized, right? But a lot of these breakthroughs aren't replicable fundamentally they can all be traced back to open research. And given enough compute and enough dollars, you know, maybe other teams are gonna be able to yield the same performance in, in, in a relatively short period of time.
So the question then becomes, well, how do these firms that have, you know, spent vast, vast sums of money on, on training and development, how do they, how do they monetize this space? How do they create a mote? And I think that is increasingly gonna focus on the product layer, on the application layer. So it is not just the raw knowledge as the dense knowledge digest. It's how you integrate the model with other tools and other systems, and then how you productize that and get that out there into the real world and into the hands of, of deployers who can use it and are willing pay for it.
So I think to some extent that may have motivated the decision. Look, I think, I think broadly speaking, it's, it's hugely promising. I think that's what they've said is exactly the right approach, which is it's not open or closed. It's open and closed, and they both have a role to play. I think their commitment to evaluating for risk prior to release is, is expected. It's fantastic.
I, I think they will potentially help to, to really advance the state of the art in terms of evaluating open weight models, not just for off the shelf risk, but also for modification risk. You know, in other words, what's the worst you could possibly do to this model through optimization and modification.
But there is this kind of lingering reservation I have about the announcement, right? Which is that a lot of the signaling around that announcement, and what OpenAI and Anthropic and others have previously said to the admin, the Biden administration and potentially the Trump administration is open sourcing is okay so long as it's slow or small, right?
By which I mean so long as it's some way behind the frontier or it's so small and its capabilities are so diminished that we don't really need to care about it. And that is what I sort of described as the poor cousin theory of open source. Right? And I think it's really troubling, right?
It's troubling because you know, on the one hand it's deferring difficult questions to another day, right? Difficult questions around like, what risks do we actually care about when it comes to models? Who determines acceptable risk, developers or regulators, or is it the court? What is the standard of care for mitigation before you send these models out into the world and how to developers satisfy that standard of care?
You know these tough conversations that we've been dancing around for years now. And, and I fear that that kind of, you know, open source, the small stuff, open source, the sub frontier stuff is just delaying that.
And then I think the other one is, it kind of, you know, it, it, it overlooks the fact that to really benefit from capable AI, it's not enough to just contain it behind a paywall, right? Like safe containment doesn't really turbocharge the U.S. economy. It doesn't give the U.S. a kind of strategic boost over its adversaries.
What will do that is like Safe Diffusion. It's like getting good, capable models in the hands of as many deployers across the economy as possible. Helping them to evaluate your risk, helping them to modify it for specific tasks and helping them to integrate it safely into what they do. And so I, I do worry that, that these announcements may end up just being, you know, we're open sourcing some small stuff.
We're open sourcing some stuff that's a year behind the frontier. But the, the capable stuff, the economically transformative technology, we're going to continue to keep behind the paywall and it ought to stay there. And, and that's, that's a position that I, I worry about.
Kevin Frazier: Yeah, it's interesting from a history of technological diffusion standpoint, right? If you could imagine, for example, instead of the rural electrification administration making full electricity available to farms, for example, they said, ah, you only, you're, you're throttled at, I don't know, I'm not electrician, 30 volts, right? You get to, you get to light up your barn, but you can't light up your bedroom or whatever. That's only for the urban city dwellers.
Seeing that kind of limited capacity, I think would, if I, if I'm understanding your point correctly, kind of undermine the whole point of open sourcing, which is allowing for broad analysis of what are the capabilities of these models, what are the risks, what are the potential benefits?
And if you're just handing out last year's tech, well then the, the, the possibility of discovering those new risks or those new benefits will be greatly diminished because you're just kicking the tires of, you know, a 2004 Sonata instead of looking at, you know, a Cybertruck and, and what are the, the potential pros and cons there? Is that somewhat of, of a way of understanding it?
Ben Brooks: Yeah. Yeah. It's, it's, it's, it's, it's really just saying that, look, I, I think 99% of deployers across the economy, whether they're, you know, individual workers, consumers, creators, or whether they're large enterprises, they're not gonna need to fold proteins.
They don't really, they won't necessarily need the tip of the spear state of the art frontier model, but they will need something that can do economically useful tasks and do them well, and do them cheaply and ideally be capable of being scrutinized, can be modified and can be implemented in a secure, private environment, which is kind of what open weight models offer.
Now, if you make those capable models openly available, I think that's the fastest path to diffusing this useful technology across the economy in ways that ultimately impact productivity and innovation.
I think if we take the view, especially if regulators and legislators take the view that capable models should not be openly available. Then we don't, we don't obtain the, the economic benefit of this technology. Or at the very least, we create these, these highly concentrated dependencies on a handful of firms for critical technology.
And, and that isn't good for, for all the usual reasons. It's not good, right, like for introduces risk, it means that people are transmitting sensitive data back and forth with two or three APIs for the rest of eternity. It means we have very little visibility into the behavior and the performance of these models and very little opportunity to modify and do something about it. So, so I think that, that, that is, that is challenging.
I mean, you, you also mentioned this, this, this wider question around, you know, open or closed. Are we heading for a world where the, again, the whole community, the whole ecosystem relies on a handful of companies like Open AI and Anthropic or Meta. And I think it’s a really interesting question right. I don’t think network effects are gonna play out in AI quite the same way that they played out in the internet, for example, right?
Like fundamentally, the platforms, the big internet platform, search and social media became big and stayed big because you know, their value increases with every additional use of plugin into the network. With AI, especially with models, you know, the calculus is a little bit different. I mean, essentially you've got just a huge publicly available, we can debate that, but publicly available dataset on the internet. You've got very large, very expensive models being trained with huge amounts of compute. The only companies or organizations that can field that capital at the moment, yes, happen to be the companies that did very, very well off the internet economy and amass that capital through things like ad revenue.
But once they've released that model, you know, especially like once Meta released Llama4, for example, that model's out there in the ether. And sure. They, they qualify how you can use it and how you can deploy it and things like that. But, but once the model's out there, I mean, I mean anyone can, can start to play around with it and integrate it into, into their own systems.
So, you know, I think, I think there is still that, that concentration risk, but it's a concentration risk that comes from the inherent cost and compute intensiveness of this research and not because of some network effects in the, in the sort of internet sense.
Kevin Frazier: Yeah. It's super fascinating because I think that for the AI labs themselves, they have the a, a sort of similar economic incentive as a social media platforms, which is to say the more users you have, the more data you have. So in that sense, there's, there's a network effects component for them.
But for a user, it doesn't, I, I don't care what model you, Ben use, I don't care what model my Lawfare colleague Alan Rozenshtein uses, from the sense that it doesn't give me any additional benefit of getting onto ChatGPT and saying, Alan just looked up a fun healthcare regime, or something like that, that doesn't add any benefit to me. I'm not friending Alan's post or something like that. So it is interesting to see how there are parallels to social media, but it is a distinct set of questions we have to grapple with.
And with that in mind, I'm curious, let's just say Senator Hawley calls into the pod, Senator Hawley, you're welcome anytime if you're, if you're listening to this. And says, Ben, you know, I agree. I don't want a. Chinese open source model to be the default model for the rest of the world, but these CBRN issues, these cyber concerns, these nuclear concerns that I just can't shake 'em.
Is there a sort of middle ground policy you'd recommend for the people who are just really scared of open weight models leading to this huge increase in the risk of bad actors deploying biological weapons, causing havoc? What's a policy that we can latch onto? What would you like to see, for example in the AI action plan with respect to open source policy, both in terms of promoting its benefits and recognizing some valid concerns?
Ben Brooks: I mean, I would love to see a clear recognition that safe diffusion is how we're going to boost productivity and innovation and quote unquote win in AI.
And so what does that mean in practice? It means a few things. It means one restrictions on useful, capable intangible technology should be a last resort, not a first resort. I don't take the position that we should open source everything for all time as sort of absolutist, but it should be a last resort. And too often these restrictions have been talked about as a first resort.
There is lots of low hanging fruit that we can grapple with before then. Right? Like the regulation of transparency in model development, the regulation of deployers users, downstream, platforms. There's a lot of work to be done there, and we haven't even done a gap analysis to determine where does our existing regulatory and legislative infrastructure fall short.
In fact, one of the only governments to do this in any systematic way was the previous UK government. And they went around, they asked for the regulatory agencies, do you feel like you have the statutory authority and the resources to deal with emerging risks in your domain? And of the 12 or 13, all but one said yes. I think the same you'll find is, you know, the FTC and, and CFPB and others said the same under the Biden administration. So I think, you know, treat model layer intervention as a last resort, not a first resort.
Build up readiness on the assumption of openness, right? So that can mean everything from defensive accelerationism, you know, build up the kind of ecosystem downstream mitigations and safeguards. And there's a lot more work that can be done there, including with, with federal support.
And then the flip side of all of this is we need a good monitoring capability in government. We don't have a monitoring capability. That's when we start to see really reactive regulation and legislation. And that's why things like the U.S. AI Safety Institute is so important cause that is the monitoring capability for the U.S. government who can monitor for trends of, of, you know, bipartisan interests and bipartisan significance. They can identify, you know, possible, proportionate forms of mitigation and they can give advice to the administration and, and potentially to legislators as well.
So, you know, I get concerned that on the one hand there are still numberings, including this administration around going harder on model layer interventions like intangible technology controls. While on the other hand, it's still very uncertain what's going to happen to the U.S. AI Safety Institute. We need that monitoring capability if we're going to preserve a maximally open 5egulatory environment. So that's what I'd say as to Senator Hawley.
But I think a lot of this is gonna happen frankly, at a, at a, at a state level. I mean, the, the first, what, what, what is it now, April, right? The first quarter of this year, we've seen as many, more state bills than we saw in the whole of the last two year legislative session. And, and there's, there's a lot happening there. And, and a lot of that could affect open sourcing and open weight in really subtle, but really significant ways.
Kevin Frazier: Yeah, and I love the sort of chicken and egg issue you're pointing out here, which is the longer we take to just set up a baseline approach to transparency and monitoring, the greater the odds of reactive legislation that really clamps down on open source because it's just that dearth of understanding that you've pointed out at the state level, at the federal level.
If all we're doing is just legislating in response to whatever China does, that's not exactly a, a great policy posture to have. And ideally, we would have a clear national vision for how we want open source to fit into our broader AI portfolio. But that's all-
Ben Brooks: Yeah. Yeah. And, and, and I mean with, with, with respect to, to the very thoughtful legislators and policy makers who, who are coming up with many of these proposals, some of the most dramatic and interventionist proposals have been ones that were, were kind of framed reactively in response to some media moment, right?
If you think about the, you know, there were at least two developer licensing frameworks that were, that were drafted in the Senate, bipartisan frameworks that were drafted in the Senate in the last session. There was a House bill that would expand export controls to model weights. There was, of course, the Hawley bill that I mentioned earlier on, on DeepSeek.
And many of these kind of pop out in response to the release of Llama, or in response to the release of DeepSeek. And I think we, we can do more to make sure that these are calibrated and proportionate.
I think we all forget that the Biden administration. It was, it was the longest executive order in U.S. history, but there wasn't much in there that was actually regulatory in nature. And in fact, if you're a developer, there was really only one obligation. Which was, if you are training a model over 10 to the 26 flock, you need to report to the federal government Department of Commerce on your red teaming results. The government didn't even tell them what red teaming to perform. They just said, you report it so we know what's over the horizon.
And I think in the scheme of things, those frameworks, I would put it the less problematic end of the spectrum, but where you start to see export control regime for multiple weights. Licensing requirements for developers. Or state legislation that modifies liability rules in ways that are fundamentally incompatible with open sourcing. If they require a level of visibility, control, and custody over models that is, is infeasible in an open source environment.
Those are the kind of interventions that are really going to impede open innovation by, by which I very specifically mean, meeting good, capable technology openly available for third parties to inspect, inspect to modify and to deploy independently.
Kevin Frazier: Well, Ben, this will not be the last news moment or media moment regarding open sourcing, so we'll be sure to have you back at some point down the road, but we'll have to leave it there for now. Thanks again for coming on.
Ben Brooks: Thanks, Kevin. Appreciate it.
Kevin Frazier: The Lawfare Podcast is produced in cooperation with the Brookings Institution. You can get ad free versions of this and other Lawfare podcasts by becoming a Lawfare material supporter at our website, lawfaremedia.org/support. You'll also get access to special events and other content available only to our supporters.
Please rate and review us wherever you get your podcasts. Look for our other podcasts, including Rational Security, Allies, the Aftermath, and Escalation, our latest Lawfare Presents podcast series about the war in Ukraine. Check out our written work lawfaremedia.org. The podcast is edited by Jen Patja. Our theme song is from Alibi Music. As always, thank you for listening.