Random Toxicity? What’s Going on in @benjaminwittes’s Mentions
An attempt to understand the hostile, and strangely repetitive, responses to @benjaminwittes tweets and to demystify some bizarre pile-ons it and other accounts provoke.
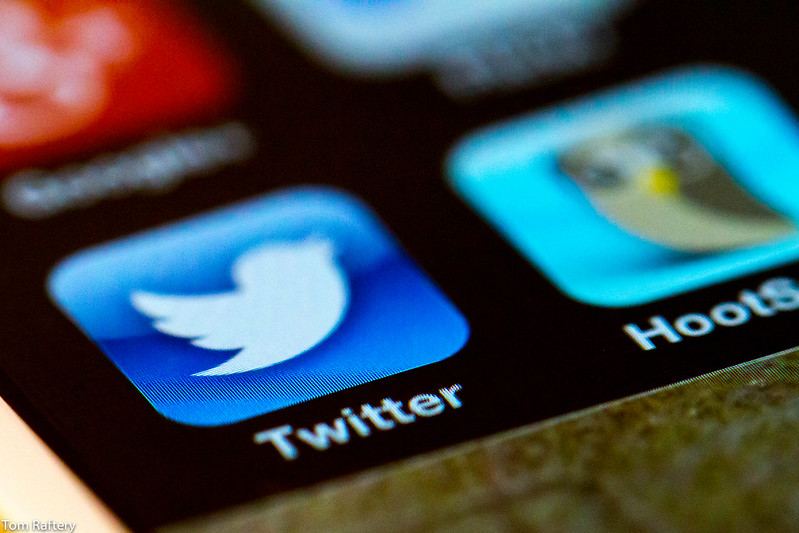
Published by The Lawfare Institute
in Cooperation With
Over the past few weeks, I have developed—in cooperation with several other people—a collection of interesting data about the way people react to me on Twitter. I did not do this because I am self-involved but because for the past year and a half, the reaction to me on Twitter has been ringing alarm bells about disinformation, and the more recent reaction to others has heightened concerns. I don’t pretend to know what this data really suggests. I don’t know how much of the pattern I describe below is automated. I am not making any allegations against anyone. I’m putting the following out in public as a preliminary indication that something weird is going on that warrants examination—examination I am not qualified to do.
(This story, for reasons that will become clear, needs to be told in my voice—that of Benjamin Wittes—but much of the analysis below was conducted by Jacob Schulz; hence the joint byline combined, somewhat awkwardly, with an article written in the first person singular. It also draws significantly on work done by Christopher Bouzy, creator of the Bot Sentinel tool.)
My interest in the Twitter reaction to me began shortly after Brett Kavanaugh was confirmed to the Supreme Court in 2018.
I had supported Kavanaugh’s nomination early on, said nice things about him and defended him against allegations I thought were spurious. But though I publicly changed my position after Christine Blasey Ford came forward and testified, and I wrote a lengthy article opposing the nomination, a strange thing happened: Almost whenever I tweeted, and almost no matter on what subject, large numbers of people would remind me that I had supported Kavanaugh. Very large numbers of people.
And strangely, they seemed all too frequently to be using the exact same lingo. I was Kavanaugh’s “buddy,” I was reminded. I had “vouched for Kavanaugh,” people told me—over and over again. Around the same time, I noticed as well that I was Jim Comey’s “BFF.” Always BFF.
Then came the Bill Barr nomination. My support was far less energetic than my initial support for Kavanaugh; it was always tepid. I regarded his nomination as a calculated risk, and I wrote about the pros and cons of his nomination with relatively open eyes—though not open enough, as it turned out. While warning about the dangers he posed, I—like a lot of commentators—regarded him as an institutionalist, and I was complimentary about his intellect and past service. I certainly preferred him to the acting attorney general, Matthew Whitaker. And I argued that we should wait to condemn Barr’s handling of the Mueller report until we saw what he did with it. I ate a hot steaming plate of crow a few weeks later.
Yet again, my Twitter mentions were full of reminders—in remarkably consistent verbiage. This actually continues to this day. I “vouched for Bill Barr,” I was told recently in response to a tweet about a possible legal humor podcast. Another tweeter told me my expression of dismay at Ken Starr’s recent behavior was what he would expect from someone who “vouched for William Barr.”
I relatively quickly began wondering why the language was always so similar. Why was it always “buddy” with Kavanaugh? And why the specific phrase “vouched for” with respect to both Barr and Kavanaugh?
It isn’t exactly disinformation. After all, it was true—if wildly simplistic—that I had vouched for Kavanaugh and Barr. And Comey and I are, indeed, friends. So a barrage of reminders was not exactly an effort to spread false information about me; rather, a large number of accounts were simply responding to whatever I said with a simple and crude effort to caricature me based on endless repetition of the same simplistic themes. Where was this coming from?
A while back, I asked a friend who specializes in disinformation to look into the question. She examined the matter and reported back to me later that too much time had passed to make any determinations. Many of the tweets were no longer around, and many of the accounts were gone too. Indeed, looking back using Twitter’s search functions, I have been unable to find anything like the volume of material that used to be there. So I dropped it.
Then Lisa Page showed up on Twitter.
The reactions to Page’s tweets were astonishingly vile: misogynistic, hateful, demeaning. As with the earlier responses to me, a huge number of responses to Page were saying the exact same thing. The word “homewrecker” and “slut” appeared with odd regularity. The specific phrase “Ok, homewrecker” showed up with a frequency that could not be due to chance. What was different in the responses to Page was the volume, which was immense. And this time, the accounts in question were still live. So I started examining them without waiting many months.
In the intervening months, I had also become aware of Bot Sentinel, a platform that classifies Twitter accounts based on their tendency to engage in misleading and coordinated behavior—in other words, their penchant to act like a troll on Twitter. Bot Sentinel operates both as a browser extension that embeds in one’s Twitter feed to flag “untrustworthy” accounts and as a stand-alone website in which one can plug in an individual account to see how the service classifies it.
The service does not provide a binary bot-or-not determination, but instead offers a probabilistic “trollbot score.” Using a machine-learning algorithm to analyze an account’s political Twitter activity, Bot Sentinel produces a score that reflects the probability that an account is a “trollbot”—a user who participates in “coordinated harassment campaign[s],” retweets known misinformation or otherwise regularly engages in “repetitive bot-like activity.” Bot Sentinel describes the calculus as fairly straightforward: “The more you exhibit irregular tweet activity, the higher your trollbot score will be.”
The platform uses a tiered system to classify users based on their trollbot scores. Users are identified as normal (0-24 percent), moderate (25-49 percent), problematic (50-74) or alarming (75-100). Bot Sentinel reserves the “alarming” designation only for the most offending accounts. On average, less than 15 percent of the accounts the platform analyzes in a given 24-hour period meet the 75 percent threshold, according to Bot Sentinel. Though some particularly zealous human trolls can achieve a trollbot rating of 75, “those accounts that exceed 75 percent are largely inauthentic,” says Bouzy.
The service is, of course, not perfect and has faced criticism from some researchers for its imperfections. It does label some accounts run by normal human users as trollbots. These aren’t false positives per se; Bot Sentinel does not claim that trollbots are necessarily automated users. But this is a major drawback for researchers chiefly interested in reliably identifying and tracking automated accounts, rather than looking for swarms of harassing or misleading posts.
Disinformation specialist Renee DiResta explained the disadvantages of services like Bot Sentinel in an email:
People are interested in understanding who or what they’re talking to on Twitter, particularly around political hot topics. There’s not much visibility into how accounts are behaving just by looking at their tweets, and anything that helps people feel more informed is filling that need. The problem is that sometimes certain “botlike” behaviors like tons of retweeting, incredibly high posting volume, hashtagging spamming - things that we do see from automated accounts - are also used by real people in “followback trains.” So while these tools are filling that need it’s also important to understand their limitations.
“Trollbot” designations understandably infuriate human users who get tagged with them, and the most vocal of these displeased users tend to be those who feel that Bot Sentinel contributes to the “dehumanizing [of] anybody who is conservative.” The propensity of Bot Sentinel to slap the trollbot tag on normal humans aboard the Trump train creates problems for researchers. In general, bot-identification services do have a bad track record of misidentifying conservatives tweeters as bots, a fact that makes disinformation researchers understandably cautious about using them as tools.
And Bot Sentinel doesn’t operate with a level of transparency that could entirely assuage these concerns. It operates, rather, as something of a black box; it offers an FAQ page but not a view inside its algorithm. Other services are more forthcoming about their inner workings. The Indiana University researchers who run Botometer, for example, publish academic papers that offer a candid look at their tool’s methodology.
That said, Bot Sentinel’s performance—at least in my view—inspires more confidence than its publicly available competitors. Botometer, unlike Bot Sentinel, offers an assessment of the likelihood an account is automated, but it sometimes struggles to identify clearly automated accounts. It assigns accounts a Complete Automation Probability (CAP) score that the IU researchers claim “is the probability, according to our models, that this account is completely automated, i.e., a bot.” What does it rate, for example, the Lawfare Podcast (@lawfarepodcast) account, a Twitter feed that automatically tweets out links to our podcasts as they are uploaded? It gets a 2 percent CAP score. The Big Ben Clock account, which tweets out the time on the hour by stringing together the syllable “BONG” (11:00 is “BONG BONG BONG BONG BONG BONG BONG BONG BONG BONG BONG”) receives a 0 percent CAP score.
BONG BONG BONG BONG BONG BONG BONG BONG BONG BONG BONG
— Big Ben (@big_ben_clock) February 22, 2020
Another bot detector, TweetBotOrNot, seems based on our tests a bit more accurate at pinning bots than Botometer. It claims (sensibly) that there is a probability of .937 that @big_ben_clock is a bot. But it is glitchy too, and it produced an error message just as frequently as it produced a bot probability in our testing.
So while Bot Sentinel is not without flaws and, critically, while it should not be taken as a firm measure of automation, it is useful and is more compelling than its competitors. Specifically, it should be taken as measuring the propensity of a Twitter account to act in a repetitive and abusive fashion. Bot Sentinel helps to illustrate which tweets and accounts demonstrate “alarming[ly]” repetitive behavior. It helps illustrate whether nasty replies are organic gripes or the result of systematic targeting from users who—whether automated or not—make it their vocation to harass and swarm in coordinated barrages of nearly identically worded abuse.
So with the understanding that Bot Sentinel is just one tool and not perfect, I recently began using the service to understand the responses provoked by a given tweet and to contextualize repetitive, abusive behavior on Twitter. What happens when you use Bot Sentinel to try to demystify the bizarre pile-ons in response to my and particularly Page’s tweets? It turns out that the dozens of users who think up the same oddly-specific trope to drop in my replies tend to share “problematic” or “alarming” ratings.
As a baseline for comparison, I asked my Twitter followers to reply to a tweet of mine with a screenshot of their own Bot Sentinel trollbot scores. The idea was to see what normal humans who follow the @benjaminwittes Twitter feed look like when you run them through Bot Sentinel. Rather to my surprise, I received more than 250 submissions.
So here's another experiment in disinformation. In replies to this tweet, post a screenshot of your own @BotSentinel trollbot rating—particularly if you are someone who posts combative stuff on Twitter of a political nature, particularly if you are someone I know.
— Benjamin Wittes (@benjaminwittes) January 19, 2020
At the time, my trollbot score was 0 percent, though it has since risen to 5 percent:
I am not a shrinking violet on Twitter. I say what I think. I argue with people. I retweet a lot of stuff. Here's my trollbot rating from @BotSentinel. pic.twitter.com/nSaOHL4xdT
— Benjamin Wittes (@benjaminwittes) January 19, 2020
And it turns out that the people who follow me don’t rank highly on the trollbot measure either. The mean score of the accounts that replied was 22, with a median score of 20—both within the “normal” range on Bot Sentinel’s scale. Only 5 percent of those who replied landed a “problematic” designation. Exactly zero of the accounts achieved an “alarming” rating. Nobody’s trollbot score exceeded 63.
This is, of course, not a scientific sample. Basic human shame might well be impeding all the real people with “alarming” scores who follow me from posting their scores; in addition, perhaps the sort of person who generates “alarming” scores is too busy abusing others online to even respond to such a request. So I don’t want to suggest that no real people who follow me generate “alarming” measures. That said, the sample is sufficiently large and sufficiently diverse—and I know enough of the people in question—to give an at-least-impressionistic feel for what people who interact with me on Twitter look like on Bot Sentinel.
The response to this tweet also lends weight, at least in my view, to Bot Sentinel’s claim that a 75 percent rating genuinely flags the accounts least likely to be humans not participating in malicious, coordinated activity. My tweet was designed to attract normal human users, and it imposed a practical obstacle for spammers or inauthentic accounts—almost like a CAPTCHA: A Twitter user had to go through the exercise of screenshotting and posting an image as a reply or recording a score. Bots don’t have the technical capacity to do that, and wholesale human trolls don’t have an interest in displaying their high scores.
The responses to my tweet also correspond roughly with Bot Sentinel’s own baseline, which is largely populated by accounts that people suspect of bad Twitter behavior and decide to test on Bot Sentinel. In a given 24-hour period, 15 percent of the accounts put through Bot Sentinel’s algorithm yield a rating above 75 percent; in my dataset, composed entirely of humans, zero meet that threshold. The high score of 63 and relatively small number of users who are even “problematic” seems to reflect the fact that most normal human users do not have substantial trollbot tendencies. This striking result can’t be chalked up to the fact that those who replied to my tweet are anodyne folks who stay away from political topics. My followers don’t tend to post only about puppies or sports, and the replies to the tweet are littered with handles that make overt political references. I think the baseline established in response to this tweet gives some modest support to the idea that a “problematic” rating from Bot Sentinel should raise red flags about a user and an “alarming” rating reflects genuinely unusual behavior rare in real people not using Twitter to harass others.
Now let’s compare these baseline results to the mass of vitriolic replies to this Jan. 18 tweet from Page:
All I can say is this: I very much look forward to Rod’s deposition. https://t.co/so43a38WBh
— Lisa Page (@NatSecLisa) January 18, 2020
Bot Sentinel’s founder, Christopher Bouzy, analyzed the 3,891 accounts that replied to Page’s tweet and found that 28 percent of them received a trollbot score of 75 or higher. More than one out of every four accounts that replied to Page, in other words, had an “alarming” history of disinformation sharing, participation in coordinated harassment or generally repetitive “bot-like” behavior. What’s more, Bot Sentinel rated 41 percent of the accounts replying to Page’s tweet as “problematic.”
Bouzy described the “large percentage of trollbot-like responses” on Page’s tweet as “exceedingly rare” for a tweet not linked to any exact current event. In other words, among normal human users responding to a @benjaminwittes tweet, only 5 percent of responses were “problematic” and none were “alarming,” whereas in response to Page, fully 69 percent of tweets were posted by accounts Bot Sentinel labeled as either “problematic” or “alarming.”
That is suggestive of something—though I don’t pretend to know what.
Following this finding, I asked Bouzy to review the trollbot data from three recent tweets of mine, embedded below, that attracted a high volume of replies. As expected, the results are nowhere near as dramatic as those for Page’s tweets. But studying the data does tend to validate my year-old suspicion that there was something, well, trollbotty about the replies to my tweets.
It’s a sad day for the institution of the United States Senate, which—with the Chief Justice of the United States presiding—is voting serially to deprive itself of information relevant to a fateful decision.
— Benjamin Wittes (@benjaminwittes) February 1, 2020
Hear no evil.
See no evil.
Speak no truth.
Prediction: The next round of this scandal will involve the president seeking some kind of an investigation of Mitt Romney.
— Benjamin Wittes (@benjaminwittes) February 5, 2020
Today, Mitt Romney truly became Pierre Delecto.
— Benjamin Wittes (@benjaminwittes) February 5, 2020
Congratulations to the people of Utah for electing the Republican senator with more guts and integrity than the rest of his caucus put together.
The users who responded to these tweets had an average trollbot rating of 32 with a median of 30—both of these are a full 10 points higher than their equivalent figures for the baseline tweet. Sixteen percent of these users earned a “problematic” rating, and 1 and a half percent passed the “alarming” threshold. In other words, nearly one out of every five responders to my tweets shares at least a “problematic” resemblance to a trollbot who routinely participates in harassing or coordinated behavior online.
That’s what my replies look like normally, but sometimes—it seems—I have a tweet that really blows up. On Feb. 7, while Bouzy was conducting this analysis, I tweeted a brief expression of sympathy for Lt. Col. Alexander Vindman, whom President Trump dismissed from a position on the National Security Council in retaliation for Vindman’s cooperation with the impeachment inquiry. Then the swarm arrived. A striking number of those replying insisted that LTC Vindman ought to be “court martialed.” Many of the users who responded made the same reference to a specific military prison in northeast Kansas. Bouzy noticed something was awry and analyzed the replies. The mean (and median) responder had a trollbot rating of 50; the average user who replied carried a “problematic” rating. Only 12 percent of those who replied rated as “normal” users. The plurality of users were “problematic,” and 15 percent rated as “alarming.” I still wasn’t competing with Page, but the reaction seemed quite similar to the “vouched for” floods from a year ago.
Thinking of Lt Col Alexander Vindman today. You should be too.
— Benjamin Wittes (@benjaminwittes) February 7, 2020
A look at the specific Twitter activity of the individual accounts responding to my Vindman tweet tends to support the trollbot ratings Bot Sentinel assigned. The users who received the highest trollbot scores often, though not always, targeted lots of different accounts that tweeted about Vindman. Take @shurriep, trollbot score of 91, who responded to my tweet by saying, “He needs to be fired.” I wasn’t the only one who got a response from @shurriep on a tweet about Vindman. This account felt compelled to respond to seven different tweets about Vindman, hitting all sides of the ideological spectrum. On Vindman, she told Rep. Ilhan Omar, “He leaked info outside the NSC you dumb broad. He was your God Oblahblah holdover. He needed to be fired.” The account hit back at former Acting Solicitor General Neal Katyal, saying of Vindmann, “He was a leaker and traitor to his country even before going to the White House. He was reprimanded many times while in the military for talking badly about America to Russians. But yeah by all means bring him back to NSC. You libs are f***ing insane!!!” And the account celebrated with Trump agitator Jack Posobiec:
Hell yeah pic.twitter.com/aToymYfaB2
— Shurrie Pennington (@ShurrieP) February 7, 2020
I do not purport to know who @shurriep is; the account did not respond to a tweet from my co-author inquiring about its tweets. But the account’s Twitter activity certainly reflects a gamified, repetitive attempt to defend the president’s action with respect to Vindman. Its quasi-vocational, political spamming is exactly the type of behavior Bot Sentinel purports to measure.
What to conclude from the broad pattern I’ve described here? I have no idea. Here’s what I do know: People are still reminding me that I vouched for Barr and Kavanaugh with amazing regularity. And when I run their accounts through Bot Sentinel, the scores tend to be pretty low. The apparent effort to flood the zone with that caricature and influence human reaction to me with some group of people has clearly succeeded.