Were Facebook and Twitter Consistent in Labeling Misleading Posts During the 2020 Election?
There's room to improve in the upcoming midterm elections.
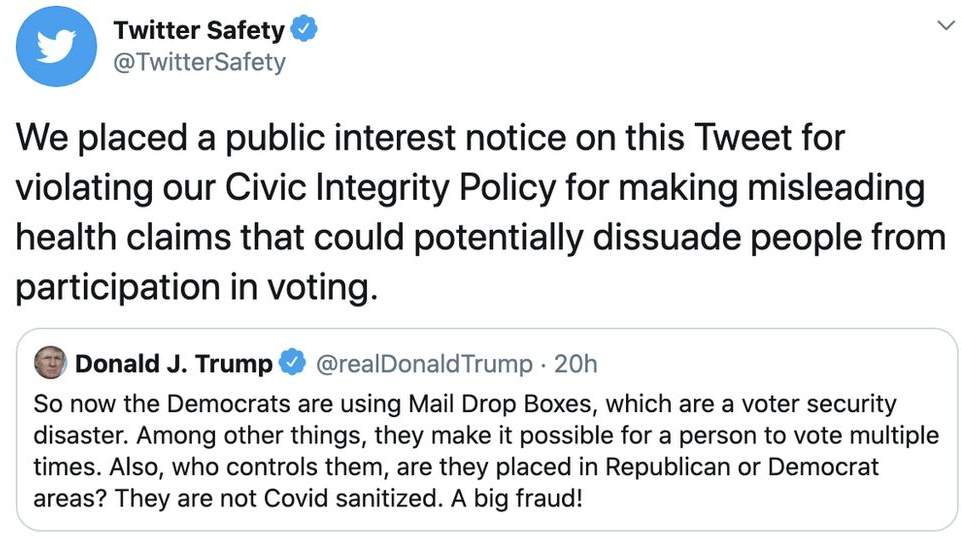
Published by The Lawfare Institute
in Cooperation With
Editor’s Note: To fight election-related misinformation, social media platforms often apply labels that let users know the information from a post is misleading in some way. Samantha Bradshaw of American University and Shelby Grossman of the Stanford Internet Observatory explore whether two key platforms, Facebook and Twitter, were internally consistent in how they applied their labels during the 2020 presidential election. Bradshaw and Grossman find that the platforms were reasonably consistent, but it was still common for identical misleading information to be treated differently.
Daniel Byman
***
As the U.S. midterm elections approach, misleading information could undermine trust in election processes. The 2020 presidential election saw false reports of dead people voting, ballot harvesting schemes, and meddling partisan poll workers. These narratives spread across online and traditional media channels, particularly social media, contributing to a lasting sense that the election was tainted. As of July 2022, 36 percent of U.S. citizens still believed that Joe Biden did not legitimately win the election.
One way social media platforms have tried to fight the spread of false information is by applying labels that provide contextual information. During the presidential election, platforms applied labels that read “missing context,” or “partly false information,” and “this claim about election fraud is disputed.” We know that when social media platforms label misleading content, people are less likely to believe it. But are social media platforms consistent in how they enforce their policies for misleading election information?
Platform consistency matters. It affects whether people trust the information labels applied to posts. Platform consistency also affects whether people perceive the platforms as fair. But figuring out whether platforms are consistent in applying their policies is actually quite difficult.
Gauging Enforcement Consistency
We developed a way to measure platform consistency, leveraging work done by the Election Integrity Partnership in 2020, which both of us were a part of. This coalition of research groups worked to detect and analyze misleading information that could undermine U.S. elections, at times flagging them to social media platforms when they appeared to violate platform policies. From the partnership’s work, we created a dataset of 1,035 social media posts that pushed 78 misleading election-related claims. The partnership identified these posts primarily by analysts running systematic cross-platform queries and then flagged these posts to Facebook and Twitter directly. We use this dataset to quantify whether each platform was consistent in labeling or not labeling posts sharing the same claim.
In our study, we find that Facebook and Twitter were mostly consistent in how they applied their rules. For 69 percent of the misleading claims, Facebook consistently labeled each post that included one of those claims—either always or never adding a label. It inconsistently labeled the remaining 31 percent of misleading claims. The findings for Twitter are nearly identical: 70 percent of the claims were labeled consistently, and 30 percent were labeled inconsistently. When the platforms were not consistent, we were either confused about the patterns we saw, or we were unable to figure out why they treated seemingly identical content differently.
Let’s look at some examples. First, on Election Day an article circulated that implied that delays in the Michigan vote count were evidence of systemic voter fraud. Facebook labeled every instance we saw of this article with, “Some election results may not be available for days or weeks. This means things are happening as expected.”
But in another instance—the day after the election—a graph from the website FiveThirtyEight circulated showing vote tallies over time in Michigan. While the figure illustrated standard and legitimate vote counting, many observers incorrectly interpreted it as evidence of voter fraud. Facebook frequently labeled these images with a “missing context” label, linking to a PolitiFact statement saying, “No, these FiveThirtyEight graphs don’t prove voter fraud.” But when a post shared a photograph of a screenshot showing the graph—rendering it slightly blurry—Facebook did not apply a label.
Left: An unlabeled Facebook post resharing the FiveThirtyEight graph with a misleading framing. Right: A labeled Facebook post spreading the same graph and claim.
We found many examples of Twitter treating identical tweets differently as well. On Nov. 4, 2020, Trump tweeted, “We are up BIG, but they are trying to STEAL the Election. We will never let them do it. Votes cannot be cast after the Polls are closed!” Twitter put the tweet behind a warning label. In response, Trump supporters shared the text of the tweet verbatim. Sometimes Twitter labeled these tweets and sometimes it didn’t, even though the tweets were identical and tweeted within minutes of each other.
Left: An unlabeled tweet resharing Trump’s tweet. Right: A labeled tweet resharing Trump’s tweet.
Explaining Inconsistency
Sometimes the inconsistency was explainable—but not necessarily in a way that aligned with platforms’ stated policies. Looking across the 603 misleading tweets in our dataset, we found that Twitter was 22 percent more likely to label tweets from verified users, compared to unverified users. In one striking example, we observed five tweets that incorrectly claimed that Philadelphia destroyed mail-in ballots to make an audit impossible. Twitter labeled just one tweet, which was from a verified user. Remarkably, this verified user’s tweet was retweeting an unlabeled tweet in our dataset.
Left: An unlabeled tweet from an unverified Twitter user. Right: A labeled tweet from a verified Twitter user, retweeting the unlabeled tweet at left.
On the one hand, it might make sense for Twitter to more frequently enforce its misinformation standards against tweets from verified accounts. Tweets from these accounts may be more likely to go viral, making their misleading messaging more dangerous. On the other hand, a novel aspect of our dataset is that we know Twitter employees saw all of the tweets that the partnership flagged. It is not clear why they would intentionally choose not to label the tweets from unverified users.
What explains some of the inconsistencies we observed on Facebook and Twitter? Our best guess is that the platforms sent the posts we flagged to a content moderator queue, and different moderators sometimes made different decisions about whether a post merited a label. If this is correct, it suggests additional moderator training may be useful.
There may also be some underutilized opportunities for automation. We repeatedly observed inconsistent enforcement in tweets sharing the identical misleading news article. In these cases, platforms could make one decision about whether the article merits a label, and then apply the decision automatically, retrospectively and prospectively. Despite these inconsistencies, it is notable that 70 percent of the time both Facebook and Twitter treated misleading narratives consistently.
An Opportunity for Improvement and Further Study
The analysis we were able to do highlights how collaborations between industry and academia can provide insight into typically opaque processes—in this case, the decision to label misleading social media posts.
As the 2022 midterm elections approach, social media platforms should build their capacity to enforce policies consistently, and also to provide researchers with access to removed and labeled content in advance of the election. This would allow a wider set of researchers to investigate content moderation decisions, improving transparency and strengthening trust in the information ecosystem, a prerequisite for a trusted electoral process.